eDiscovery, financial audits, and regulatory compliance - streamline your processes and boost accuracy with AI-powered financial analysis (Get started for free)
Assessing AI's Real-World Impact on Financial Audit Compliance and Efficiency
Assessing AI's Real-World Impact on Financial Audit Compliance and Efficiency - Current AI Footprint in Audit Workflows
As of May 2025, artificial intelligence has become a more tangible presence within audit workflows, moving beyond theoretical potential to active implementation in various aspects of the audit process. Many firms are now leveraging AI tools to enhance data analysis capabilities, refine risk assessment procedures, and explore continuous monitoring techniques. The aim is to automate repetitive tasks, uncover deeper insights from increasingly complex datasets, identify anomalies more effectively, and ultimately support auditor decision-making and improve efficiency throughout engagement phases, from planning through fieldwork. However, the degree to which AI is integrated varies significantly across the profession. While some organizations are piloting or selectively adopting AI for specific use cases, others remain hesitant or are still in early planning stages, particularly concerning its broader application in financial reporting assurance. This uneven adoption highlights that while AI offers substantial potential benefits for improving compliance and speed, the practical challenges of deployment, ensuring audit quality, and maintaining integrity in a rapidly evolving technological landscape require careful, ongoing critical assessment in real-world audit scenarios. The current landscape therefore reflects a significant shift, characterized by active exploration and partial implementation, but not yet uniform or complete transformation across all audit activities.
Examining the current state of AI integration across financial audit tasks reveals a landscape still under construction, yet with some discernible shifts becoming apparent as of mid-2025. Automated continuous monitoring tools, for instance, are showing improved performance, with systems targeting areas like accounts payable reportedly achieving anomaly detection rates in the low nineties percentile. This represents a notable step up from the accuracy levels commonly observed in older, rule-based systems a couple of years prior, though the challenge remains in fine-tuning these to reduce false positives and understand the types of anomalies still being missed.
Within the daily grind, we're seeing AI-assisted functionalities creeping into the workflows of less experienced staff. It's estimated that roughly half of junior auditors are now interacting with tools designed to help with preliminary risk assessments. While proponents claim this speeds up the initial scoping phase, potentially by a fifth, the crucial question for observers is how effectively these individuals are learning foundational skills when some early analysis is abstracted away.
One area showing significant potential lies in navigating complex compliance landscapes. AI is being trained on extensive libraries of global regulations, aiming to flag potential deviations with a granularity that might be difficult for a human auditor to maintain across multiple jurisdictions. This capability is still maturing, and verifying the system's interpretation against specific business contexts remains a necessary step, highlighting that AI serves more as a powerful search and pattern-matching engine here than a final decision-maker.
An interesting, perhaps counterintuitive, observation is the impact on staffing dynamics. While there were early concerns about widespread job displacement, the reality unfolding appears to involve a modest *increase* in the demand for experienced senior auditors. Their expertise is proving vital for critically validating the outputs and insights generated by AI systems, acting as a necessary layer of human judgment and quality control, rather than AI simply replacing their analytical role.
Finally, the capabilities of AI are extending beyond simple data crunching. Advanced language models are starting to assist with drafting routine audit documentation or narrative summaries for straightforward findings. The intent is clearly to free up auditors from mundane writing tasks so they can concentrate on more complex, judgmental areas requiring human insight and communication. However, relying on auto-generated text necessitates rigorous review to ensure accuracy, appropriate tone, and completeness, suggesting this is more about augmentation than full automation of reporting.
Assessing AI's Real-World Impact on Financial Audit Compliance and Efficiency - How AI Tools Influence Compliance Procedures
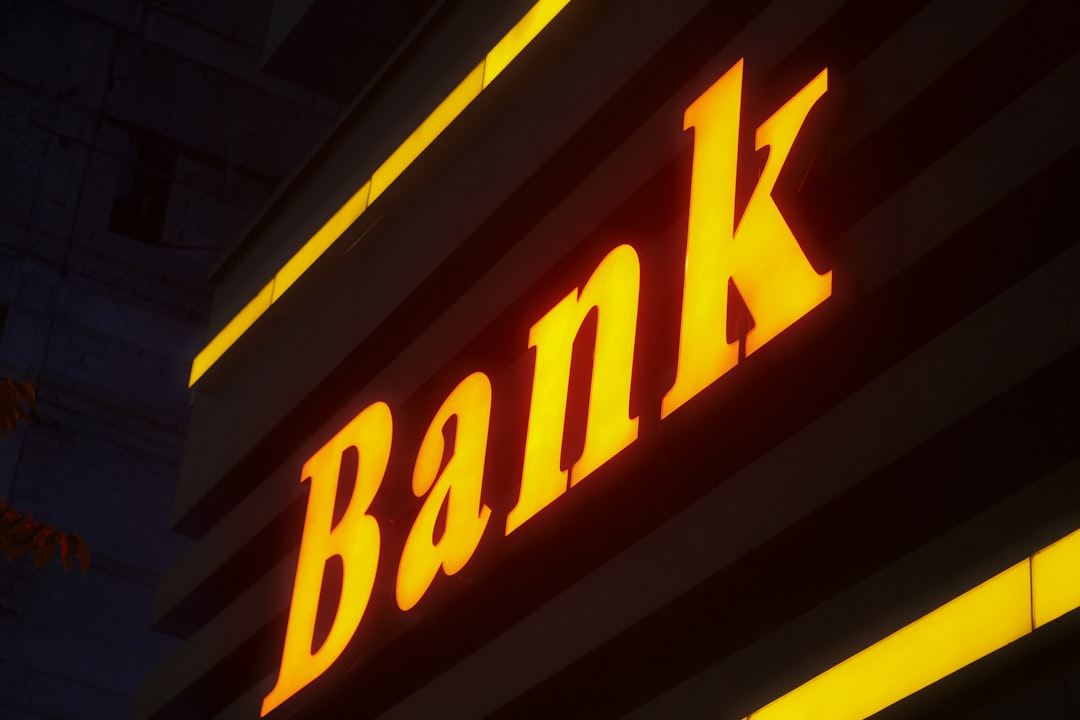
The increasing integration of AI tools is significantly reshaping how organizations manage their compliance responsibilities. Efficiency gains are being achieved through the automation of various routine compliance functions, notably in the analysis and monitoring of regulatory information and the identification of potential risks. This shift aims to allow compliance professionals to concentrate their efforts on more complex, high-judgment matters and strategic advisory. However, embedding AI into compliance processes introduces considerable challenges; the intricate nature of these systems can sometimes obscure the logic behind their outputs, creating difficulties in satisfying regulatory demands for transparency and explainability. Furthermore, the dual evolution of both AI capabilities and regulatory frameworks requires constant, diligent re-evaluation of risks associated with AI deployment. While AI offers clear potential for streamlining compliance activities, implementing it demands a critical perspective, acknowledging that human expertise and oversight remain an essential component for effective risk management and ensuring adherence to the rules in practice as of mid-2025.
Investigating how AI is currently influencing specific compliance procedures within the financial audit context reveals several distinct patterns of application as of mid-2025.
AI systems capable of processing vast amounts of documentation and extracting or classifying specific relevant information are reportedly accelerating initial review steps. Claims of significant time reductions in this preliminary screening process highlight AI's utility for focused information retrieval, though verifying the accuracy and completeness of the extracted data across diverse document formats remains a necessary step requiring human oversight.
Machine learning models are being developed and trained on historical compliance data to identify patterns that may correlate with past issues. The aim is to move beyond simply detecting known violations to proactively assessing the potential likelihood of future non-compliance based on learned associations from historical events, a probabilistic approach that inherently depends on the quality and representativeness of the training data.
Tools utilizing natural language processing are being employed to continuously monitor external regulatory updates and internal policies. This capability seeks to dynamically identify proposed or enacted changes and their potential impact, aiming to bridge the gap between shifting regulations and the necessary updates to internal compliance frameworks more rapidly than manual processes typically allow, although the interpretation and integration of these changes still demand expert human judgment.
Algorithms are being explored for their potential to analyze communication patterns or identify specific terms within internal data streams that might statistically indicate potential ethical or regulatory concerns. While offering a new avenue for identifying risks that might be missed in traditional reviews, the implementation of such monitoring capabilities necessitates careful consideration of privacy implications and the management of potential false positives that could incorrectly flag innocuous communications.
There is also the widely discussed potential for AI to handle certain high-volume, repetitive data processing tasks, freeing up auditor capacity. The underlying hypothesis is that this time saved could be redirected towards activities that fundamentally require human cognitive strengths – engaging in complex discussions with management, performing qualitative assessments in ambiguous areas, or exercising professional skepticism and judgment in nuanced situations. Realizing this benefit fully relies on how effectively AI output integrates into these higher-level tasks and if it genuinely provides the cognitive capacity required for deeper human analysis.
Assessing AI's Real-World Impact on Financial Audit Compliance and Efficiency - Assessing Actual Time Savings from AI Use
As of May 2025, evaluating the concrete time savings delivered by AI tools in financial auditing is a growing area of scrutiny. While there are observations of AI enabling faster execution of certain tasks, notably those involving high-volume data processing or preliminary checks, the impact on total engagement time is less clear-cut. Reports indicate efficiencies in defined workflow segments like initial document analysis or supporting junior staff in basic risk identification. However, realizing net time reductions faces challenges, primarily due to the persistent need for human oversight and critical review of AI-generated insights or outputs, particularly in judgmental areas or when complexity increases. The fundamental question for many firms is whether these localized speedups genuinely free up valuable auditor time for higher-level analytical work and critical thinking, or if the effort previously spent on data processing is simply being repurposed for validating AI results and managing the AI tools themselves, demanding a nuanced assessment of actual productivity gains.
Observation from the field as of May 2025 suggests that assessing the genuine time dividends reaped from integrating AI into financial audit processes presents a nuanced picture, often less straightforward than initial projections might imply.
First, while AI tools readily manage the heavy lifting of initial data ingestion and pattern identification across large datasets, the final stages – validating exceptions, reconciling outputs against source documents, and translating findings into auditable conclusions – frequently remain manual-intensive. This "last mile" of the process, requiring significant human judgment and verification, can consume a substantial portion of the total effort, potentially masking the efficiencies gained earlier in the workflow, particularly when dealing with intricate or ambiguous transactions.
Second, the operational overhead associated with maintaining and effectively deploying these systems is proving more demanding than initially estimated. Beyond the upfront implementation, the ongoing effort dedicated to curating training data, fine-tuning models for specific entity nuances, and crucially, ensuring audit staff are adequately skilled to interact critically with evolving AI capabilities represents a continuous time sink that wasn't always fully factored into efficiency forecasts.
Third, there's an observable cognitive penalty from workflow fragmentation. Auditors often find themselves needing to switch contexts between reviewing AI-generated analysis or flags within one system and then transitioning to separate, traditional audit tools or source systems for deeper investigation and documentation. This back-and-forth, while seemingly minor, introduces frictional losses in concentration and workflow momentum that can collectively erode potential time savings.
Fourth, anecdotal evidence suggests instances of what might be termed "automation complacency" or "bias" setting in. Some auditors, particularly those less experienced, appear to exhibit a reduced inclination to question or independently verify AI-generated outputs when the results seem plausible on the surface. Identifying and rectifying errors stemming from this over-reliance downstream can unexpectedly introduce delays far greater than the time 'saved' by initial acceptance of the AI's suggestion.
Finally, empirical data from some early and extensive AI deployments indicates that the relationship between further investment in AI capabilities and corresponding increases in time efficiency is often non-linear. After achieving initial gains from foundational AI applications, subsequent efforts to automate increasingly complex or bespoke audit tasks appear to yield diminishing returns in terms of actual hours saved, suggesting a point of diminishing marginal utility for certain types of AI integration within current audit paradigms.
Assessing AI's Real-World Impact on Financial Audit Compliance and Efficiency - The Changing Skill Set for Audit Professionals
The capabilities now expected of financial audit professionals are undergoing a significant evolution driven by the increasing integration of artificial intelligence into workflows. While a deep understanding of accounting principles and audit methodologies remains foundational, it is no longer sufficient in isolation. Practitioners today must cultivate a more diverse range of abilities, effectively blending their core audit expertise with a growing fluency in technology and data analysis. This requires not only skill in utilizing AI-powered tools that process and analyze vast datasets but crucially, the critical judgment to interpret the results generated by these systems. Understanding the potential limitations, biases, and underlying logic of AI is becoming essential for validating findings and ensuring the reliability of audit evidence. Furthermore, success in this new landscape increasingly depends on the ability to collaborate effectively with data scientists and IT specialists, navigating complex technical environments and translating technological insights into auditable conclusions. Ultimately, the transforming profession demands professionals who can seamlessly integrate advanced technological capabilities with indispensable human skepticism and analytical prowess to uphold audit quality.
The introduction of artificial intelligence into audit workflows is necessitating a re-evaluation of the competencies required to perform effectively. Observations from the field as of mid-2025 suggest shifts in the needed skill set beyond merely understanding the technical functionality of new tools.
* The ability to effectively communicate complex insights derived from AI models to clients and stakeholders who may not possess a technical background is becoming increasingly critical. This involves translating statistical correlations and algorithmic outputs into actionable audit conclusions.
* Paradoxically, as AI excels at identifying patterns in data, the human auditor's need for deep, contextual understanding of specific industries and business models is amplified. This domain expertise is essential for interpreting the relevance and implications of AI-flagged anomalies or trends.
* Proficiency in areas related to data integrity, privacy regulations, and the ethical considerations of using AI – including recognizing potential algorithmic biases – is emerging as a foundational skill set for audit professionals placing reliance on these systems.
* A less discussed, yet vital, skill is the capacity for auditors to actively 'unlearn' and discard manual processes and traditional routines that AI is now capable of performing more efficiently, reorienting their focus to higher-value tasks.
* Cultivating a nuanced approach to professional skepticism is key; auditors must learn to critically evaluate not only the source data but also the AI's methodology and the reliability of its outputs, integrating these new inputs into their established judgmental framework.
Assessing AI's Real-World Impact on Financial Audit Compliance and Efficiency - Navigating the Challenges of AI Implementation
As financial auditing workflows increasingly look to incorporate artificial intelligence, navigating the process of putting these tools into practice is proving to be far from straightforward. While the potential for greater speed and better adherence to rules is clear, successfully embedding AI requires directly confronting several practical obstacles inherent in the implementation journey. A critical challenge lies in establishing and maintaining the necessary data quality infrastructure, as unreliable inputs will inevitably undermine the credibility of AI-generated insights and impact overall audit quality. Furthermore, the technical complexities of integrating new AI platforms seamlessly within existing, often fragmented, audit software ecosystems presents significant operational hurdles. Managing this integration work, coupled with the essential requirement for auditors to possess the skills and mandate to rigorously review and challenge what the AI produces, underscores that successful deployment isn't simply a matter of switching on software. Firms implementing AI must actively build frameworks to manage these technical and human elements together. The real value of AI in audit compliance and efficiency will ultimately depend on how effectively these foundational implementation challenges are navigated, requiring ongoing effort and a critically informed approach to managing the technology itself as of mid-2025.
Examining the actual journey of integrating artificial intelligence into financial audit processes reveals several practical hurdles often underestimated in initial planning, observed as of mid-2025.
One notable finding is that achieving truly "explainable AI" outputs that satisfy audit scrutiny and regulatory demands remains substantially more challenging and resource-intensive than anticipated. While models might provide technical justifications for their results, translating these into a narrative that clearly demonstrates *why* a specific audit assertion is supported or a risk identified, in a manner comprehensible and defensible to auditors, clients, and regulators, continues to be a significant operational and intellectual task.
Furthermore, the practical complexities of preparing and governing the necessary data for AI training and ongoing operation have proven unexpectedly arduous. Integrating datasets from disparate legacy systems, ensuring consistent data quality, meticulously documenting data lineage, and managing metadata for AI purposes often require a level of effort that consumes substantial project resources beyond the model development itself.
Experience is showing that a focused, incremental approach—deploying AI for narrowly defined tasks with clear objectives and metrics ("Minimum Viable AI")—tends to yield more tangible results and smoother organizational adoption compared to attempting comprehensive, transformative AI integrations across entire audit methodologies simultaneously. The former allows for quicker iteration and demonstrated value.
The required evolution of the audit professional's skill set is proving multifaceted; it’s not simply about technical AI proficiency. A critical emerging need is for individuals who understand the cognitive interaction between auditors and AI—how AI outputs might influence human judgment, the potential for subtle biases to manifest, and the sophisticated skepticism required to effectively leverage AI without undue reliance.
Finally, maintaining the integrity and relevance of AI models throughout a lengthy audit engagement requires continuous, non-trivial effort. As the audited entity's systems, processes, or underlying data patterns shift, keeping the AI models effectively calibrated through ongoing data curation and validation introduces an operational strain that must be explicitly factored into engagement timelines and resource allocation.
eDiscovery, financial audits, and regulatory compliance - streamline your processes and boost accuracy with AI-powered financial analysis (Get started for free)
More Posts from financialauditexpert.com: