AI-Powered Anomaly Detection in Financial Audits A 2025 Analysis of Machine Learning Applications in Risk Assessment
AI-Powered Anomaly Detection in Financial Audits A 2025 Analysis of Machine Learning Applications in Risk Assessment - Neural Network Analysis Catches 3M USD Fraud at Deutsche Bank Trading Desk Through Pattern Recognition
The identification of a $3 million fraud case at a Deutsche Bank trading desk using neural network analysis highlights the capability of these modern techniques to pinpoint complex patterns indicative of misconduct. This specific instance exemplifies the increasing reliance on AI-driven anomaly detection systems now integrated into financial audits, designed explicitly to flag unusual activities within vast and intricate trading datasets that might go unnoticed by conventional methods. The effectiveness seen here supports the broader adoption of machine learning in augmenting detection where historical approaches face limitations.
As we consider the landscape in 2025, the role of machine learning in financial risk assessment continues its evolution. Neural networks and related deep learning frameworks, including those designed for understanding relationship graphs in data, are becoming foundational elements in creating more adaptive and potent fraud detection mechanisms. Progress in refining these algorithms is expected to further sharpen their ability to identify potential threats with greater precision, representing a significant shift in how institutions approach maintaining financial integrity and regulatory adherence, albeit with ongoing efforts needed for seamless implementation and oversight.
A notable demonstration of this capability appeared at Deutsche Bank, where a neural network-based system analyzing trading activity successfully flagged patterns associated with approximately $3 million in fraud. Trained on historical trading records, the algorithm was able to pinpoint subtle, complex arrangements, reportedly involving multiple accounts, that constituted the fraudulent scheme. Such pattern recognition technologies possess the processing speed to sift through extensive transaction volumes rapidly, potentially identifying anomalies far quicker than manual reviews and highlighting non-obvious correlations across disparate data points. While promising, relying on these models brings its own set of considerations, including the continuous need for fresh data to keep the system relevant and the risk of models becoming overly specialized to past examples, potentially missing novel forms of deceit. Nonetheless, instances like this underscore the increasing integration of advanced analytical techniques into financial operations and risk management workflows, sparking discussions about the future of audit practices, the necessary evolution of regulatory frameworks to accommodate automated decision-making, and how successes in one institution might influence broader industry adoption by 2025.
AI-Powered Anomaly Detection in Financial Audits A 2025 Analysis of Machine Learning Applications in Risk Assessment - Machine Learning Models Now Process 100 Million Daily Transactions at Goldman Sachs Audit Department
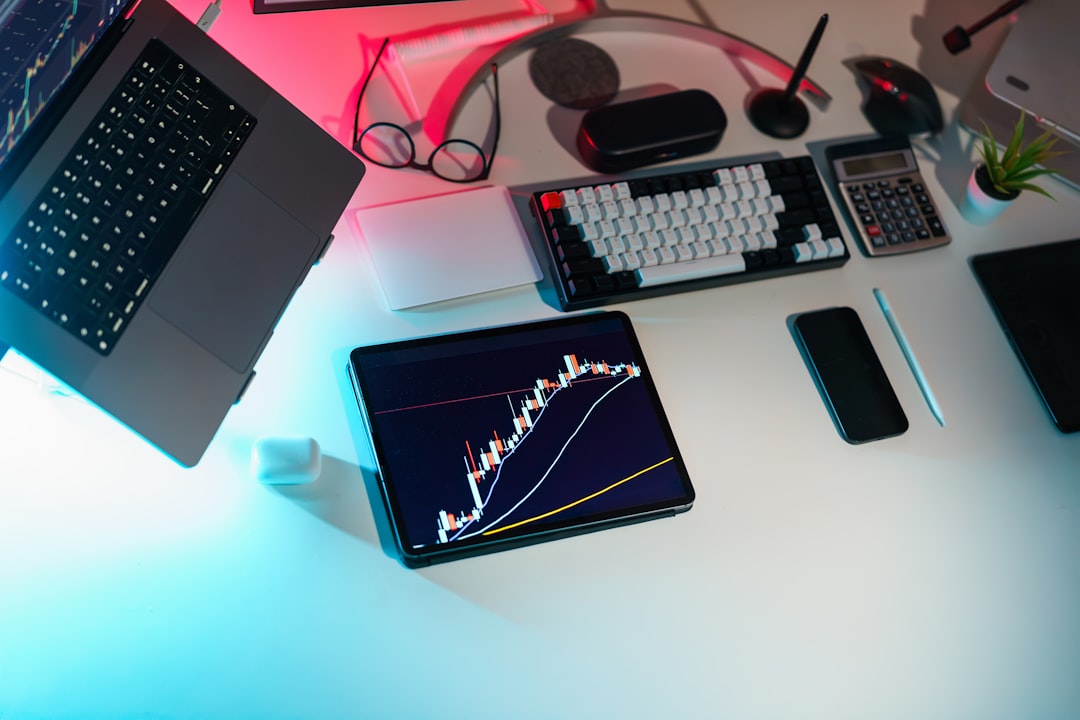
Goldman Sachs' audit department has reportedly integrated machine learning models to process approximately 100 million transactions each day. This implementation aims to leverage AI-powered systems for enhanced anomaly detection within financial audits. By applying these models to vast transaction flows, the intention is to analyze patterns and identify potential irregularities or suspicious activities more effectively and potentially faster than traditional methods might allow across such volume. Utilizing technology in this manner reflects a significant step in applying computational power to internal oversight at scale. However, operating and maintaining these sophisticated models daily against the sheer volume of changing financial data, and ensuring their accuracy and ability to spot novel risks, presents ongoing operational challenges despite the ambition for improved efficiency and risk management. The move aligns with broader trends among large financial institutions exploring AI applications to enhance security and operational control.
Goldman Sachs’ audit function is handling a remarkable volume, apparently processing well over 100 million transactions each day. They're employing machine learning models specifically to sift through this immense dataset in what seems to be near real-time. The scale alone is noteworthy, suggesting that relying solely on traditional manual reviews for timely anomaly detection at this magnitude simply isn't feasible.
From a technical standpoint, the systems reportedly leverage fundamental statistical approaches like clustering to group similar transactions and regression analysis, likely for modeling expected behavior or dependencies. The aim is clearly to pull out patterns or deviations that might signal risk. While these techniques are established, applying them effectively to enhance the accuracy of risk assessments across such a vast and dynamic dataset presents its own set of implementation challenges.
Key to making any such system work is the data it learns from. We're told these models are trained on historical transaction records. This is crucial for establishing what constitutes "normal" activity against which deviations are flagged. The capability to detect even subtle anomalies depends heavily on the quality and comprehensiveness of this historical data, as well as the model's ability to capture complex, non-obvious relationships within it.
A system operating at this scale in finance needs to be adaptable. The mention of continuous learning is vital; the effectiveness of detection models can degrade rapidly as new types of transactions or fraudulent techniques emerge. A model that can refine its understanding and detection capabilities as fresh data flows in is far more likely to remain relevant than one that requires periodic, disruptive retraining. This dynamic learning aspect introduces complexities in model governance and validation, however.
One of the persistent practical headaches with high-volume anomaly detection is the issue of false positives. When you're analyzing 100 million transactions daily, even a tiny fraction of incorrect alerts translates into a significant number of investigations. Fine-tuning models to reduce these spurious flags while still catching genuine issues is an ongoing, often arduous engineering task. It highlights the need for a pragmatic balance between sensitivity and specificity.
The development and deployment of these tools also require a close partnership between the technical teams building the models and the finance professionals who understand the intricate nuances of financial operations and regulations. Ensuring the algorithms are not just mathematically sound but also practically meaningful and aligned with audit objectives is critical for their real-world utility. This bridge between data science theory and financial practice is often where significant hurdles lie.
Expanding beyond structured transaction tables, the ability to analyze unstructured data sources, such as associated emails or internal notes, can provide invaluable context. Incorporating insights gleaned from text and other less organized data types adds another layer of sophistication to the anomaly detection process, potentially revealing contextual clues that pure quantitative analysis might miss. This step significantly increases the complexity of data pipelines and model architectures.
However, the increased reliance on automated decision-making processes raises important questions about transparency and accountability. When a machine learning model identifies a potential issue, or perhaps more critically, *fails* to identify one, understanding *why* that decision was made can be difficult. The inherent 'black box' nature of some complex models poses challenges for auditors and regulators who need clear justifications, particularly when significant decisions are involved without direct human intervention.
This integration inherently shifts the role of the human auditor. Their focus increasingly moves from painstaking manual reviews to interpreting the outputs and alerts generated by the models. This requires auditors to develop new skills related to understanding model capabilities, limitations, and how to investigate the anomalies highlighted by the AI. It's a transition that requires significant training and adaptation within the profession.
Ultimately, as these sophisticated machine learning systems become integral to financial audit processes, there's a clear and growing need for regulatory bodies to develop specific guidelines and frameworks. These need to address how such models should be validated, monitored, and governed, ensuring they are used responsibly, transparently, and ethically, balancing the undoubted efficiency gains with the need for rigorous compliance and oversight standards in the financial sector.
AI-Powered Anomaly Detection in Financial Audits A 2025 Analysis of Machine Learning Applications in Risk Assessment - The SEC Adopts Mandatory AI Risk Assessment Protocol Following March 2025 Financial Market Events
Following unsettling activity in financial markets during March of 2025, the regulatory landscape has shifted significantly, with the SEC mandating new protocols for AI risk assessment among firms like broker-dealers and investment advisers. This move appears directly linked to the March events, pushing for greater scrutiny on how predictive data analytics and artificial intelligence are used, particularly concerning potential conflicts of interest and the broader need for adherence to rules. Regulators have been voicing increasing concerns about the risks inherent in widespread AI adoption within finance, even as the technology is explored for its potential efficiencies in areas such as identifying anomalies in large datasets. Recent discussions hosted by the SEC itself have highlighted this tension, acknowledging AI's capabilities while emphasizing the need for robust governance and risk management to protect market integrity. The practical rollout and effectiveness of these mandatory assessments across diverse financial operations remains a key challenge, requiring careful adaptation from firms navigating this evolving oversight.
Stepping back from the immediate operational successes seen with machine learning in detecting specific anomalies, the regulatory landscape has taken a significant turn following the financial market events in March 2025. The SEC has now implemented a mandatory protocol for assessing risks specifically associated with the use of AI systems within financial institutions. This isn't merely a suggestion; it's the first time we've seen regulators worldwide mandate a *specific framework* aimed at standardizing how these systems are evaluated for safety and reliability across the board.
A key technical demand of this new protocol is the requirement for regular, deep audits of AI systems. Previously, scrutiny might have focused on the outputs, but this protocol shifts the focus inwards, demanding insight into the *algorithmic transparency* itself and a close examination of potential biases embedded within the data the models are trained on. From an engineering perspective, this is a complex undertaking, requiring detailed documentation. Institutions are now compelled to log everything from the sources and preparation of their training data to the specific methodologies used to build the models, along with their performance metrics. This level of mandatory audit trail for machine learning models is quite unprecedented and introduces a significant layer of accountability, making it theoretically possible for regulators to understand the computational basis for decisions or to trace potential issues arising from flawed data or model design.
Navigating this regulatory mandate requires more than just the data science team. The protocol necessitates a genuinely multidisciplinary effort, pulling in expertise from legal and compliance alongside the engineers and analysts. This integration can be challenging for firms not accustomed to bridging these typically distinct domains, yet it underscores the multifaceted nature of managing AI risk beyond purely technical performance. The SEC has also signalled the depth of its commitment by establishing a dedicated task force. This group isn't just processing paperwork; it's focused on identifying and understanding emerging technical threats, such as *adversarial attacks* – the subtle manipulation of AI inputs designed to cause the system to fail or generate false results, a real concern for market integrity.
Furthermore, the protocol emphasizes that AI systems aren't 'set it and forget it' tools. It mandates *continuous monitoring* and iterative refinement, acknowledging that these technologies are dynamic and require ongoing adjustment to remain effective, particularly in spotting novel forms of risk or market manipulation that weren't present in historical training data. This implies a continuous operational burden for technical teams. It also pushes institutions toward proactive vulnerability testing through *scenario analysis*. Firms are now required to simulate various "what-if" situations to predict potential failures or biases within their AI systems before they manifest in the real world, aiming for a more anticipatory approach to risk management rather than reacting after an incident occurs.
Perhaps a more unexpected, though arguably critical, element is the protocol's focus on the human interface. It highlights that auditors working with these sophisticated AI outputs need more than just traditional financial knowledge; they require a blend of technical understanding and critical thinking skills to interpret the AI's findings and exercise sound judgment, a considerable shift in the expected skill set for the auditing profession. A particularly stringent requirement is the mandate for *explainability*. All AI systems used in financial audits must be capable of explaining their workings or the rationale behind their outputs to external stakeholders like clients and regulators. Achieving true explainability for complex deep learning models remains an active area of research and poses a significant challenge for deployment, but it's deemed essential for maintaining trust in automated processes. This move by the SEC isn't happening in isolation either; it's reportedly prompting other regulatory bodies globally to consider similar frameworks, potentially laying the groundwork for a set of international standards governing AI risk assessment in financial services, which could influence future AI development trends.
AI-Powered Anomaly Detection in Financial Audits A 2025 Analysis of Machine Learning Applications in Risk Assessment - Natural Language Processing Identifies Accounting Discrepancies in Cross Border Payments Through Transaction Metadata
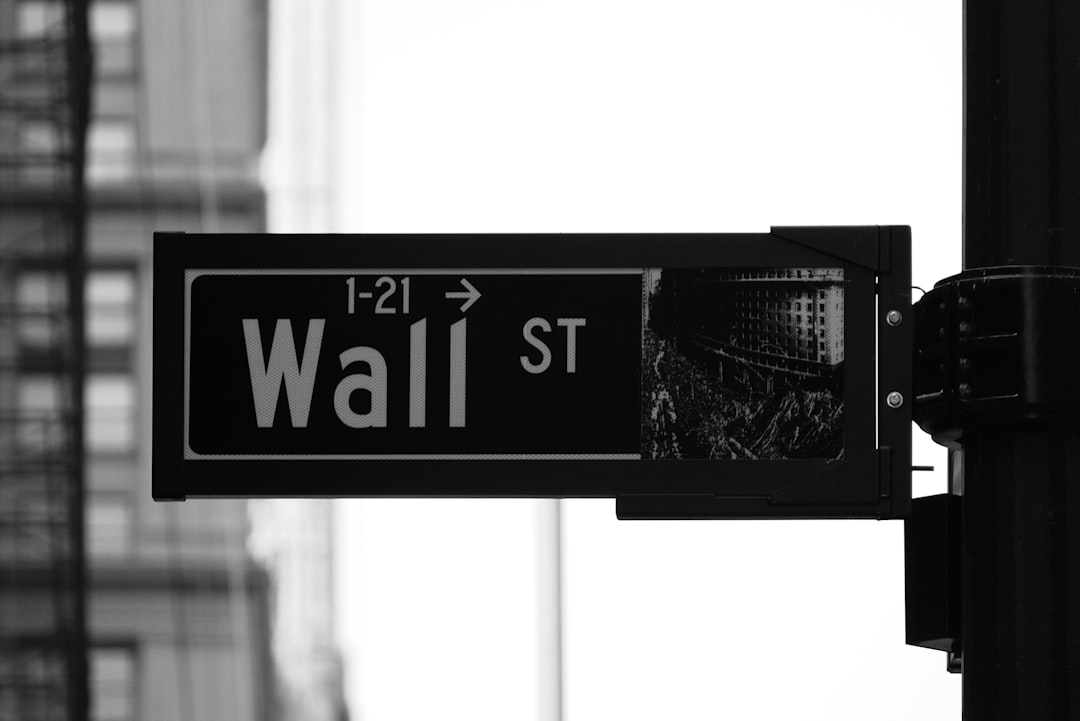
Focusing on specific applications within financial audits for 2025, Natural Language Processing is showing utility in pinpointing potential accounting discrepancies, particularly within the complexities of cross-border payments. It operates by examining the textual context and metadata surrounding these transactions – elements like payment descriptions, attached notes, or related communications. The intent is to use NLP to read and interpret this unstructured data, searching for language patterns, inconsistencies, or references that might not align with the numerical figures or could signal unusual activity. This represents an effort to glean insights from qualitative information at a scale impractical for manual review. While promising for augmenting anomaly and potential fraud detection by adding a layer of contextual understanding, the accuracy depends heavily on the quality and nature of the text data available, and the interpretation models themselves require ongoing refinement to handle diverse language and nuances across jurisdictions and payment methods.
Systems are exploring the application of Natural Language Processing to dissect the contextual information often embedded within transactional metadata – think notes fields or attached messages. The idea is to move beyond just the numbers and dates, seeking qualitative cues that might hint at unusual activity, particularly relevant when examining complex global flows that lack standardized descriptions.
Navigating cross-border payment data brings inherent complexities, from differing conventions in descriptive text across jurisdictions to variations driven by currency handling and local rules. NLP techniques are being applied to help normalize and interpret this disparate textual data, aiming to improve consistency in how potential discrepancies are flagged despite the underlying heterogeneity across different payment systems and formats.
Much of the descriptive data accompanying payments arrives as free text – the kind found in unstructured notes or communications. Training models to reliably extract meaningful, structured signals relevant to anomaly detection from this 'messy' natural language remains a persistent technical challenge, though essential for uncovering hidden clues that aren't captured in standard fields.
A core potential benefit often cited is the ability of NLP to process the sheer volume of textual transaction data at speeds impractical for manual review. This near real-time analytical capability could theoretically allow institutions to identify potential issues within cross-border payments significantly faster, potentially mitigating exposure or rectifying errors more rapidly than traditional batch processes allow.
Analyzing text generated across diverse linguistic and cultural contexts poses a subtle but significant challenge for NLP models. The same phrase or intent might be expressed differently, or local jargon could obscure meaning. Developing robust models requires acknowledging and accounting for these cultural and linguistic nuances to avoid misinterpretations that lead to detection errors or overlooked risks.
Combining the features extracted by NLP from text with structured transactional data within machine learning frameworks offers a more holistic view. This integrated approach is being tested to see if it can improve the identification of complex anomalies by leveraging the interplay between quantitative metrics and qualitative textual descriptions in ways that neither method could achieve in isolation.
A critical concern with any NLP application is its reliance on training data. If the historical transaction text reflects historical biases – perhaps in how certain types of transactions or participants from specific regions were described – the resulting NLP models could inadvertently perpetuate these biases, leading to unfair or inaccurate anomaly flagging. This requires careful auditing of training sets and ongoing monitoring.
Ensuring these NLP-driven detection systems remain effective over time in a dynamic environment necessitates robust feedback loops. Models need to learn from the inevitably high rate of initial false positives and refine their understanding of 'normal' versus 'abnormal' text patterns as new data, new business practices, and new methods of obfuscation emerge.
From a compliance perspective, the ability to automatically analyze transaction descriptions for terms or phrases that might indicate potential regulatory red flags – even subtle ones relating to sanctioned entities or restricted goods – is seen as promising. This could help organizations proactively identify transactions requiring closer scrutiny to meet complex international regulations.
Ultimately, while NLP provides powerful analytical tools for sifting through text, the interpretation of ambiguous textual data or the nuanced reasons behind a flagged item will likely still require human expertise. The role appears to be shifting towards auditors interpreting complex model outputs, validating findings, and applying professional judgment where automated systems reach their limits, particularly with subtle textual cues or highly novel scenarios.
More Posts from financialauditexpert.com: