eDiscovery, financial audits, and regulatory compliance - streamline your processes and boost accuracy with AI-powered financial analysis (Get started for free)
AI in Financial Auditing Transforming Risk Assessment and Fraud Detection by 2025
AI in Financial Auditing Transforming Risk Assessment and Fraud Detection by 2025 - AI-Powered Anomaly Detection Revolutionizes Audit Processes
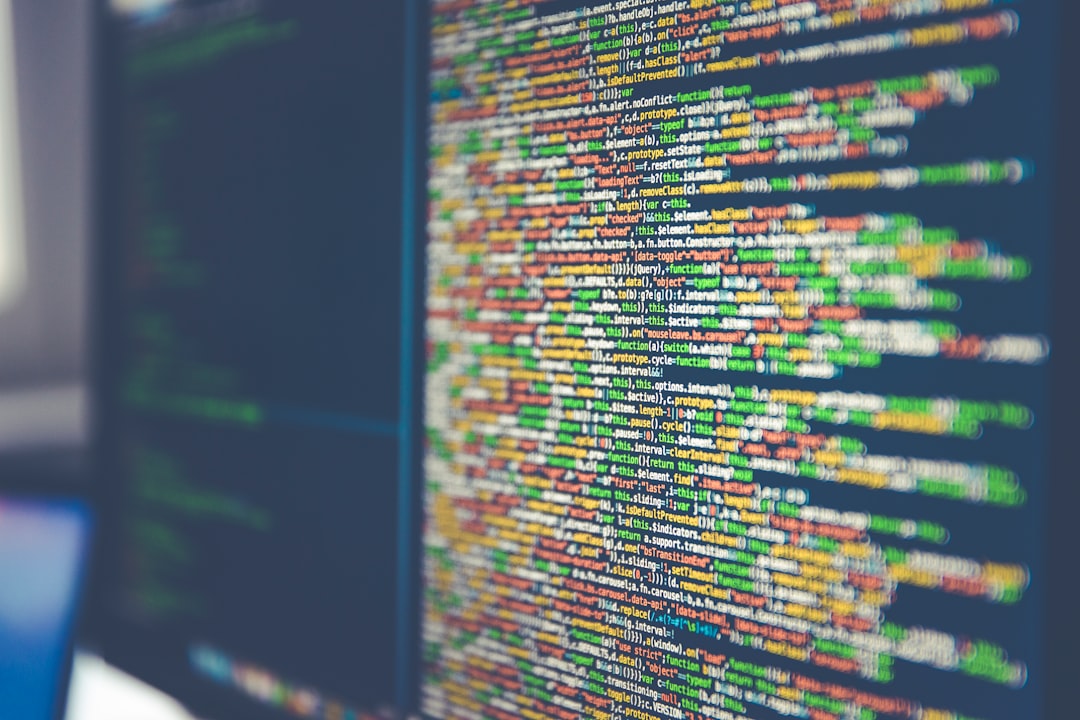
AI is revolutionizing auditing by making it much smarter. Imagine an auditor being able to sift through mountains of data, spotting hidden patterns and anomalies that might indicate fraud or risk. That's what AI is doing. It's like having a super-powered detective that can see things humans miss.
This means auditors can focus on the most important issues, the ones that need their human expertise. This leads to better quality audits and a more efficient process. The ability to automate routine tasks and analyze data in real time changes the whole game. Instead of being reactive, audits can become proactive and insightful.
While AI is a game-changer, it's not a magic bullet. There's a lot of potential for further development, especially in how it's used for internal audits. The key is to use AI's power wisely, combining its strengths with the critical thinking and experience that human auditors bring to the table.
It's fascinating how AI is revolutionizing anomaly detection in audits. The ability to sift through massive datasets in seconds, finding patterns that humans might miss, is truly remarkable. This isn't just about uncovering obvious fraud, but also subtle indicators of irregularities. Imagine an AI system that can learn and adapt, constantly improving its ability to spot potential issues. That's a huge leap forward from traditional auditing methods.
One particularly interesting development is the use of natural language processing (NLP) to analyze unstructured data like emails and contracts. This helps to identify inconsistencies that may suggest fraudulent activity.
Predictive analytics is another area where AI is showing promise. By forecasting potential risk areas, auditors can take proactive steps to mitigate issues before they become major problems.
There's also the potential for cost savings with AI. If algorithms can handle some of the more tedious tasks, auditors can focus on higher-level analysis. Of course, cybersecurity is paramount. AI anomaly detection systems can provide real-time monitoring of financial transactions, quickly identifying suspicious activity.
However, we can't overlook the challenges. AI's "black box" nature is a concern. How can we trust AI's findings if we don't understand how it arrived at them? We need more transparency and explainability to ensure accountability in the auditing process. It's a critical issue to address as AI plays a larger role in financial audits.
AI in Financial Auditing Transforming Risk Assessment and Fraud Detection by 2025 - Machine Learning Enhances Credit Risk Assessment Accuracy
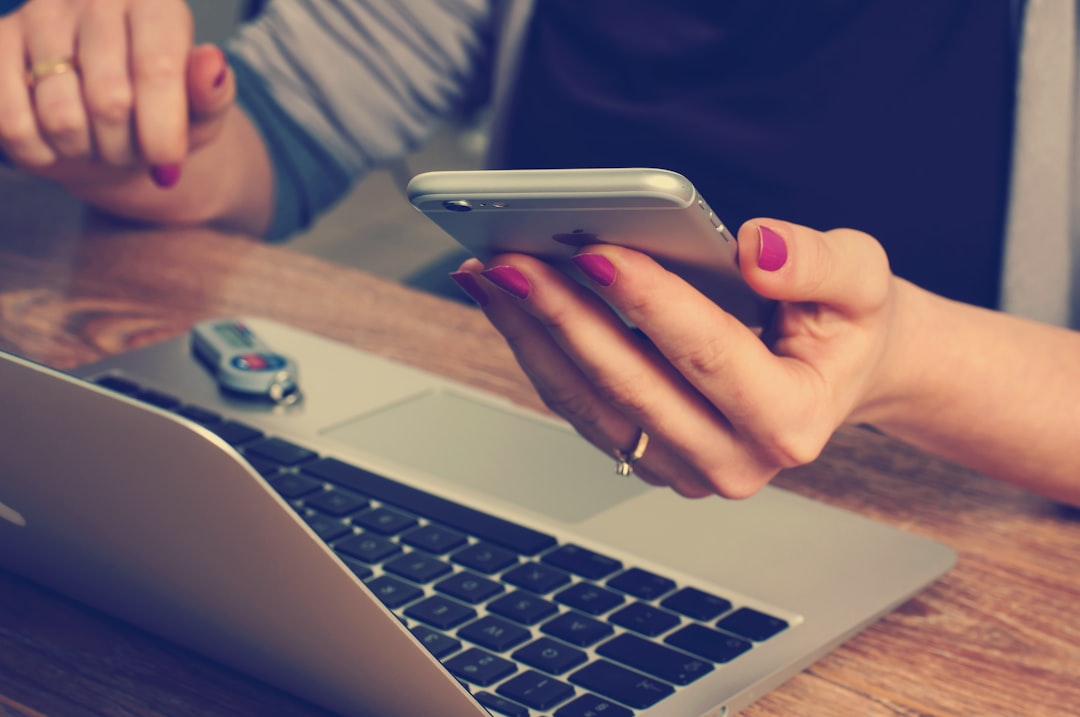
Machine learning is revolutionizing how financial institutions assess credit risk. By analyzing vast amounts of data, ML algorithms can pinpoint patterns and insights that traditional methods often miss. This allows for more accurate assessments of borrowers' creditworthiness, leading to better decision-making, improved risk management, and potentially lower costs.
The shift towards ML-driven credit risk assessment is impacting the entire financial landscape, improving customer experience and driving greater efficiency. These advanced models are particularly powerful because they can integrate various data sources, providing a more comprehensive view of a borrower's financial picture. This is a significant advancement over traditional methods that often relied on limited data points.
While AI promises a brighter future for credit risk assessment, concerns remain about transparency and explainability. We need to understand how these complex models reach their conclusions, especially in a field as crucial as finance. This ensures that we can trust the results and remain accountable for the decisions made. The key is to harness the power of machine learning responsibly, striking a balance between innovation and ethical considerations.
Machine learning is changing the way we assess credit risk. It's like having a magnifying glass that can examine a huge number of factors at once, revealing hidden patterns and trends that would be impossible to see with the human eye alone. This means we can identify risk indicators that might have been missed before, leading to much more accurate and informed credit decisions.
Studies have shown that using machine learning algorithms can increase the accuracy of credit assessments by 20% or even 30%. This means lenders can be more confident in their decisions, reducing the risk of loan defaults and potentially improving their bottom line.
The coolest thing about machine learning is that it can go beyond the traditional data sources like credit scores and income statements. It can also use alternative data sources, like social media activity and transaction history, to gain a much deeper understanding of a borrower's financial behavior. This can reveal valuable insights that might not be captured by the traditional methods.
Another great advantage is that machine learning algorithms can constantly learn and adapt to new information. This is important because economic conditions are always changing, and what might have been a reliable indicator of risk in the past, might not be so reliable in the future. A machine learning system can evolve to stay up-to-date with these changes, maintaining its accuracy over time.
It's pretty interesting to see what kind of patterns emerge when machine learning dives deep into the data. For example, it might find that a person's payment behavior on utility bills is a better predictor of credit risk than their credit score! These insights can be quite surprising, and they definitely challenge traditional ways of thinking about credit risk.
Of course, it's not all sunshine and roses. There are some challenges we need to address. Machine learning models can be incredibly sensitive to the quality of the data they are fed. If the data is flawed or biased, it can lead to inaccurate and unreliable assessments. So, we have to be really careful about the quality and completeness of the data that we're using to train these algorithms.
Another issue is what's called the "black box" problem. We're not always able to understand how machine learning models reach their conclusions. This can make it difficult to explain decisions, which is important for both regulatory compliance and transparency. We need to find ways to make these models more transparent so we can understand the logic behind their decisions.
Bias is another important concern. We have to be mindful of the potential for bias to creep into the training data and influence the algorithms. Biased algorithms can lead to unfair credit decisions that disproportionately impact certain groups. This is a serious issue that we need to address by implementing de-biasing techniques and ensuring that our machine learning models are fair and equitable.
Despite the challenges, machine learning has the potential to transform the way we assess credit risk. It offers a more accurate, efficient, and insightful approach. But it's essential to use it responsibly and to be aware of its limitations. It's an ongoing process of research and development, but the potential benefits are truly exciting.
AI in Financial Auditing Transforming Risk Assessment and Fraud Detection by 2025 - Continuous Auditing Becomes Reality Through AI Integration
AI is taking financial auditing to a new level. It's no longer just about looking at numbers at the end of a period; AI lets auditors analyze vast amounts of data in real time, uncovering potential risks and fraud as it happens. This is a big shift from the old ways, where audits were reactive.
Think of it like having a super-powered magnifying glass that can examine every financial transaction instantly, spotting patterns that might be hiding in plain sight. Auditors can use this information to be more proactive, identifying and addressing issues before they become major problems. This makes audits more efficient, accurate, and insightful.
However, with this powerful new tool comes a big responsibility. We need to make sure the AI algorithms used for auditing are transparent and accountable. We need to understand how they work and be confident in their results. After all, we're dealing with money and trust. As AI becomes more integrated into financial audits, this issue of transparency will only become more important. It's a balancing act between innovation and responsibility.
The idea of continuous auditing powered by AI is really intriguing. Imagine a system that automatically analyzes financial data in real-time, constantly looking for anomalies and potential risks. It's like having a tireless, data-crunching auditor working around the clock, freeing up human auditors to focus on the more complex and strategic aspects of their job.
Studies show that AI can drastically speed up auditing processes, potentially reducing the time required by as much as 75%. This is huge, because it allows financial professionals to spend less time on tedious tasks and more time on analyzing insights, developing strategies, and making informed decisions.
One of the most exciting aspects of this is the ability to analyze unstructured data. We're talking about things like emails, contracts, and even internal communication – data that traditional auditing methods often struggle with. By using AI to analyze these kinds of data sources, auditors gain a much deeper understanding of an organization's operations, which could lead to a more comprehensive and accurate risk assessment.
Of course, it's not all rosy. There are concerns about the potential biases of AI systems, and the "black box" problem still needs to be addressed. We need to find ways to understand how these systems reach their conclusions, so we can ensure that their findings are trustworthy and transparent.
Overall, AI-driven continuous auditing has the potential to revolutionize the way we think about financial audits. It's a powerful tool that can help us identify risk, prevent fraud, and make more informed business decisions. But it's important to use it responsibly and with a critical eye, addressing the challenges and limitations that come with any new technology.
AI in Financial Auditing Transforming Risk Assessment and Fraud Detection by 2025 - Natural Language Processing Transforms Financial Document Analysis
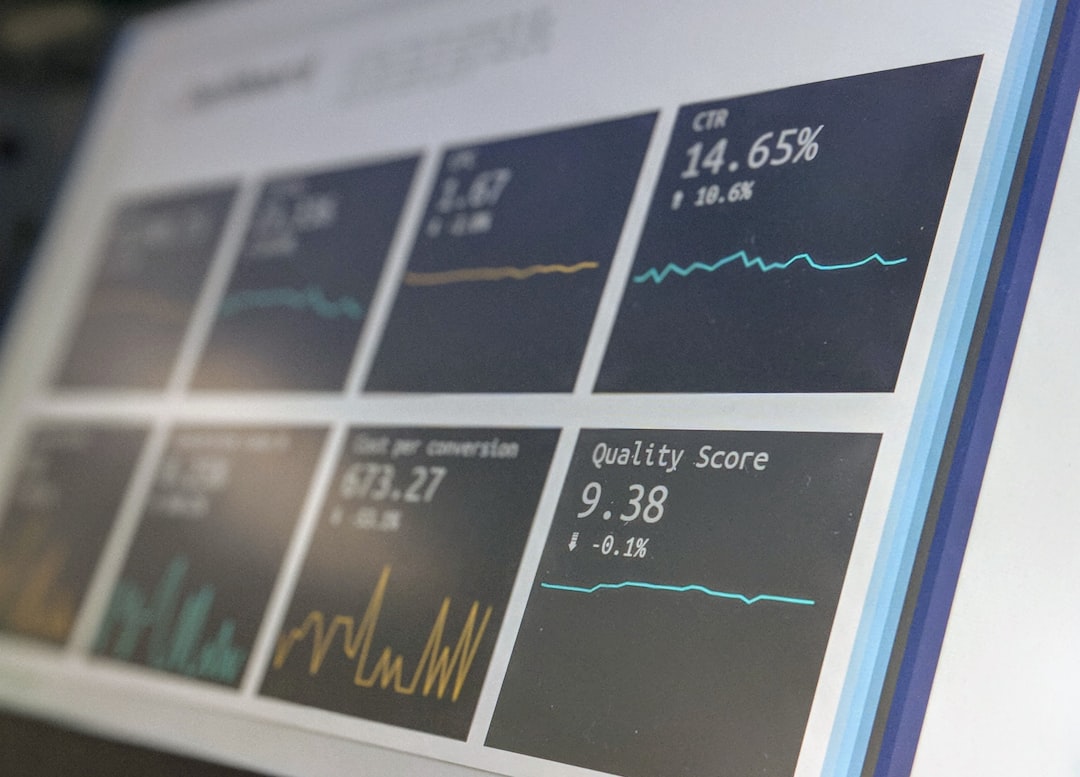
Natural Language Processing (NLP) is transforming the way we analyze financial documents. This technology can make sense of unstructured text, like emails and contracts, which is crucial in financial auditing. By using NLP, auditors can sift through these documents, uncovering hidden inconsistencies and patterns that might signal fraud or risk. This means they can be more proactive in spotting problems and taking action to prevent them.
NLP relies on techniques like text mining and machine learning to find valuable insights in the data. This helps auditors gain a deeper understanding of an organization's financial activities and identify potential issues that might otherwise be missed. However, we need to address some concerns with NLP, like the "black box" problem - understanding how these algorithms make their decisions is vital for trust and accountability. In the future, we need to find a good balance between leveraging NLP's power and ensuring that humans remain in control of the financial analysis process.
Natural Language Processing (NLP) is emerging as a critical tool for financial document analysis. While AI has been revolutionizing audits by finding hidden patterns in data, NLP goes a step further by unlocking the secrets hidden within the text itself. Think of it as a kind of language detective, capable of understanding the nuances of financial documents, not just the numbers they contain.
NLP can be incredibly precise, grasping the contextual meanings within legal jargon and contracts. This kind of analysis is vital when evaluating complex deals or identifying potential red flags that traditional data analysis might miss. And NLP is adept at spotting unusual patterns in text, helping to flag anomalies within financial statements, emails, or contracts. This kind of textual anomaly detection is essential for fraud prevention.
The ability of NLP to extract and categorize information within documents, like dates, monetary amounts, and company names, is a major time-saver. This function streamlines the audit process by eliminating the need for auditors to manually search for these details.
Another interesting aspect is NLP's versatility. Financial documents can be written in a multitude of styles, ranging from formal financial reports to informal email exchanges. NLP's adaptability to different language styles means it can navigate this diverse landscape, extracting meaningful information from every kind of communication.
This technology is also proving beneficial for analyzing historical financial data. By comparing documents over time, NLP can uncover trends and patterns, which can be crucial for understanding the long-term health of a company and predicting potential risks.
Beyond detecting anomalies, NLP can also be used to perform sentiment analysis, gauging the mood and tone of internal communications. While this might seem tangential to financial audits, understanding employee sentiment can reveal potential risks or issues within the company that could ultimately impact its financial performance.
The potential of NLP to automate the tedious task of ensuring regulatory compliance is also significant. This is a critical aspect of financial audits, and NLP's ability to process and check documents against ever-changing regulations could greatly reduce the risk of non-compliance.
Moreover, with the global nature of finance, NLP's multilingual capabilities become essential. It can process and translate documents across multiple languages, ensuring that consistent auditing practices are maintained across different jurisdictions.
Another intriguing application is the integration of NLP with knowledge graphs. These graphs create a web of interconnected data points, allowing auditors to see relationships within the financial documents that might have been missed. This kind of network visualization enhances decision-making by presenting a more complete picture of the data.
While still in its early stages of development, NLP's potential to transform financial document analysis is undeniable. Its ability to decipher complex textual information, identify anomalies, and even understand the sentiment within financial communications presents a powerful tool for auditors. But, as with any AI technology, careful consideration should be given to the potential for bias and the need for transparency in how it arrives at its conclusions. Nonetheless, as NLP matures, we can expect its role in financial audits to grow, making the auditing process more efficient, accurate, and insightful.
AI in Financial Auditing Transforming Risk Assessment and Fraud Detection by 2025 - Predictive Analytics Improves Fraud Prevention Strategies
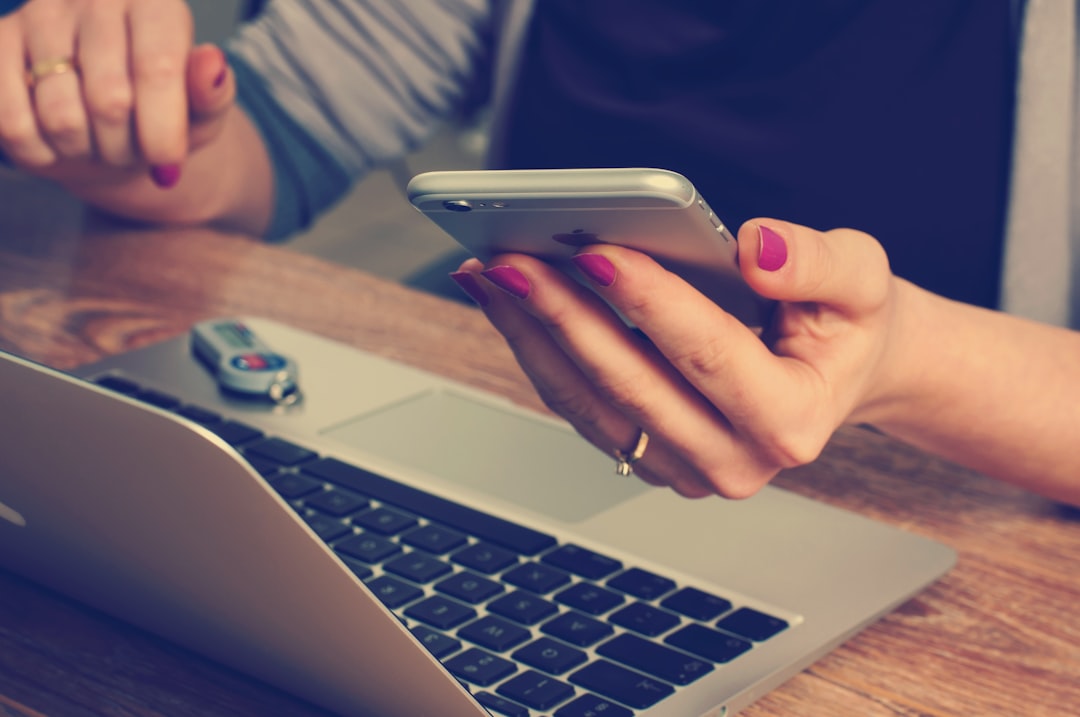
Predictive analytics is changing how we prevent fraud. It's no longer about reacting to problems after they occur. Instead, organizations are now using predictive analytics to spot potential fraud before it happens. These systems work by analyzing huge amounts of data, using statistical models and machine learning to find patterns and trends that could indicate fraud. This helps create early warning systems, giving companies a heads-up about potential issues.
It's a big shift from the old ways of detecting fraud. Previously, we were mainly reactive, looking for problems after they occurred. Now, we're becoming more proactive, using predictive analytics to anticipate potential threats.
This approach is gaining traction in the financial industry, where it's helping institutions detect fraud more effectively. They are using predictive analytics to identify suspicious activity, making decisions based on data rather than gut feelings.
While it's exciting to see how this technology is improving fraud prevention, it's not without its challenges. We need to make sure these systems are transparent and explainable. After all, we need to understand how these models reach their conclusions, especially when dealing with something as critical as fraud. There's always the risk of relying too heavily on technology, so it's crucial to maintain a balance between using these tools and maintaining human oversight.
Predictive analytics is like having a crystal ball for financial fraud. Instead of just reacting to fraud after it happens, predictive analytics can use historical data to spot patterns and predict potential fraud before it even occurs. This is a huge leap forward because traditional methods of detecting fraud are often reactive and slow. Imagine an AI system that can look at thousands of transactions, user behaviors, and other financial data and identify suspicious activity in real-time. That's what predictive analytics can do.
It's not just about spotting obvious fraud, either. Predictive analytics can also pick up subtle patterns that human auditors might miss, like unusual spending habits or unusual combinations of transactions. The algorithms are constantly learning, too. So, as new fraud schemes emerge, the models can adapt and stay ahead of the curve.
Some research suggests that predictive analytics can improve the accuracy of fraud detection by up to 95%. That's a significant jump! Another interesting aspect is that the technology can integrate diverse data sources, creating a richer understanding of potential fraud. This means that the models can identify complex relationships between different pieces of information that humans might not catch.
There are some interesting applications for predictive analytics beyond just fraud detection, too. For instance, financial institutions can use the data to understand customer behavior and improve their customer service strategies.
Of course, we have to be mindful of the potential drawbacks. There's always the concern about the "black box" problem - understanding how these algorithms work is crucial for trust and accountability. We also need to make sure the models are free from bias. After all, the last thing we want is for the technology to perpetuate existing inequalities.
However, despite these challenges, the potential benefits of predictive analytics for fraud prevention are significant. It's a powerful tool that can help us stay ahead of the curve and protect ourselves from the evolving threat of financial crime.
AI in Financial Auditing Transforming Risk Assessment and Fraud Detection by 2025 - AI Augments Human Auditors' Capabilities in Complex Investigations
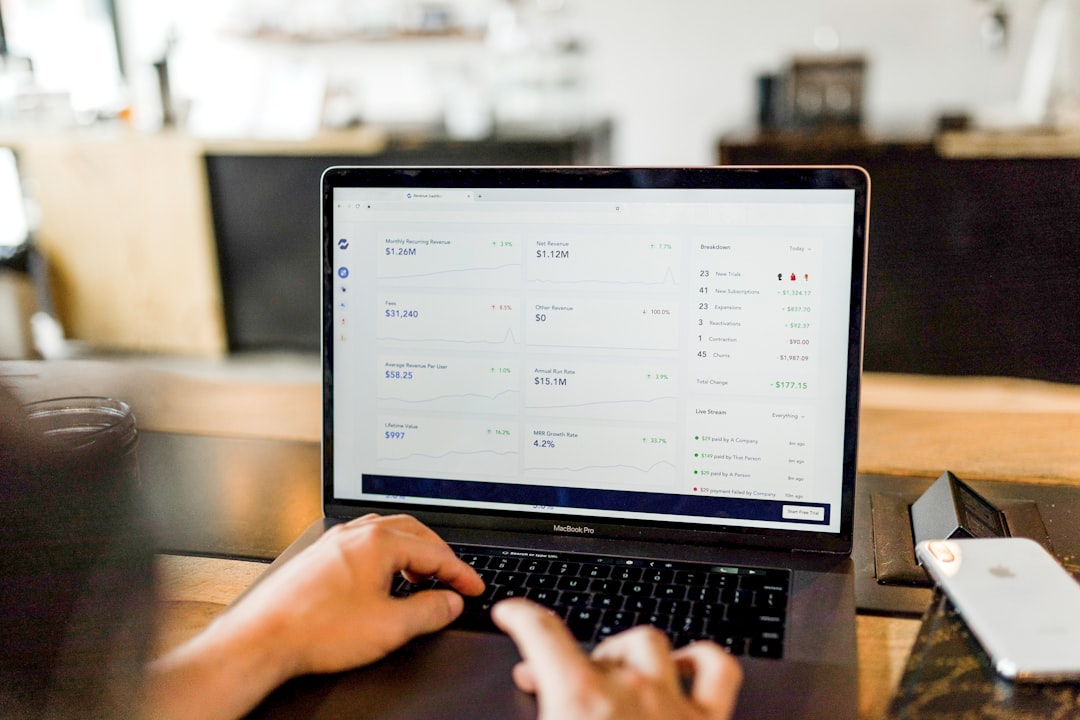
AI is changing the game for financial auditors, especially when tackling complex investigations. Imagine having a super-powered magnifying glass that can analyze mountains of data in a flash, pinpointing hidden patterns and potential problems that would normally slip under the radar. This is what AI is doing for auditors, allowing them to focus their energy on the really important stuff – the tricky stuff that needs their human expertise. So, AI isn't just about automating tasks, it's about giving auditors the power to do their job better and smarter. It's a win-win – audits become more effective and efficient.
But there's a catch. While AI is incredible at crunching numbers and spotting anomalies, we need to be careful. It's vital that we understand how AI reaches its conclusions. We can't just blindly trust the machines, we need to be able to see the logic behind their decisions. We need transparency, and we need to ensure that humans remain in control of the process, even with all this powerful AI technology.
So, the future of auditing looks promising, with AI at the forefront. But it's important to be smart about how we use it. The ideal scenario is one where AI and human auditors work together, each leveraging their unique strengths to achieve the best possible results. It's all about striking the right balance between AI power and human insight.
It's fascinating how AI is transforming the role of human auditors. While the potential of AI in financial audits is undeniable, it's important to view it as a powerful tool, not a replacement for human judgment. AI systems are capable of analyzing complex data in milliseconds, effectively scanning thousands of transactions simultaneously. This speed drastically reduces the time needed to spot anomalies and potential fraud, which previously could take weeks or even months to uncover.
Studies suggest that AI integration in auditing can boost the detection rates of fraudulent activities by as much as 70%. This means auditors can more effectively focus their skills on areas that AI identifies as high-risk, ultimately improving the quality of the audit. It's like having a digital assistant that points you to the most critical areas of investigation, saving time and resources.
But the best part is that AI algorithms can learn and adapt over time. They can analyze past audits and identify patterns of fraud that might have gone undetected before. This ongoing learning process is a huge departure from static auditing approaches and could revolutionize how we adapt to new and emerging financial threats.
One of the most interesting aspects of AI in auditing is its ability to utilize natural language processing (NLP) to analyze correspondence and uncover insights that traditional methods miss. For example, NLP can understand the tone or sentiment within emails, offering valuable clues about unethical behavior or conflicts of interest.
Advanced AI systems can go even further by predicting and simulating different fraud scenarios. By running thousands of simulated transactions through different models, auditors can better understand potential vulnerabilities within organizations’ financial frameworks. It's like playing a game of "what if" with financial data to identify potential risks before they become actual problems.
The potential of AI for improving regulatory compliance is also exciting. AI-powered tools can flag potential compliance issues in real time, alerting auditors before they escalate into larger legal or financial problems. This makes navigating the complex world of regulations more manageable than ever before.
In a globalized financial world, AI can even process and translate financial documents across different languages instantly. This ensures that audits maintain consistent standards regardless of regional complexities in financial reporting. This is a game-changer for auditors working across borders.
Some AI frameworks for auditing use graph analytics to visualize complex interrelations between financial entities and transactions. This reveals hidden connections that conventional auditing methods might miss, making the entire picture clearer. Imagine seeing the entire network of financial transactions and relationships, uncovering subtle patterns that could point to fraudulent activity.
Another benefit is that AI can help auditors make more informed forecasts of future risks. Predictive analytics allows them to look at historical data and predict potential problems before they occur. This proactive approach to risk management can save firms a significant amount of money in potential fraud losses.
While AI systems have enormous potential, we should remember that they still require human oversight to contextualize findings and ensure ethical standards are upheld. The balance of human intuition and AI efficiency is crucial for successful audits. The rise of AI doesn't signal the end of the human auditor, but rather a shift in focus towards more strategic and complex tasks. This highlights the ongoing need for skilled auditors in the AI age, who can expertly navigate the rapidly evolving financial landscape.
eDiscovery, financial audits, and regulatory compliance - streamline your processes and boost accuracy with AI-powered financial analysis (Get started for free)
More Posts from financialauditexpert.com: