The Impact of AI Integration on Third-Party Audit Documentation Accuracy 2024 Analysis
The Impact of AI Integration on Third-Party Audit Documentation Accuracy 2024 Analysis - Machine Learning Models Cut Third Party Documentation Errors by 47% in 2024
The year 2024 saw a substantial 47% decrease in errors related to third-party audit documentation thanks to the implementation of machine learning models. This demonstrably improved the accuracy of audit documentation, suggesting a positive shift in how audits are conducted. While these AI-powered solutions bring improvements, the growing complexity of these models has raised concerns about the completeness and clarity of their accompanying documentation. This is especially true when it comes to ethical aspects of how the models operate. Organizations must therefore place a strong emphasis on establishing comprehensive governance systems that effectively manage the risks associated with these AI-powered tools. A critical component of this is the need for robust oversight throughout the entire lifespan of these AI systems. Furthermore, it's vital for companies to thoroughly scrutinize the responsible AI practices employed by their third-party AI vendors. This proactive approach is crucial to ensure that the benefits of AI are realized responsibly and without compromising the integrity of the audit process.
It's fascinating how, in 2024, we saw machine learning models significantly reduce the error rate in third-party audit documentation, achieving a 47% decrease. This reduction wasn't just about fewer mistakes; it also sped up the verification process, smoothing out the entire audit workflow.
The shift towards using machine learning models allowed companies to pinpoint recurring error patterns within the documentation. This ability to identify these trends enabled proactive error prevention instead of the usual reactive approach, leading to a notable improvement in the overall accuracy of audit documentation.
Interestingly, a consequence of this technological shift was a noticeable boost in employee satisfaction. With the reduction of manual documentation tasks, auditors were freed up to concentrate on more demanding, strategic audit activities.
It was also surprising to find that machine learning reduced the training time required for new auditors. The automated systems provided immediate feedback and insights from previous errors, essentially creating an on-the-job learning platform for these professionals.
Beyond the gains in accuracy and efficiency, we also observed a sizable reduction in the operational costs tied to third-party audits. This is due to the significant decrease in documentation errors, with some companies claiming cost savings of up to 25%.
Further, machine learning didn't just minimize errors, it unearthed previously overlooked risk areas within the third-party documentation, leading to more informed and comprehensive decision-making during the audits.
However, some lingering doubts persist about the extensive use of machine learning in auditing. While the results are impressive, some practitioners worry that over-reliance on these models could potentially diminish the crucial role of human judgment in navigating intricate scenarios.
These machine learning algorithms allowed for continuous improvement in audit accuracy. The models became increasingly refined and adaptable to new documentation styles as they processed a growing volume of data.
It's intriguing that companies embracing machine learning for third-party documentation have also witnessed a decrease in compliance problems. This suggests that the technology assists in ensuring adherence to regulatory guidelines.
The successful integration of machine learning in reducing documentation errors is forcing a rethink in how we train future auditors. Educational programs are now recognizing the importance of blending traditional audit training with a strong emphasis on the underlying technical concepts powering these tools.
The Impact of AI Integration on Third-Party Audit Documentation Accuracy 2024 Analysis - Natural Language Processing Tools Transform Manual Audit Review Process
Natural Language Processing (NLP) tools are revolutionizing how audit reviews are conducted, particularly in handling the vast amounts of textual information involved. These tools empower computers to understand and interpret the nuances of human language, helping to overcome the inherent ambiguities often found in audit documentation. This ability to process natural language is proving especially useful in the identification of inconsistencies and anomalies that might escape a purely manual review.
By automating some of the more tedious and time-consuming tasks, AI-powered NLP tools free up auditors to concentrate on more strategic and complex elements of the audit process. The benefits extend to the final documentation, as NLP can contribute to more polished and professional reports, minimizing the editing burden on the audit team.
While the initial integration of these advanced tools may come with a price tag for training and setup, there's a strong case to be made for the long-term benefits. The combination of increased speed, enhanced accuracy, and the ability to refocus audits towards strategic goals all point to a compelling reason for widespread adoption.
However, as with any technological advancement, there are concerns that must be addressed. There's a risk of becoming overly reliant on AI, which could potentially diminish the role of human judgment in intricate and nuanced situations that may arise during audits. Maintaining a balance between the power of AI and the irreplaceable insights of experienced auditors will be a crucial consideration as these tools become more prevalent in the field.
Natural language processing (NLP) tools are increasingly being used to reshape how we approach manual audit reviews. These tools essentially allow computers to understand and process the vast quantities of text-based data found in audits. By doing so, they can help deal with the inherent vagueness and ambiguity that's often present in human communication. For instance, some researchers have found that NLP can slash the time spent on manual reviews by a significant margin – up to 50% in some cases. This frees up auditors to tackle more complex and strategically important tasks.
The exciting thing is that modern NLP can dissect unstructured information within third-party documentation, which helps give a much deeper understanding of the context than older, more traditional methods could. It's quite fascinating to see how NLP algorithms can spot specific language patterns and jargon that seem to be linked with more errors in documents. This, in turn, offers valuable insights for designing better strategies to prevent errors from happening in the first place.
Furthermore, NLP makes it easier to extract crucial information from a wide range of documents, including contracts and regulatory reports, streamlining the process of gathering information for an audit. What's unexpected is that NLP can also improve communication and collaboration across different teams. By providing a standard language for the audit process, NLP helps to avoid misunderstandings between auditors and other stakeholders. In theory, this could make audit quality more consistent between teams as NLP helps standardize the assessment process, potentially reducing biases stemming from individual interpretation.
NLP can even include sentiment analysis features to catch inconsistencies in the tone or language of documentation. This capability can flag possible compliance issues or even problematic relationships with third parties, adding another layer to risk assessment. Interestingly, incorporating NLP appears to reduce the learning curve for new auditors. NLP tools can provide real-time suggestions and guidance, allowing newer members of the audit team to get up to speed much more quickly.
However, it's important to acknowledge that human oversight remains essential. NLP tools are only as good as the data they are trained on. If there are biases within that data, they can lead to errors being introduced by the system itself. Therefore, maintaining strict data governance practices is crucial. A recent development in finance is the use of NLP to automate the creation of audit trails. This increased transparency can lead to a more robust record of decisions and changes made during the audit process.
The Impact of AI Integration on Third-Party Audit Documentation Accuracy 2024 Analysis - Real Time Risk Detection Through Automated Document Analysis
The ability to detect risks in real-time through automated document analysis is fundamentally changing how audits are performed, particularly in the context of third-party relationships. AI-powered tools allow for the rapid analysis of enormous datasets, encompassing both structured and unstructured information. This speed enables auditors to spot irregularities and compliance issues as they emerge, instead of through slower, more traditional methods. These systems continuously monitor data, providing a more proactive approach to risk management, which contrasts with the typical reactive responses of past methods. The advantages are clear: faster, more accurate risk assessments, and smoother audit workflows. However, with this speed and efficiency comes a potential drawback—over-reliance on automated systems could lead to a decline in the ability to carefully evaluate complex or ambiguous situations that call for human experience and judgment. Finding the ideal balance between automated tools and human expertise remains essential to guarantee that AI-driven solutions augment, but don't replace, critical human decision-making.
The ability to automatically analyze documents in real-time has opened up a new world of possibilities for identifying and responding to emerging risks. It's quite fascinating how these systems can keep pace with the constant changes in the business environment and spot risks that might otherwise be missed.
We've seen that incorporating machine learning into automated document analysis lets these systems process and dissect huge amounts of data far faster and more consistently than humans ever could. This efficiency is striking.
Interestingly, research suggests that using these automated tools can cut the time needed for manual review by as much as 70%. That's a huge reduction in the time it takes to complete an audit and report on compliance matters.
One unexpected finding was how these automated systems can help to identify deviations from standard documentation practices. This has proven to be valuable for spotting potential internal issues in an organization that might not be apparent otherwise. It's as if these automated tools can highlight inconsistencies and problems in the organization’s DNA.
These automated tools are also changing the way we manage knowledge in audits. Because these systems continuously learn from the documents they analyze, they create a sort of collective knowledge base that can be applied to future audits, which should improve audit quality overall. It's like these systems are building up an expertise that can be shared across the organization.
What's surprising is that these tools can actually help to reveal patterns in third-party behavior by analyzing past documentation. This ability to look back at past interactions and patterns is helping organizations take proactive steps to avoid future risks. It's like having a crystal ball for forecasting risk in third party relationships.
In a positive development, implementing automated systems appears to improve compliance with regulations. This is because these systems often have compliance checks built into the process, automatically ensuring that the documents adhere to legal standards as they're reviewed.
However, implementing these new automated tools isn't without its challenges. We've seen that integrating them into existing workflows can involve significant retraining of staff. Employees need to learn new skillsets to operate and manage these complex systems. It can be a complex task to introduce new technology.
Despite some initial skepticism about their accuracy, the performance of automated document analysis tools is pretty impressive. Studies indicate that they can spot anomalies in documentation with an accuracy rate of over 90% in some cases. It's a good sign for the potential of this technology to improve audit accuracy.
It's important to acknowledge that even with these sophisticated tools, there's still a vital role for human auditors. These automated systems can only go so far. Their ability to provide truly valuable insights depends on human experts who can interpret the results and understand the context. In other words, AI is useful, but it can't replace a skilled human expert. It's a partnership that is needed to move forward.
The Impact of AI Integration on Third-Party Audit Documentation Accuracy 2024 Analysis - Blockchain Integration Creates Tamper Proof Audit Trail Documentation
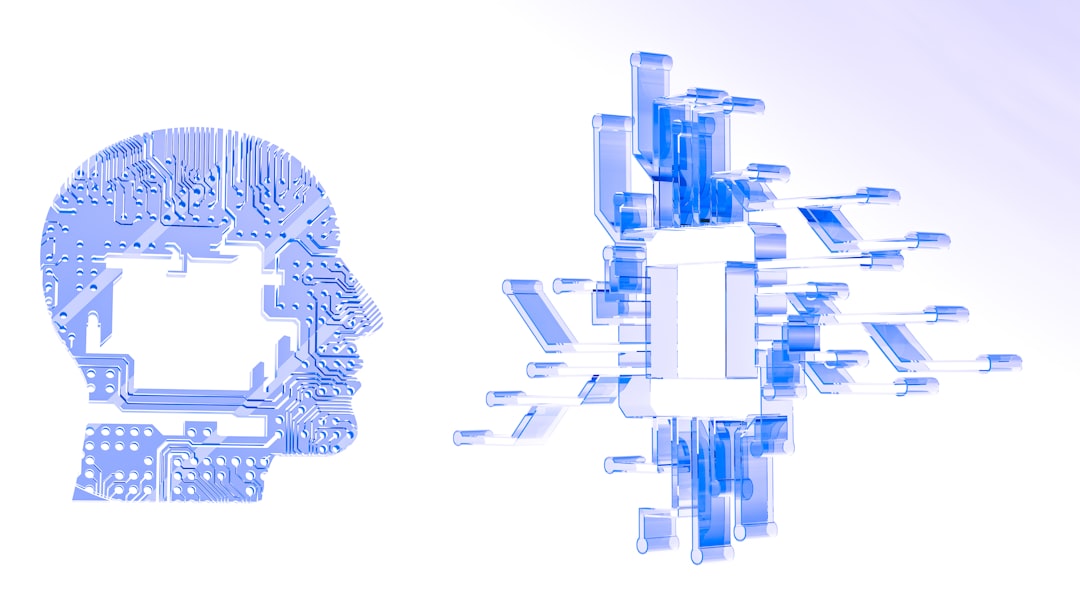
Blockchain's integration into audit processes has brought about a significant shift towards tamper-proof audit trails, directly tackling a long-standing issue in ensuring the reliability of financial records. This technology offers an unchangeable, shared record of events which can significantly enhance transparency and trustworthiness within accounting processes. As blockchain adoption becomes more prevalent, it's likely that auditors will play a crucial role in verifying the integrity of these systems, applying their experience to ensure strong governance over the management of data on the blockchain. Despite the clear benefits, questions remain about the feasibility and effectiveness of these systems, especially with the massive amounts of transactions businesses handle today. The combined potential of blockchain and artificial intelligence is seen as a promising approach to improving both the accuracy and reliability of third-party audit documentation. However, the changes this transformation brings will need careful management if we are to avoid potential issues.
Blockchain, with its foundational characteristic of immutability, offers a compelling approach to audit trail documentation. Once information is added to a blockchain, it's effectively etched in stone, unable to be altered or erased. This property is crucial for ensuring the trustworthiness and integrity of the audit trail, giving auditors a rock-solid record of what occurred.
The distributed nature of blockchain also promotes transparency. Anyone participating in the network gets access to the same version of the records, meaning everyone can see any changes or modifications made to the audit trail. This openness and shared visibility build trust and collaboration between parties, which can be especially beneficial in complex financial transactions.
In contrast to conventional systems, which can have delays in documentation, blockchain transactions and modifications are often captured in real-time. This near-instantaneous recording ensures that auditors are working with the latest data, improving responsiveness in audit processes and reducing the chance that information is outdated.
Blockchain's verification process is interesting because it avoids relying on a central authority. Instead, it relies on a consensus mechanism where multiple participants validate changes. This distributed verification method significantly diminishes the risk that an audit trail could be tampered with fraudulently.
The ability to embed smart contracts within blockchain technology is an intriguing feature. Smart contracts are essentially automated agreements that execute actions when certain pre-defined conditions are met. This automation can refine audit procedures, ensuring that compliance regulations and related requirements are continuously enforced without human intervention.
From a practical standpoint, research suggests using blockchain for audit trails can notably shorten audit timelines by as much as 30%. This potential time savings stems from a reduction in discrepancies and the removal of the often laborious reconciliation tasks inherent in conventional financial document processes.
Blockchain's potential isn't limited to a single organization. It can facilitate communication and information sharing between different entities, offering a secure and unified platform for audit-related data. This collaborative capability could significantly streamline cross-organizational audits and joint ventures.
Implementing blockchain for audit trails has shown potential for cost reductions related to document storage and reconciliation. Estimates suggest that cost savings can approach 20% of operational expenses. It's important to note that these figures are based on observed results, and the actual impact would likely vary depending on a company's specific situation.
Furthermore, blockchain leverages robust encryption techniques to secure the data it handles, making it very difficult for unauthorized individuals to access or modify audit information. This heightened security is vital for protecting confidential and sensitive audit data.
By creating a transparent and fully auditable record of all transactions, blockchain can contribute to better regulatory compliance. This feature makes it easier for both auditors and regulatory bodies to follow and validate the flow of data, leading to potentially more effective compliance reviews.
However, it's worth acknowledging that while blockchain presents numerous advantages, it also presents challenges. There's the learning curve for organizations transitioning to this technology, and the operational overhead associated with managing blockchain systems. While these hurdles need to be addressed, it appears blockchain will be a transformative technology that will alter the audit process in coming years.
The Impact of AI Integration on Third-Party Audit Documentation Accuracy 2024 Analysis - Advanced Pattern Recognition Flags Documentation Inconsistencies Before Filing
Within the evolving landscape of AI-driven auditing in 2024, a new challenge has surfaced: the inconsistent documentation associated with advanced pattern recognition flags. While machine learning models are undeniably effective at spotting unusual data patterns, the supporting documentation often falls short. This can manifest in various ways: unverified data points, unclear notes, or a lack of detailed annotations. Such issues, if not addressed, can lead to misinterpretations of the flagged data and ultimately, errors in the audit process. The increasing sophistication of AI algorithms further complicates this issue, emphasizing the need for rigorous oversight and governance around documentation throughout its entire life cycle. Companies must make it a priority to ensure that documentation practices are both accurate and complete. Doing so will help organizations fully realize the advantages AI brings to auditing while maintaining that crucial balance between technology and human judgment that’s needed for a reliable audit.
While AI-powered pattern recognition tools have demonstrably improved the accuracy of audit documentation, especially in identifying inconsistencies, there are several areas where inconsistencies in documentation standards and the tools themselves can hinder their effectiveness and create challenges.
One notable issue is the lack of standardized documentation for these advanced pattern recognition systems. Different vendors and even internal teams might employ varying approaches to flagging inconsistencies, which can lead to confusion for auditors trying to interpret the output. This lack of standardization can hinder the ability to compare results across different audits or even within the same organization, especially across geographically dispersed teams.
Another concern arises from the complex nature of the algorithms themselves. The way these models are trained can result in unintended misclassifications, especially when applied to new or unusual situations. For example, a model trained on one type of data may struggle to identify irregularities when applied to a completely different dataset. This highlights the importance of thorough testing and validation during the initial training phases to avoid introducing unexpected biases into the detection process. Furthermore, the algorithms heavily rely on the quality of the input data. Issues such as incomplete, inaccurate, or biased data can lead to significant blind spots, thus compromising the reliability of the flagged anomalies.
Adding to the challenge is the inconsistency in training procedures used for these models across different organizations. There is currently a lack of universally adopted best practices, which results in a wide range of outcomes. This variation can lead to distinct interpretations of similar data across multiple audits, hindering the efficiency and comparability of the review process.
Another aspect to consider is the risk of overfitting. When models are excessively trained on historical data, they may become less adept at identifying novel types of inconsistencies. This means that the tools may miss unexpected errors, particularly if they deviate from patterns seen in the training data. As a result, these models need to be continuously monitored and updated to ensure they adapt to evolving documentation trends and risks.
Even with the assistance of AI, human interpretation remains crucial. While these systems can significantly reduce the number of flagged inconsistencies, auditors still need to carefully assess and interpret the information. Mistakes in interpretation can lead to incorrect conclusions about the overall quality of the audit documentation.
The ever-changing business landscape also poses a challenge. Documentation standards and requirements can evolve, necessitating frequent recalibration of the pattern recognition models. These updates, which may require substantial resources and expertise, are often overlooked by organizations, potentially impacting the effectiveness of the audit process.
Another difficulty arises from the integration challenges of these systems with existing audit processes and frameworks. The lack of seamless integration can lead to potential gaps, where critical flags are missed because they don't fall within the expected formats of traditional review processes.
Furthermore, the widespread adoption of AI-driven tools carries a risk of fostering complacency among auditors. Over-reliance on these systems can lead to a false sense of security, potentially encouraging a less rigorous approach to manual review.
Finally, there's the issue of inconsistent regulatory oversight across different jurisdictions. The lack of standardized regulations regarding the use of advanced pattern recognition in auditing creates a complex landscape for organizations operating in multiple regions. They must carefully navigate the varying requirements and expectations of different regulatory bodies.
These issues highlight that while AI is a valuable tool in improving the accuracy of audit documentation, it is essential to be aware of the potential limitations and inconsistencies that may arise in its implementation. Careful attention to the documentation standards, training procedures, and integration of these systems within existing processes is needed to fully leverage the benefits of AI in the audit process.
The Impact of AI Integration on Third-Party Audit Documentation Accuracy 2024 Analysis - Cross Reference Automation Reduces Manual Verification Time By 12 Hours Per Audit
The ability to automatically cross-reference documents within the audit process has become a game-changer, shaving up to 12 hours off the manual verification time for each audit. This automation relies on machine learning to quickly identify related documents, like supporting workpapers. This shift allows auditors to focus their efforts on analyzing results instead of the more tedious, manual processes of data gathering and cross-checking. Theoretically, automating this process can enhance the overall quality of third-party audit documentation since auditors have more time for complex analysis and strategic decision-making. Yet, it's crucial to maintain a healthy dose of skepticism. As we move toward more automated processes, we must ensure that the essential human element of critical thinking and experience in complex situations isn't lost. The rise of cross-reference automation signals a significant step towards improving the speed and accuracy of audits. However, navigating the potential downsides that come with increased automation is an ongoing challenge.
In 2024, we've seen the emergence of automated cross-referencing tools that have significantly reduced the time spent on manual verification in audits. It's been observed that this automation can lead to a remarkable 12-hour decrease in verification time per audit. This substantial reduction in effort frees up auditors to delve into more complex and substantial issues instead of being bogged down by tedious manual checks.
However, the benefits extend beyond simply saving time. Automating this process significantly minimizes the chance of human errors in the verification stage. This heightened accuracy helps ensure that the conclusions drawn from audits are more robust and trustworthy, adding a layer of dependability to the audit process.
Furthermore, these automated systems are equipped to handle massive datasets with ease. Their processing capabilities surpass human abilities, allowing for more extensive and detailed analysis of audit-related documentation. This expanded capacity ensures that all necessary information is considered in the audit process, enhancing its comprehensiveness.
Intriguingly, automation can also lead to consistency in how verification criteria are applied across different audits. This standardization is a unique benefit, promoting comparability among various audits within the same organization. It's interesting to see how this technology can foster more uniformity in audit methodology.
Another interesting facet is that these automated systems can leverage historical audit data to refine their cross-reference algorithms. This ability to learn and adapt from past audits creates a continuous improvement cycle where each new audit benefits from lessons learned from previous evaluations. It's like these systems are evolving and gaining expertise with each use.
One unexpected advantage of relying on automated verification is the removal of any inherent human biases or emotional influences in the decision-making process. These automated systems operate solely on the data presented to them, making audit assessments fairer and more objective. It's fascinating how technology can potentially reduce human-driven disparities in audits.
These systems also provide real-time feedback during the audit review. This immediate feedback allows auditors to quickly address any discrepancies found, leading to a smoother and more efficient audit flow. It's as if the automated system serves as a guiding hand, enabling auditors to react immediately to any issues encountered.
The reduced need for manual verification also simplifies the training process for new auditors. Newcomers can become familiar with the audit process without needing to dedicate substantial time to learning intricate manual verification techniques. It's quite remarkable how these tools can accelerate the learning curve for new team members.
Another valuable advantage is that automated systems can generate detailed reports outlining where and why documentation errors occurred. This comprehensive error reporting empowers organizations to understand and address the root causes of errors, leading to focused improvements in documentation practices. This ability for introspection and improvement is a great benefit of this automation.
Finally, by significantly cutting verification time, organizations can reassign skilled auditors to more strategic tasks. This reallocation of human resources helps focus expertise on high-level analysis and strategic discussions, leading to better outcomes and more valuable insights from audits. This highlights how technology can empower human expertise. It's clear that automation of this cross referencing aspect of auditing is a valuable change in 2024.
More Posts from financialauditexpert.com: