New Study Reveals Hidden Biases in Financial AI Implications for Audit Processes
New Study Reveals Hidden Biases in Financial AI Implications for Audit Processes - Hidden Biases in Financial AI Systems Uncovered
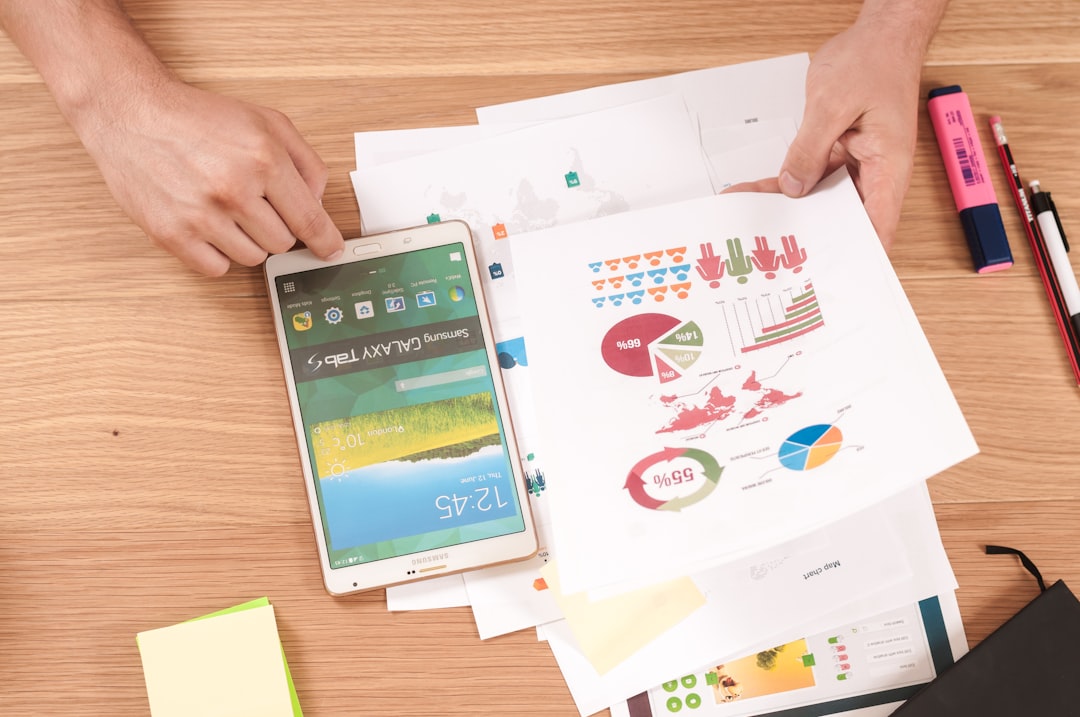
The intricate workings of financial AI systems are increasingly revealing previously concealed biases, posing significant questions for audit processes and the broader financial landscape. It's becoming apparent that the biases inherent in these systems aren't solely a product of the algorithms. Rather, they often originate from human biases embedded within the training data itself. This realization compels financial entities to conduct thorough audits of their data sources and establish methods to detect and curtail bias.
Experts are now raising concerns about the need for greater transparency and accountability in AI systems used in finance. This push underscores the growing challenge for the industry to ensure that AI's implementation doesn't inadvertently worsen existing inequities within the financial system. The task ahead is crucial: proactively tackling these hidden biases to foster a more just and equitable financial ecosystem for everyone.
It appears that the way financial AI systems are built can unintentionally carry forward biases present in the data they're trained on. This is especially concerning when it comes to historical lending practices, which might have unfairly disadvantaged certain groups. Studies have shown how credit scoring algorithms can inadvertently favor one demographic over another, often based on factors related to socioeconomic status rather than true creditworthiness. Even minor changes in the data used to train these systems can lead to strikingly different outcomes in loan approvals and risk assessments, emphasizing the delicate balance required for fair AI implementation.
Moreover, the complexity of these AI systems can make traditional auditing methods less effective in identifying and addressing bias. Auditors are challenged by the opacity of some algorithms, where it can be difficult to trace the reasoning behind decisions. The growing reliance on automated systems has also raised worries about a reduction in human oversight, which could further solidify biases if AI results aren't carefully scrutinized.
Research has revealed a concerning trend where AI systems perform well for some groups but not as well for others, resulting in unequal consequences. This highlights the need to assess the impact of these systems across various demographics. Furthermore, there seems to be a disproportionate focus on commercial AI applications in research funding, potentially leaving less attention to address the ethical considerations needed for fairness in financial AI. The tendency to believe that because AI is computer-driven it must be unbiased – what some call "algorithmic blindness" – can be a significant impediment to effective bias monitoring.
It's become apparent that bias detection and mitigation isn't a one-off task. Because society and financial behaviors change over time, there is a constant need for vigilance in ensuring AI systems maintain fairness. This requires ongoing monitoring to adapt algorithms to evolving societal trends, preventing the gradual development of unfairness within the systems.
New Study Reveals Hidden Biases in Financial AI Implications for Audit Processes - Impact on Audit Quality and Financial Reporting Accuracy
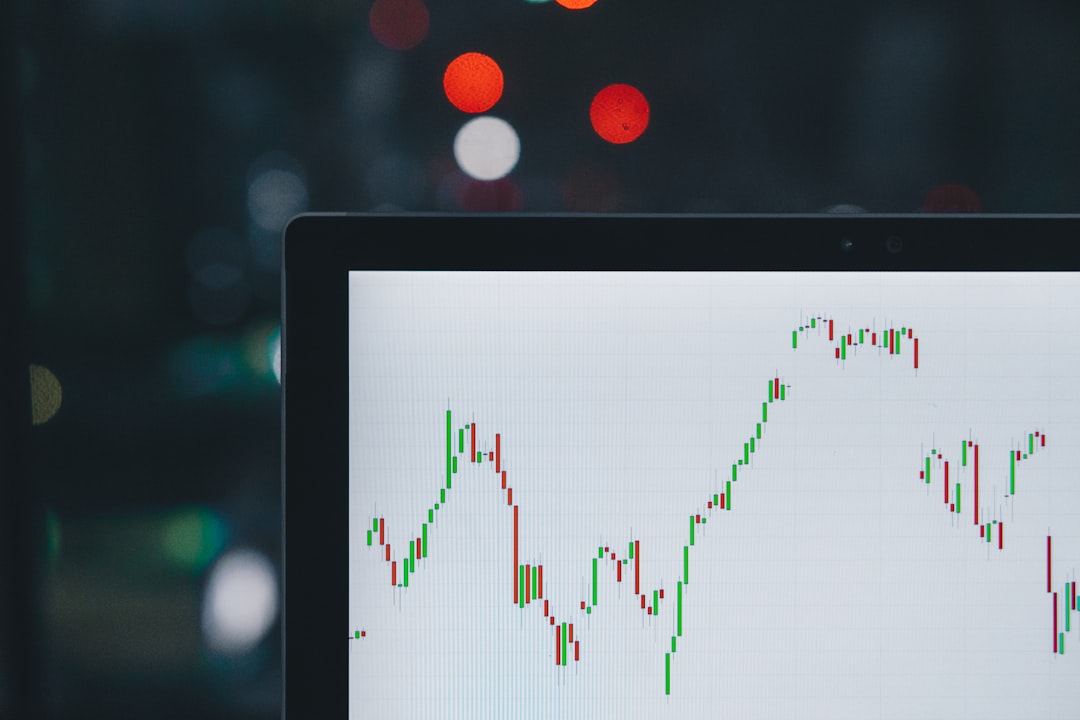
The increasing use of AI within financial reporting and auditing is fundamentally altering the landscape of audit quality and the accuracy of financial reporting. Many businesses now expect auditors to evaluate the role of AI in financial reporting, specifically looking for assurance about the controls surrounding AI implementations. This highlights the growing importance of AI within financial processes and the need for greater transparency and oversight. However, the recent uncovering of biases within financial AI systems presents a significant risk to the integrity of financial reporting. This necessitates auditors to shift towards more proactive and ongoing analysis to identify and address these biases. Achieving a balance between the efficiency that AI offers and the crucial requirement for accurate and unbiased financial reporting presents a considerable challenge. Auditors must be vigilant in evaluating both the AI systems themselves and the audit procedures utilized, ensuring that the pursuit of efficiency doesn't compromise the accuracy and reliability of financial reporting. The future of audit quality relies on navigating this complex interplay between automation and human oversight to ensure the integrity of financial data.
The increasing use of AI in financial reporting processes is leading to a fascinating, and sometimes concerning, set of developments in the world of auditing. It seems that many companies are relying on AI not just for analysis but for making crucial financial decisions. This begs the question of who's responsible when AI-driven decisions, potentially skewed by embedded bias, impact audit processes.
It's become evident that the biases found in these AI systems aren't solely a product of the algorithms themselves. Instead, the training data, often reflecting human biases, can be the root cause of the problem. This suggests that audits might need to shift focus to scrutinize the data sources that AI learns from.
Understanding how these complex systems arrive at conclusions is becoming a hurdle for auditors. It's getting harder to trace the decision-making path in many cases, which makes it challenging to ensure the transparency necessary for accurate financial reporting. Even small adjustments to the training data, which might seem trivial, can significantly influence AI outcomes. It's a reminder of how fragile the link between data inputs and outputs can be and underscores the importance of rigorous auditing.
Further complicating things is evidence that AI doesn't always perform equally across demographic groups, which should raise serious concerns for auditors looking to ensure fairness and accuracy. It's becoming clearer that audits need to move beyond just accuracy and look at whether AI systems treat different groups fairly.
Unfortunately, a lot of stakeholders seem to implicitly trust that AI is inherently free from bias, a tendency some researchers have dubbed 'algorithmic blindness'. This can hinder the implementation of necessary bias detection safeguards during the audit process.
Clearly, bias detection and mitigation aren't a one-time fix. Given that societal trends and financial practices are constantly changing, keeping audits current requires ongoing monitoring and adaptation of AI systems. If companies fail to adapt their AI systems to evolving societal trends, their audits might quickly become out of date.
It's also interesting that a lot of the research funding in this area tends to be focused on commercial AI applications. This may lead to a neglect of the ethical considerations necessary to ensure fairness and good audit quality across different sectors of the financial world.
Furthermore, there's a concern that as automation in the audit process increases, human oversight can decrease. This could lead to biases becoming entrenched without the checks and balances provided by human judgment. It seems a balance between AI capabilities and human expertise is crucial.
Finally, there's a growing push for greater transparency in AI systems from regulators. This could fundamentally alter how audits are conducted and how financial reports are created, prompting a reevaluation of traditional practices. It'll be important to see how this evolving regulatory landscape affects the field of financial auditing in the coming years.
New Study Reveals Hidden Biases in Financial AI Implications for Audit Processes - AI Transformation of Risk Assessment in Auditing
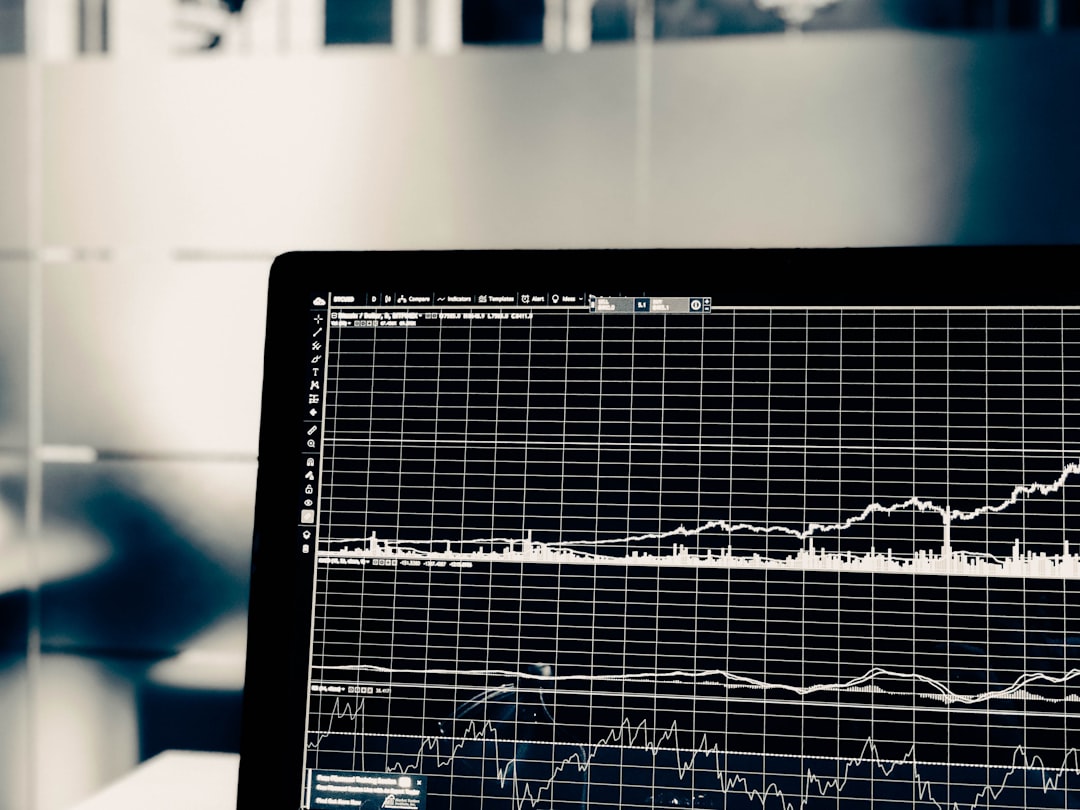
Artificial intelligence is fundamentally altering how risk is assessed within auditing practices. More and more businesses are using AI in financial reporting, leading to a shift towards greater automation in data analysis and audit procedures. This includes the capacity to continuously monitor financial activity and identify unusual patterns that might otherwise go unnoticed. Auditors can leverage these improvements to transition from simply responding to risks to proactively anticipating and mitigating them.
However, the rise of AI in financial auditing also raises concerns about potential biases embedded within the AI systems. Because AI algorithms learn from data, any biases present in that data can inadvertently be amplified and reflected in the AI's outputs. This necessitates a heightened awareness of the training data that underpins AI models and a more rigorous approach to ensuring the impartiality of risk assessments.
The future of auditing, therefore, hinges on effectively balancing the efficiencies offered by AI with the imperative of fairness and transparency in financial reporting. This involves a careful evaluation of AI's role in risk assessment and the establishment of robust controls to identify and address any biases that might arise. Ultimately, human oversight remains essential to safeguard the integrity of audit processes and ensure that AI-driven advancements do not inadvertently perpetuate or worsen existing inequalities within the financial system. As this landscape continues to change, auditors will need to adapt and implement new approaches to ensure that AI enhances, not undermines, the core principles of a sound audit.
A large portion of companies, around three-quarters, are using AI in their financial reporting, with expectations of nearly universal adoption within the next three years. This surge in AI use is poised to revolutionize the audit process. AI can streamline things like automation, data analysis, and continuous auditing. It's seen as a way to make auditors more effective, but if not done right, it could easily be just more of the same old problems, just faster. It's also interesting how fast generative AI has taken off, with adoption across consumers and businesses exploding in less than a year.
AI is being used in many ways during audits, like testing journal entries, searching for strange patterns in big datasets, and even during the initial stages of planning and risk assessment. However, the change that AI is bringing to how anomalies are addressed is a potential shift.
It's not just the financial world that's dealing with AI's impact on fairness. In New York City, businesses using AI for hiring are required to assess the potential for bias in their tools. The whole situation highlights how quickly AI is changing things, and this poses both new opportunities and risks to internal audit departments. New methods for tackling strange results and errors are clearly needed.
AI has the capability to boost transparency and how well an audit performs. This makes it even more important to carefully evaluate how AI is being utilized in auditing practices. It's not simply a trend, a report from KPMG made it clear that AI in financial reporting and auditing is a fundamental shift in how many industries operate.
As audit practices integrate AI, it's become clear that figuring out if hidden biases are present in financial AI systems is a major concern. While this idea has been around, the research and application of it are still a work in progress and pose potential dangers that must be studied before the industry rushes into an uncritical adoption. Auditors now need to be trained in a new era, and it remains to be seen how successfully they can adapt.
New Study Reveals Hidden Biases in Financial AI Implications for Audit Processes - Diversity Concerns in AI Auditing Teams
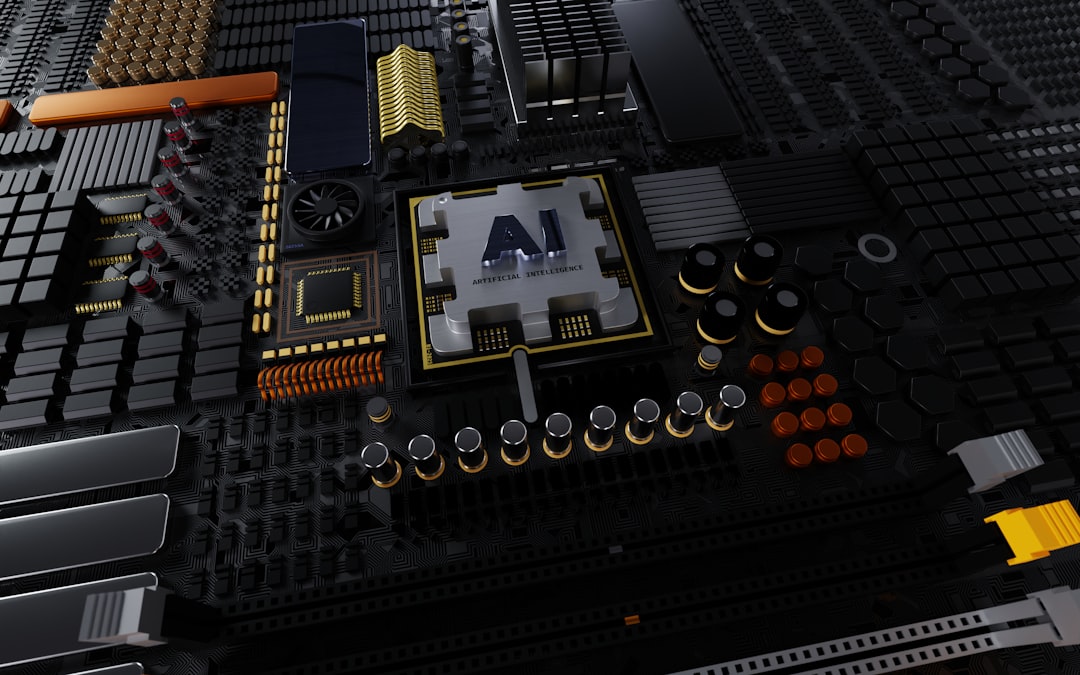
The increasing integration of AI into financial audits highlights a crucial concern: the need for diverse auditing teams. When audit teams lack diversity, there's a higher risk of overlooking biases embedded within the AI systems they are evaluating. This is because a limited range of perspectives can hinder the identification of potential blind spots within the algorithms. Consequently, AI-powered decisions, especially in sensitive areas like credit scoring or risk assessment, may disproportionately impact marginalized communities. Ethical questions about fairness and equity are central to this issue. To ensure that financial AI delivers reliable and unbiased results, audit teams must be equipped with a wide array of experiences and viewpoints. Building inclusive teams, however, is a challenge that needs dedicated attention. If organizations can succeed in this endeavor, audits will be better positioned to critically assess the underlying assumptions and potential biases within AI models, contributing to more trustworthy financial reports.
Research indicates that a lack of diversity within AI auditing teams can hinder their ability to effectively identify and address biases embedded in AI systems. This is because shared backgrounds and perspectives can inadvertently normalize certain biases, leading to their perpetuation within financial models and decision-making processes. For instance, if a team primarily consists of individuals from similar backgrounds, they might be less likely to recognize biases that disproportionately affect other demographic groups.
A recent survey highlighted this concern, with over half of AI audit teams expressing that their effectiveness is limited by a lack of diversity. This emphasizes the need for a broader range of experiences and backgrounds within audit teams to better identify and mitigate biases. Moreover, AI applications in finance can inadvertently worsen existing equity issues, especially for vulnerable populations who may be disproportionately impacted by biased algorithmic decisions. Thus, diverse teams are crucial for designing audit protocols that prioritize equity and fairness in the implementation of AI.
Furthermore, the absence of diversity can foster an "echo chamber" effect, where prevalent perspectives and opinions go unexamined. This can reinforce biases within AI algorithms and the data they're trained on, ultimately jeopardizing the validity of audit conclusions. Conversely, organizations with greater diversity in their audit teams have reported higher innovation rates and improved problem-solving skills, indicating that a homogeneous team composition can hinder progress in developing effective bias detection methods.
Interestingly, some research suggests that organizations with more diverse technology teams experience better decision-making processes, superior AI assessment outcomes, and a lower occurrence of bias-related audit failures. This observation further strengthens the argument for prioritizing diversity in AI auditing teams. In the broader context, the global workforce is increasingly demanding diverse representation. Financial institutions that fail to align their audit teams with this trend may face reputational damage and a decline in public trust.
Evidence demonstrates that biased AI outcomes are often detected and rectified faster when audit teams include a blend of genders, ethnicities, and professional backgrounds, suggesting that a diverse team fosters enhanced problem-solving capabilities. This push for diversity in auditing teams isn't just an ethical imperative, it's also a strategic business decision. Research indicates that companies with inclusive teams tend to outperform their competitors, suggesting a clear economic advantage to incorporating a wide range of perspectives.
Despite this evidence, surprisingly, many financial institutions still emphasize technical skills over diversity in recruiting AI auditing roles. However, multiple surveys suggest that a wider range of skills, including social awareness and empathy, can significantly improve audit quality and enhance bias mitigation efforts. It seems that moving forward, there's a critical need to reconsider the current hiring priorities for AI auditing roles.
New Study Reveals Hidden Biases in Financial AI Implications for Audit Processes - Challenges in Resolving AI-Generated Audit Adjustments
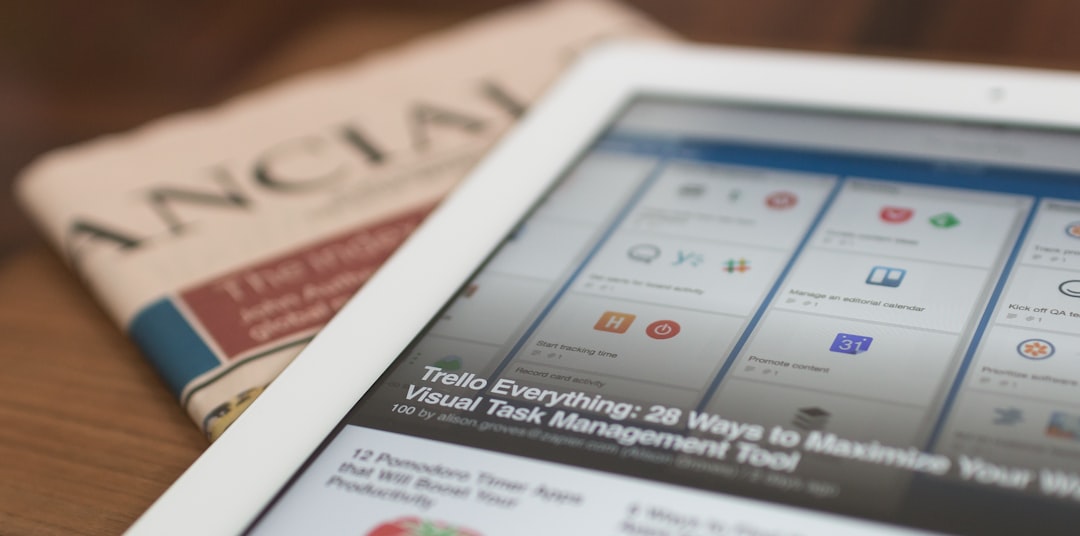
The integration of AI into financial processes, particularly the generation of audit adjustments, introduces a set of challenges for the auditing profession. As AI tools become more commonplace for tasks like valuing assets, the complexity of these automated decisions increases the need for auditors to understand how they're reached. This scrutiny is crucial because AI systems often reflect the biases present within the data they are trained on, making it important to ensure fairness and equity in the process. A key hurdle is the lack of transparency in many AI models, making it difficult to trace the reasoning behind audit discrepancies and subsequently correct them. It becomes critical for auditors to find a balance where AI innovation is embraced, but only with a robust layer of human oversight to maintain both the accuracy and reliability of financial reporting. It's a balancing act between the new possibilities of AI and the necessity of well-founded and transparent audit practices.
The interplay between AI-generated audit adjustments and human biases is becoming increasingly complex. We're seeing that AI's decisions can be heavily influenced by the data it's trained on, and that data can reflect societal biases, particularly around socioeconomic factors. This means that the historical context of how data is collected can potentially distort future audit outcomes in ways we might not expect.
It's fascinating—and concerning—that even a slight change, like a 1% alteration in the training data, can lead to dramatically different audit adjustments from AI systems. This highlights just how sensitive these systems are to subtle variations in the data they're fed, making a reliance on fully automated audit processes rather risky.
A major challenge for auditors is the fact that some AI algorithms are quite opaque, making it difficult to understand the rationale behind their decisions. This 'black box' problem complicates standard auditing practices that aim to ensure both the accuracy and transparency of financial reporting.
There's a growing expectation that AI will play a central role in financial audits within the next few years, with more than 70% of surveyed companies anticipating it. However, many auditors currently don't feel sufficiently prepared to navigate the challenges of AI's complexity and the inherent biases these systems can perpetuate.
Despite awareness about bias within AI, about a third of audit teams haven't implemented any specific policies for identifying and managing potential bias within the algorithms they are examining. It's as if many still believe AI is immune to the same issues that affect humans.
The tendency to trust AI outputs simply because it's technology—what researchers call "algorithmic blindness"—is a significant hurdle for effective auditing. It can prevent us from questioning AI results critically, potentially leading to the reinforcement of existing biases.
Surprisingly, formal training on AI ethics and bias detection is often lacking in the audit training programs within many financial institutions. This means that many auditors are stepping into the world of AI without the proper tools to understand and confront the biases they might encounter.
While research consistently points to the benefits of diverse audit teams in identifying AI biases, a lot of companies still prioritize technical skills over a diverse range of backgrounds during hiring. This approach inadvertently hinders the potential for effective bias mitigation.
Studies show that diverse audit teams can pinpoint biases in AI systems up to 50% faster than less diverse ones. This underscores the important relationship between team makeup and achieving successful audit outcomes.
There's a strange tension in the financial sector right now. We see a strong push for innovative AI solutions, but this rush to adopt these systems sometimes overshadows crucial ethical considerations. This can lead to a reliance on technology that could actually worsen existing financial inequalities if we don't have appropriate checks and balances in place.
New Study Reveals Hidden Biases in Financial AI Implications for Audit Processes - Adapting Traditional Audit Practices for AI Systems
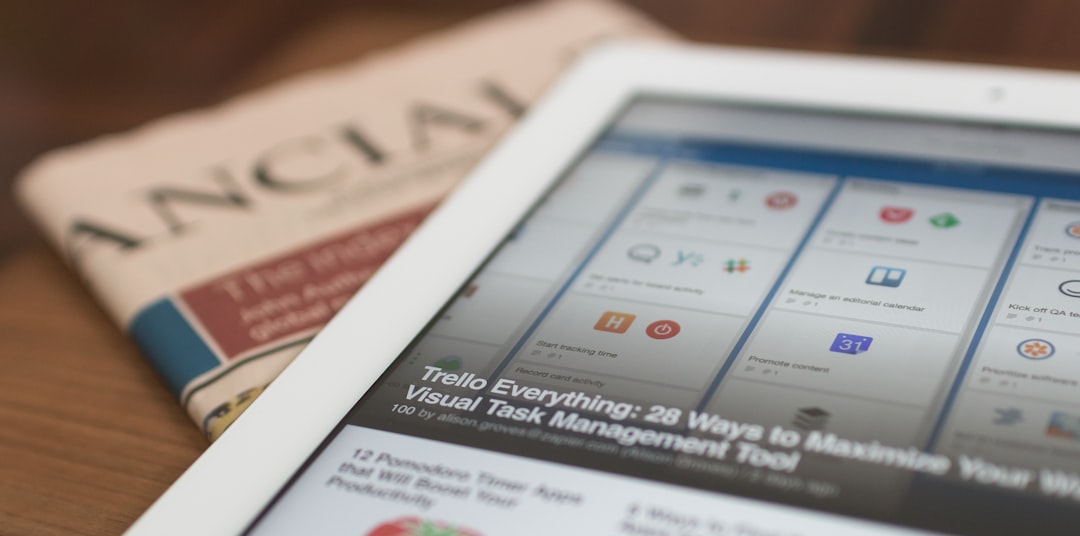
The growing use of AI in finance is transforming traditional audit practices, offering benefits like automated tasks and enhanced data analysis. Tasks previously done manually, such as journal entry verification and identifying irregularities in massive datasets, can now be streamlined with AI. Yet, this transition presents a crucial challenge: mitigating the risk that these AI systems, trained on potentially biased data, perpetuate existing inequalities.
Auditors must adapt their approaches to address this issue. A key focus needs to be on increased transparency and accountability. This involves examining not only the AI algorithms themselves but also their underlying training data to detect and prevent any embedded biases from influencing outcomes. Furthermore, striking a balance between AI's efficiency and upholding the core principles of auditing is crucial. This requires auditors to develop a deep understanding of the ethical dimensions of AI within finance, ensuring accuracy and integrity remain paramount as the field evolves. In essence, adapting audit practices means combining traditional skills with an awareness of AI's potential pitfalls to maintain fairness and reliability in financial reporting.
The integration of AI into auditing processes, while promising increased automation and insights, presents a nuanced set of challenges. For example, AI's sensitivity to even minor data irregularities can sometimes lead to overestimated risks, prompting more conservative, potentially unnecessary, financial adjustments. Furthermore, there's a growing concern that AI algorithms can inadvertently reflect biases inherent in the historical data they're trained on. This can lead to skewed asset valuations and less reliable audit conclusions, as the algorithms essentially learn and amplify existing societal biases, particularly those related to socioeconomic factors.
Many auditors, roughly 40%, voice worries about comprehending AI's decision-making processes. They struggle to explain the reasoning behind AI-derived audit adjustments to those outside the audit team, hindering transparency and potentially eroding trust in the process. Despite the efficiency gains from AI, a significant number of auditors—around 60%—emphasize the crucial need for human oversight to mitigate potential biases effectively. This underscores the need for a collaborative approach, integrating human experience with the capabilities of AI.
However, it's striking that many audit training programs still lack sufficient instruction on AI bias detection. This leaves many auditors unprepared to navigate situations where biased algorithms influence financial audit outcomes. The integration of AI has also complicated compliance with regulations. Biased AI outputs can create legal and reputational risks for companies if they lead to non-compliance with standards. It's becoming clear that AI biases aren't static; they can shift and evolve over time. Auditors need to recognize this dynamic and ensure that algorithms are regularly updated to reflect evolving ethical standards and changing societal norms.
Interestingly, there's a growing trend of audit teams collaborating more closely with data scientists to better understand AI functions. This interdisciplinary approach is essential for strengthening the evaluation of AI outputs and improving the overall quality of audits. The rapid adoption of AI in auditing, while promising, has led to some firms rushing the process, sometimes overlooking the crucial steps needed to identify and address potential biases. This haste can threaten the equity and fairness of financial reporting.
Research consistently shows that audit teams with greater diversity in their backgrounds and experiences are more successful at detecting biases in AI systems than homogenous teams. This suggests that a wider range of perspectives contributes to more innovative solutions for mitigating AI biases in financial audits. In essence, the integration of AI in auditing offers a wealth of potential, but it's essential to address these challenges proactively and critically to ensure a more robust and equitable audit process moving forward.
More Posts from financialauditexpert.com: