The Financial Auditor's Guide to Privacy-Enhancing Technologies in 2024 Balancing Data Utility and Protection
The Financial Auditor's Guide to Privacy-Enhancing Technologies in 2024 Balancing Data Utility and Protection - AI-Powered Auditing Tools Enhancing Efficiency and Accuracy in 2024
The year 2024 marks a significant shift in the auditing landscape, driven by the integration of AI-powered tools. These tools, utilizing machine learning and advanced analytics, are fundamentally changing how audits are conducted, primarily by enhancing efficiency and accuracy. Auditors are now capable of analyzing entire datasets, uncovering insights previously hidden within large volumes of information, which was previously impossible or impractical. This enhanced data analysis capability allows for a more comprehensive and thorough assessment of financial data. The automation of repetitive tasks, a key benefit of AI, empowers auditors to shift their focus from tedious manual processes towards interpreting complex patterns and drawing insightful conclusions from the data. This is increasingly in demand by businesses who recognize the value of streamlined and efficient audits. Despite the evident advantages, the adoption of AI in auditing is not without its hurdles. Concerns around the availability of skilled professionals and the significant investments required for implementation are persistent obstacles. Ultimately, auditors, as crucial advisors, find themselves at a critical juncture. They must not only embrace and effectively manage the capabilities of AI-powered tools but also ensure these powerful technologies are responsibly deployed, upholding data privacy in a world where digital data is the foundation of business.
In the evolving landscape of auditing, AI is increasingly influencing how audits are performed in 2024. Tools now leverage AI and machine learning to sift through massive datasets, including transactional records, allowing for audits that used to take weeks to be completed in a fraction of the time. This speed increase is changing how we think about audit timelines.
Furthermore, the incorporation of machine learning is not just about finding unusual transactions; these systems are starting to anticipate potential risks, allowing auditors to concentrate on the highest-risk areas before significant problems emerge. Natural language processing is also expanding the scope of what can be audited. Systems can now parse unstructured data, such as emails and contracts, going beyond the traditionally limited scope of just numerical data.
Interestingly, AI is pushing the idea of continuous auditing—essentially a constant monitoring of an organization’s financial health. This is a departure from the traditional periodic audits, which can miss real-time issues. This continuous monitoring aspect is interesting to consider. AI algorithms are also learning with each audit. The idea that each audit cycle can lead to a more refined ability to spot errors and non-compliance is potentially quite beneficial, creating a virtuous cycle of improvement.
Another notable aspect of these AI tools is their enhanced ability to identify fraud. AI models are becoming better at recognizing patterns and behaviors often associated with fraud, potentially outperforming human auditors at recognizing these signs. We also see an interesting tradeoff between automation and human subjectivity. AI systems rely on defined algorithms, eliminating the possibility of human biases that could influence an audit. This aspect might be debated in the long run, but is an interesting current trend.
The predictive capabilities of AI within these tools are quite notable. The ability to model various scenarios and anticipate possible future financial situations is an interesting new possibility. This allows organizations to take a proactive approach to risk management rather than simply reacting to what's already happened. Also, AI-driven tools are becoming better at handling regulatory changes automatically, potentially reducing compliance risks and the potential for financial penalties.
Finally, the reports generated by AI audit tools are evolving too. They are now not just static summaries but include detailed insights and even practical recommendations for action. This ability to directly translate audit findings into useful insights is likely to improve decision-making within organizations and make audit results more impactful. While we still need to examine the validity of such insights in real-world scenarios, it's a promising direction for the future. Overall, it's evident that AI is starting to revolutionize auditing and that these changes are leading to some interesting new trends and possible consequences for the field that will need to be carefully examined.
The Financial Auditor's Guide to Privacy-Enhancing Technologies in 2024 Balancing Data Utility and Protection - Implementing Homomorphic Encryption for Secure Data Analysis
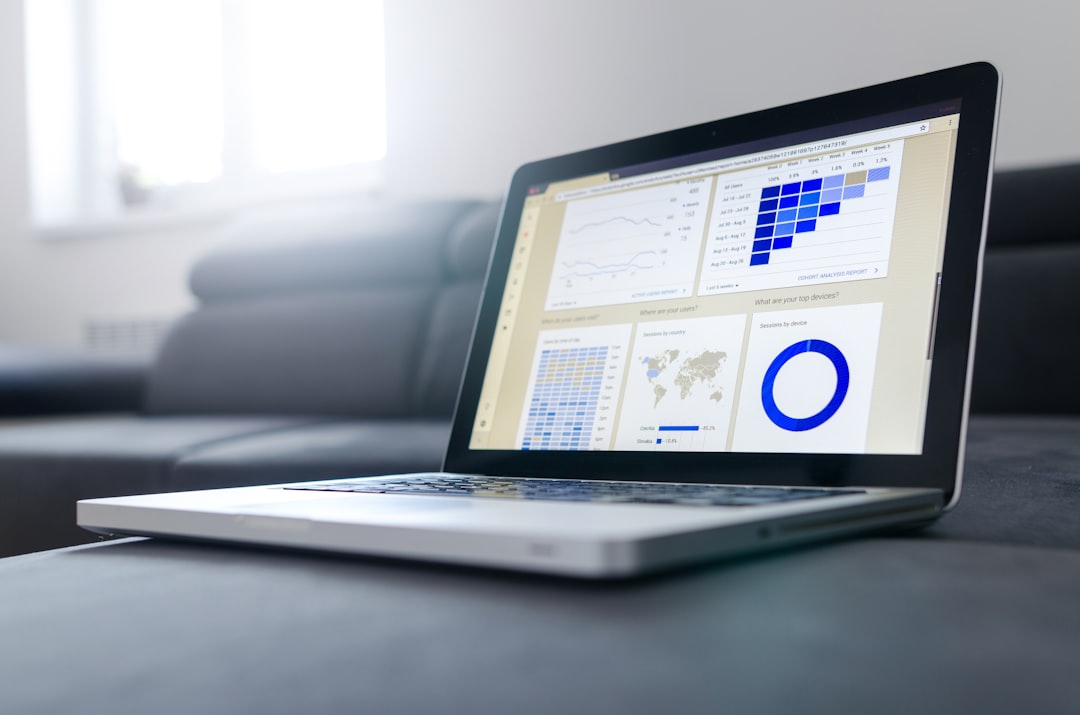
Implementing homomorphic encryption presents a compelling approach to secure data analysis, especially in domains like finance where data privacy is critical. This technique enables calculations to be performed directly on encrypted data, thereby safeguarding sensitive information while allowing it to be used for analysis and decision-making. As financial institutions increasingly leverage sophisticated data analytics for tasks like risk management and fraud detection, homomorphic encryption can contribute to preserving data utility without compromising the confidentiality of underlying information. However, fully realizing the benefits of this technology isn't without its hurdles. The intricacy and computational requirements associated with this encryption method can present significant obstacles that need careful attention. Ultimately, as the field develops, the interplay between robust data protection and the capacity for insightful analysis will likely define the future of financial auditing.
Homomorphic encryption presents a fascinating approach to securing data analysis, allowing calculations to be performed on encrypted data without needing to decrypt it first. It essentially provides a way to keep data protected while still gaining insights. This is achieved through complex mathematical underpinnings, often based on lattice-based cryptography. However, this mathematical sophistication comes at a price. The encryption and decryption processes themselves can be quite resource intensive, leading to a noticeable performance hit during analysis. It's a classic tradeoff between security and speed.
Furthermore, not every type of mathematical operation is easily adaptable to encrypted data. While basic operations like addition and multiplication are fairly manageable, more involved operations can pose challenges. This can limit the kinds of analysis that auditors are able to conduct directly on the encrypted data.
The reliability of homomorphic encryption is very dependent on secure key management. If those keys are lost or compromised, the entire system is compromised. This highlights the importance of robust and carefully designed protocols for managing these keys.
Interestingly, we're seeing more adoption of homomorphic encryption across several industries, like finance and healthcare. This isn't just about regulatory compliance, though that is certainly a factor, but also about fostering user trust. Businesses seem to be recognizing the value in proactively addressing data privacy alongside their typical operational demands.
Organizations are experimenting with hybrid solutions that combine homomorphic encryption with other encryption methods. This approach is an attempt to strike a balance—gaining performance boosts without compromising the strong security offered by homomorphic encryption.
While homomorphic encryption offers robust data privacy, it's not a magical solution for complete anonymity. It's possible that analyses on the encrypted data might still reveal some patterns that could expose sensitive information. This suggests a need for careful consideration of privacy beyond just encryption.
There's also a growing ecosystem of open-source tools supporting homomorphic encryption, which is beneficial for encouraging research and experimentation. However, these libraries can be a mixed bag. Some may not be as well-documented, and security features might not always be as polished. This can create hurdles when integrating such tools into existing systems.
The legal landscape around homomorphic encryption is still somewhat undefined. As audit practices continue to adapt, the laws and regulations guiding the use of encrypted data will likely change as well. This creates a dynamic environment where auditors need to stay informed on the latest developments.
Some newer homomorphic encryption designs are being developed with an eye towards quantum computing resistance. This is an intriguing development, given the potential for quantum computers to break some traditional encryption methods. This forward-thinking approach is crucial as we consider the long-term security implications of various technologies.
The Financial Auditor's Guide to Privacy-Enhancing Technologies in 2024 Balancing Data Utility and Protection - Blockchain Integration in Financial Auditing Processes
Blockchain's integration into financial auditing offers a significant shift towards increased transparency, efficiency, and data security. Utilizing features like immutability and decentralized record-keeping inherent to blockchain, auditors can potentially bolster the trustworthiness of financial reporting and refine audit procedures. However, challenges emerge, notably in navigating data privacy regulations, necessitating a delicate balance between innovation and regulatory compliance. As blockchain's adoption in finance develops, both internal and external auditors become increasingly crucial in reinforcing trust and sound governance. Ongoing discussions surrounding transparency, trust, and governance will be vital in navigating the evolving implications of blockchain in auditing. The journey towards widespread adoption is still unfolding, with considerable considerations and obstacles remaining.
Blockchain, with its inherent features of secure and distributed data storage, holds promise for revolutionizing financial auditing processes. By recording transactions in an immutable and transparent way, it allows auditors to easily verify and trace the history of financial data. This real-time access to records can streamline the audit process, potentially reducing the time it takes to complete an audit from weeks to a matter of moments. Furthermore, the use of smart contracts, automated agreements built into the blockchain, can automate compliance checks, potentially eliminating the need for many manual procedures.
However, the distributed nature of blockchain presents its own set of challenges. While increasing the security and integrity of financial records, ensuring compliance with existing data privacy regulations such as GDPR can be complicated. The need to strike a balance between the inherent transparency of the technology and the necessary confidentiality of sensitive data poses a considerable hurdle to adoption.
Blockchain's inherent features of immutability and a chronological record of transactions can improve the reliability and trust in the accuracy of financial accounting practices. This can lead to more efficient decision-making and a more solid foundation for establishing financial trust. Auditing practices themselves are in a transitional phase with the rise of blockchain. The shift to automated and real-time auditing is likely to change the skills needed by auditors, potentially requiring a new set of expertise in the intricacies of blockchain technologies and its underlying principles.
Despite some organizations identifying blockchain as a priority, as shown in Deloitte's 2019 Global Blockchain Survey, widespread adoption is not yet widespread. Research, as seen in a bibliometric study of 67 articles, suggests that core themes in blockchain's application to auditing center on issues of governance, transparency, and trust. The integration of blockchain is poised to transform how financial reporting is conducted, leading to faster and potentially more accurate audits. This applies to both external and internal auditors, as both roles share the responsibility of providing assurance and strengthening risk management through improved governance.
The journey of blockchain into financial auditing is not without its pitfalls. We need to consider what happens if inaccurate data is initially entered into the blockchain. Because of immutability, errors can become part of the permanent record, necessitating robust controls and validation procedures at the data input stage. Also, we need to consider that the combination of blockchain and AI presents an intriguing avenue for enhancing the capabilities of anomaly detection and improving the sophistication of predictive auditing tools. This potential development can lead to a fundamental change in how audits are designed and performed.
Ultimately, the effective implementation of blockchain technologies in auditing will depend on how well we address concerns about data protection and privacy. Privacy-enhancing technologies will play a key role in establishing a sustainable and trustworthy framework for the use of blockchain in financial auditing, allowing for data utility and confidentiality to be maintained. This balance will be critical as blockchain technologies continue to evolve and integrate into the practices of financial auditing.
The Financial Auditor's Guide to Privacy-Enhancing Technologies in 2024 Balancing Data Utility and Protection - Federated Learning Techniques for Collaborative Audits Across Institutions
Federated learning presents a new way for financial institutions to work together on audits, particularly in the area of fraud detection, while also protecting sensitive information. This approach lets organizations train machine learning models without directly sharing their raw data, promoting a decentralized system that emphasizes privacy. However, putting these techniques into practice can be complex, especially in finance where data often exists in separate, fragmented pieces, both across different data silos within an institution and between institutions. New tools, like the Starlit mechanism, aim to make it easier to use federated learning for fraud detection across multiple institutions, but the finance industry remains cautious about taking risks with innovative solutions in the face of ever-increasing financial crime. Over time, as federated learning technology matures, it holds the potential not only to improve the detection of unusual patterns that may indicate fraud across many institutions but also to change the overall nature of how audits are conducted by promoting collaboration while also firmly preserving data privacy. The long-term impact of federated learning on financial auditing needs to be carefully considered.
Federated learning (FL) presents an intriguing approach to collaboration in the financial auditing space, especially given the increasing need for both data utility and robust protection in 2024. Essentially, FL lets institutions work together to train machine learning models without needing to directly share their sensitive data. This decentralized approach aligns well with the privacy requirements of financial data, particularly with regulations like GDPR and CCPA.
One of the appealing aspects of FL is that it eliminates the need for a central data repository. Instead of sending all the data to a single server, which could be a security and compliance nightmare, FL allows each institution to train their own local models on their own data. This reduces the risk of data breaches, lowers costs associated with data transfer, and can expedite model training. This faster training is crucial in fields like finance where situations change quickly and insights are needed promptly.
However, the application of FL in financial anomaly detection is a bit more complex. Financial data is often partitioned in intricate ways, both horizontally (different institutions) and vertically (different departments within one institution), creating unique challenges for standard FL techniques. That said, there are solutions emerging, like the 'Starlit' mechanism, which seems to be a promising approach to address the scalability issue of FL in complex fraud detection tasks.
Interestingly, financial institutions are experiencing a surge in financial crime, with reports indicating an average of $102 million lost per institution in scams. This has understandably made institutions more hesitant to adopt new solutions. At the same time, it has emphasized the need for robust systems. Traditional fraud detection methods rely on locally-trained AI models, but those models may miss sophisticated fraud schemes that extend across different institutions. Here, FL potentially offers an advantage. By pooling knowledge from multiple institutions through a federated model, we might achieve a more accurate representation of the broader landscape of financial transactions. This leads to better anomaly detection.
But there are more benefits than just anomaly detection. For example, with novel representation learning techniques enabled by deep learning, there is the possibility of automated feature engineering for audit data without the need for extensive human intervention. This is useful because data can be highly specific to an industry or institution. Also, FL, being a form of privacy-preserving machine learning, can assist in navigating the tricky balance between contextualizing data and protecting user privacy during collaborative audits. We are currently in a phase of evolution of FL techniques, with new developments potentially leading to more effective tools to tackle increasingly complex fraud scenarios.
The application of FL in financial audits is being explored in research with experimental frameworks that utilize publicly available financial payment datasets. The outcomes of these experiments will be critical in understanding the real-world performance of FL in this specific domain. We are still in the early stages, but it's a potentially powerful technique that is worth keeping an eye on, especially as financial institutions navigate the evolving risks of the digital age.
The Financial Auditor's Guide to Privacy-Enhancing Technologies in 2024 Balancing Data Utility and Protection - Differential Privacy Approaches to Protect Individual Financial Records
Differential privacy offers a robust framework for protecting the privacy of individuals whose financial records are being analyzed. It's a newer approach that enhances traditional methods of anonymization by reducing the risk of re-identification, making data sharing more secure. This framework mathematically defines and quantifies the risk to individuals when data is collected and shared, providing a measure for ensuring compliance with emerging privacy regulations. However, the effectiveness of differential privacy comes with a necessary compromise between the level of privacy achieved and how useful the data remains for analysis. This balancing act is a primary consideration for financial organizations as they integrate data privacy into their practices. Given the growing reliance on privacy-enhancing technologies within financial auditing, differential privacy is a technology to watch, as it will likely play a key role in how future auditing processes are designed and managed.
Differential privacy offers a way to add carefully controlled randomness to the results of data queries, making it impossible to pinpoint any single individual's information with absolute certainty. This novel approach is particularly valuable for financial data, where individuals are rightfully cautious about their data being exposed.
The relatively new idea of "epsilon-differential privacy" helps put a number on the privacy loss when sharing data. It creates a standard that dictates how much noise should be added to datasets to protect sensitive information while still allowing the data to be useful for financial analysis. This is a way of quantifying privacy, which is quite helpful.
Interestingly, differential privacy can be applied not just when storing data but also when analyzing it. Financial institutions can now use sensitive datasets to generate insights without revealing the details of any individual's records, which is crucial for industries like finance with stringent regulations.
When financial audits involve data analysis, differential privacy provides a framework to ensure that statistical queries give accurate results without betraying any single transaction's specifics. This is essential for auditors who need to utilize data analytics while minimizing risks to individuals' privacy.
The success of differential privacy depends on carefully managing the 'privacy budget', a model that limits how much information can be deduced about any single person in the data. This relationship between privacy budgets and the usability of data is an active area of research that challenges traditional ways of thinking about financial data.
However, implementing differential privacy often leads to a difficult balancing act. Too much noise makes the data less useful, while not enough can lead to privacy breaches. This delicate tradeoff makes it a complex issue when considering its use in financial audits.
As more organizations turn to machine learning for fraud detection, differential privacy allows these systems to learn from large datasets while protecting the details of individual transactions. This effectively helps promote innovation without sacrificing privacy, a very interesting combination.
The applications of differential privacy aren't limited to just querying data. It can also improve the aggregation of data during the generation of financial reports. This allows institutions to develop comprehensive insights without having to expose any specific data points from individuals.
Researchers are also exploring how to combine differential privacy with blockchain technology. The goal is to leverage the secure ledger properties of blockchain while using differential privacy to protect user data during transactions. This combination holds significant potential to revolutionize financial applications that handle sensitive information.
While differential privacy is promising, its adoption in the finance world has been relatively slow. This is partly due to the complexity of implementing the technology and the need for trained professionals, creating a gap between the potential of the technology and its real-world application in financial auditing.
The Financial Auditor's Guide to Privacy-Enhancing Technologies in 2024 Balancing Data Utility and Protection - Regulatory Compliance and PETs The Intersection of GDPR, CCPA, and Emerging Laws
In the evolving regulatory landscape, particularly with the impact of GDPR, CCPA, and other emerging privacy laws, financial institutions are under increasing pressure to ensure data protection while also maximizing the value of their data. Privacy-enhancing technologies (PETs) have emerged as crucial tools in this environment, allowing for the sharing and analysis of information in a way that protects individual privacy. Simply checking off compliance boxes isn't sufficient; organizations must embed a culture of responsible data handling and trust into their operations.
The intersection of these emerging laws and PETs creates a complex environment for organizations. Auditors must understand how to effectively navigate this complex terrain, ensuring that data privacy remains a core principle of operations. The regulatory landscape is in constant flux, demanding continual adjustments and the integration of new PETs into established processes. Financial auditors will need to remain adaptive to balance the value of data with the need to protect individual privacy as they perform their duties.
Privacy-enhancing technologies (PETs) are becoming increasingly important as organizations navigate the complex and evolving landscape of data privacy regulations. The General Data Protection Regulation (GDPR) in the EU and the California Consumer Privacy Act (CCPA) in the US, while both focused on data subject rights, offer different approaches to achieving them. This difference highlights a key challenge: complying with a patchwork of global privacy regulations that often have conflicting or overlapping requirements. Countries like Brazil and India are also introducing their own data protection laws, making the landscape even more complex and requiring companies to stay nimble and adapt quickly.
The potential penalties for non-compliance are also a driving force behind the adoption of PETs. Fines for GDPR violations can be substantial, reaching up to a significant percentage of global revenue, making data privacy a serious financial concern for businesses. But it's not just about avoiding fines; there's also a growing market demand for PETs from consumers, who are increasingly valuing companies that prioritize data protection. This demand puts pressure on companies to go beyond just compliance and actively demonstrate their commitment to responsible data practices.
The GDPR's focus on "data minimization"—limiting the collection of personal data to only what's strictly necessary—has pushed developers of PETs to create tools that enable more efficient and targeted data collection, reducing the potential for accidental data exposure or inappropriate use. This emphasis on minimizing data has fundamentally changed how companies approach product development and service delivery, aligning with the "privacy by design" mandate within GDPR, which requires embedding data protection measures from the initial stages of design.
This evolving landscape places a new responsibility on financial auditors. They are no longer just focused on compliance but are becoming integral advisors in ensuring data protection. This often means getting involved in the early stages of new technology developments to ensure alignment with existing and emerging privacy laws.
Federated learning is an example of a promising PET that aligns well with these emerging regulatory requirements. It allows institutions to collaborate on machine learning models for tasks like fraud detection without directly sharing their sensitive data. This addresses core concerns under GDPR and CCPA, while still allowing the institutions to achieve shared gains in data analysis and insights.
However, the path to compliance is not always straightforward. Recent enforcement actions and lawsuits show that regulatory agencies are becoming increasingly proactive in overseeing data practices. Organizations must continually update their compliance frameworks to stay ahead of these changes. Moreover, the use of advanced technologies for data processing in finance only increases the complexity of adhering to these regulations. This underscores the critical need for robust auditing mechanisms that bridge the gap between regulatory compliance and the practical use of innovative technologies within the financial sector.
This ongoing evolution of technology and legislation creates a dynamic and fascinating space for researchers to explore. Finding the balance between allowing organizations to leverage the power of data while ensuring that the privacy of individuals is protected will be a continual challenge and require careful consideration of these complex interactions.
More Posts from financialauditexpert.com: