eDiscovery, financial audits, and regulatory compliance - streamline your processes and boost accuracy with AI-powered financial analysis (Get started for free)
The Role of AI in Enhancing Anti-Money Laundering Compliance for Contract Review
The Role of AI in Enhancing Anti-Money Laundering Compliance for Contract Review - AI-Powered Pattern Recognition in Contract Analysis for AML Compliance
AI's ability to identify patterns within contract data is rapidly transforming AML compliance. These systems, using sophisticated algorithms, can dissect complex webs of transactions, offering a more powerful lens to spot hidden money laundering operations. The capacity to analyze huge volumes of data helps in conducting more thorough investigations while simultaneously reducing the number of false alarms that can bog down compliance efforts. This translates to a smoother process for reporting potentially suspicious activity. However, it's crucial to recognize that human judgment remains a critical component. AI can’t fully grasp the subtleties of risk assessment, nor can it consistently accommodate the varied approaches institutions take to AML. Ultimately, achieving effective AML compliance in this era necessitates a hybrid strategy that utilizes AI's strengths while acknowledging its boundaries.
Within the domain of contract analysis, AI can unveil intricate connections between parties, surfacing potential links that might suggest money laundering activities. It achieves this by leveraging natural language processing (NLP), essentially teaching machines to understand the nuances of legal text. This allows AI to extract key details that might easily be missed by human eyes during manual review, especially when sifting through huge quantities of contracts.
Interestingly, these systems can detect peculiar patterns in the financial dealings described within contracts. This capability is particularly valuable as it alerts compliance teams to potentially problematic transactions that might otherwise escape attention. The foundation of this ability lies in the system's capacity to learn from prior compliance cases, allowing it to refine its pattern recognition over time and decrease the frequency of false alarms. The training data for these AI models is often enormous, encompassing thousands of contracts. This large dataset provides a broad foundation for the system to generalize and identify unusual patterns.
While impressive, AI-powered contract analysis does offer a considerable speed advantage. Large batches of contracts, previously needing substantial human effort, can be processed within hours. This accelerated processing can greatly reduce the time needed for compliance checks. Yet, human involvement is not obsolete. The role of compliance teams remains pivotal in scrutinizing the AI-generated insights and ensuring the fulfillment of regulatory guidelines. AI can additionally monitor alterations and updates within contracts. This constant surveillance assists in detecting any revisions that could potentially mask unlawful financial dealings.
AI's integration in contract analysis holds promise in boosting the scalability of compliance activities. It empowers companies to handle an increasingly larger number of contracts without dramatically expanding their staff. However, it is crucial to acknowledge the constant evolution of legal language. The AI models must undergo continuous updates to stay current with new trends and terminologies. Failing to adapt could diminish their effectiveness in AML endeavors. This ongoing need for refinement poses a challenge for sustained effectiveness.
The Role of AI in Enhancing Anti-Money Laundering Compliance for Contract Review - Machine Learning Algorithms Streamline Due Diligence Processes
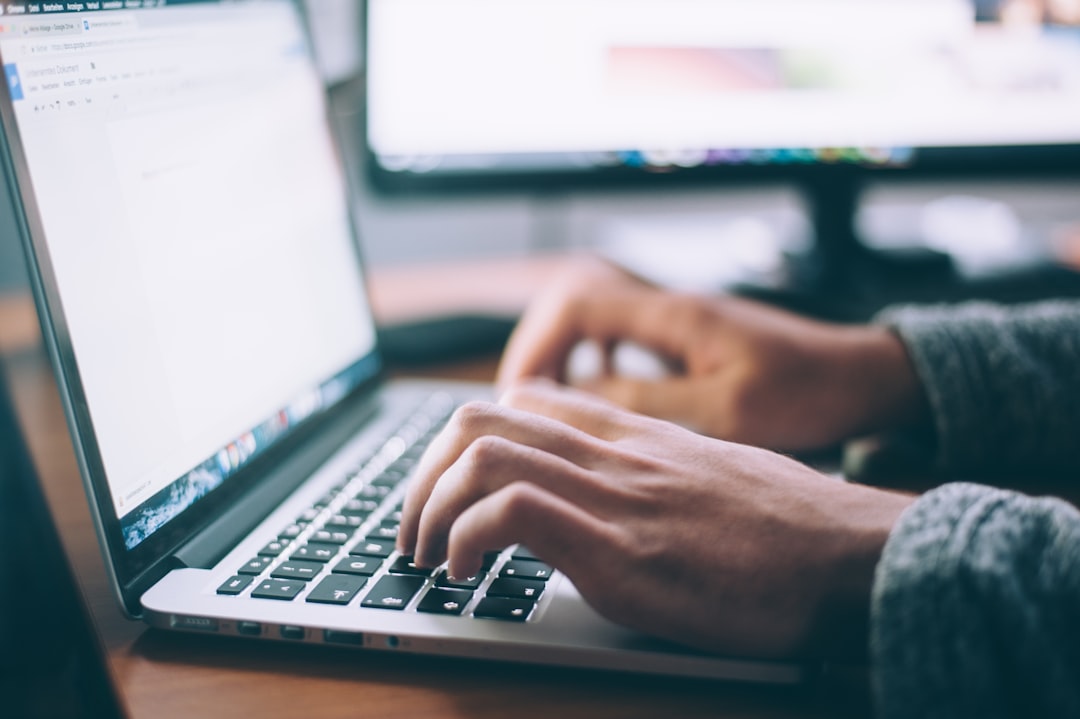
Machine learning algorithms are transforming how due diligence is performed, especially in areas like anti-money laundering compliance. These algorithms can automate tasks like verifying identities and monitoring transactions, making customer due diligence more efficient. By analyzing huge amounts of data rapidly, these algorithms can identify unusual patterns linked to potential money laundering much faster, resulting in better detection and fewer mistaken alerts that require manual review. Despite these significant benefits, it's crucial to remember that human judgment is still essential. There are complex financial risks that these systems simply aren't yet sophisticated enough to fully understand. A successful approach to compliance needs to integrate these tools while acknowledging their shortcomings to create a robust framework.
The surge in financial crime defense spending, reaching roughly $214 billion in 2020 alone, underscores the critical need for advanced solutions in combating money laundering. Machine learning (ML) is emerging as a powerful tool in this fight, particularly within customer due diligence processes. By automating tasks like identity verification and transaction monitoring, ML algorithms can significantly enhance anti-money laundering (AML) compliance efforts. This is especially crucial as criminals are also leveraging technological advancements to conceal their illicit activities, making traditional methods increasingly ineffective.
ML shines in real-time transaction monitoring, identifying unusual patterns that could signal money laundering attempts. Moreover, ML models can sift through the data to improve the accuracy of AML detection, reducing the frequency of false positives that often bog down compliance teams. These false alerts, which are essentially mistaken warnings requiring manual review, are a common pain point, and AI offers a potential path to resolving it. By learning from historical data and building up profiles of expected transaction behavior, sophisticated ML algorithms can streamline the AML process, freeing up compliance professionals to focus on more complex investigations.
Financial institutions are increasingly turning to ML to manage and analyze unstructured data—data that doesn't fit neatly into traditional databases. This is particularly relevant in AML, where a wealth of information exists in contracts, emails, and other text-based sources. It's an ongoing effort to utilize this wealth of data more effectively. The goal here is not just to adhere to legal standards, but also to optimize compliance processes and bolster overall risk management within the financial sector.
Researchers are actively exploring the intersection of AI and AML, incorporating advanced techniques like natural language processing and deep learning. These innovations are crucial for building the next generation of AML technologies. The evolution of money laundering schemes is a persistent challenge that threatens global financial security and stability. This constant struggle highlights the need for ongoing advancements in compliance tools.
While the potential of ML in AML is significant, it's not without its challenges. There's the risk of reinforcing existing biases in the data used to train these models, potentially leading to unfair or discriminatory outcomes in compliance decisions. It's a complex area, where we need to ensure that these systems are fair and don't inadvertently introduce new inequalities. Ultimately, a thoughtful and balanced approach that incorporates both the strengths of ML and human oversight is essential to ensuring the integrity and fairness of AML efforts moving forward.
The Role of AI in Enhancing Anti-Money Laundering Compliance for Contract Review - Natural Language Processing Enhances Contract Risk Assessment
Natural Language Processing (NLP) is significantly enhancing the evaluation of contract risks. Previously laborious manual analysis can now be automated, allowing for faster identification and classification of potentially problematic contract clauses. This not only increases the accuracy of risk assessment but also frees up valuable time and resources that can be used in other areas. While NLP undeniably boosts efficiency in this area, a balanced approach is critical. The technology must constantly evolve to remain effective in the face of changes in both legal language and regulatory frameworks. The reliance on AI highlights the ongoing need for human oversight to ensure a holistic and comprehensive approach to contract risk management.
Natural language processing (NLP) is evolving rapidly, allowing machines to understand the complexities of legal language with increasing precision. This improved understanding is crucial for contract risk assessments, especially within the context of anti-money laundering (AML) efforts. NLP's ability to grasp the nuances of legal jargon means it can now recognize subtle indicators of risk that might have been missed previously. The fact that NLP can now handle contracts written in multiple languages has significant implications for global finance, allowing for more thorough AML compliance in international business dealings. Moreover, it can go beyond simply understanding the words and analyze the tone and intent embedded in the text through a technique called sentiment analysis. This approach could offer unique insights into the motives of the parties involved, potentially highlighting potential risks.
One interesting area where NLP excels is in finding irregularities in the text. Instead of just picking up on typical contract language patterns, NLP algorithms can also spot anomalies. These unexpected deviations from norms can indicate unusual clauses or concealed modifications, giving us a fresh layer of scrutiny that human reviewers may overlook. Furthermore, NLP models are constantly being updated as they process new contracts. This continuous learning allows them to adapt to new legal trends and the evolving tactics used in money laundering, enhancing the models' ability to anticipate and identify potential problems.
Another exciting advancement is the ability to assign risk scores to individual clauses in a contract. NLP can leverage historical compliance data to evaluate the risk associated with specific clauses, providing a streamlined approach for prioritizing which clauses require more in-depth review by humans. It can also integrate this analysis with actual transaction data, which provides a much fuller picture of the risk landscape. It's a multifaceted approach to evaluation.
It's important to acknowledge that AI, including NLP, isn't without its limitations. One potential pitfall is the risk of the algorithms reflecting existing biases in the data they are trained on. This can lead to skewed assessments that might unfairly target certain groups. Researchers are working to ensure these biases are minimized, designing models that can identify and adjust for them. Furthermore, the output of these models can be challenging for compliance teams to interpret. To mitigate this, NLP can provide visualizations of complex contractual structures, making it easier for humans to understand the relationships and potential risks. Finally, NLP systems can monitor contracts for changes, quickly flagging any unusual alterations. Changes to sections dealing with financial transactions, for example, could indicate an attempt to conceal illicit activity.
This continuous evolution of NLP tools holds significant promise for improving the efficiency and accuracy of contract risk assessment within AML efforts. However, it's crucial to acknowledge the inherent complexities and biases that must be carefully managed in these systems. As researchers further refine NLP and its integration with broader AML compliance frameworks, we can expect to see ever more sophisticated tools that bolster the fight against financial crimes.
The Role of AI in Enhancing Anti-Money Laundering Compliance for Contract Review - Automated Red Flag Detection in Financial Agreements
Automated systems for identifying red flags within financial agreements are changing how AML compliance is managed. Financial institutions are now able to leverage AI, specifically natural language processing and machine learning, to sift through complicated contracts and detect potentially problematic clauses or unusual patterns in transaction information. This capability allows for a more accurate detection of suspicious activity, while at the same time significantly reducing the need for time-consuming and resource-intensive manual checks. This ultimately leads to a smoother and more streamlined AML compliance process. However, it's crucial to remember that human expertise is still needed, particularly in areas where AI's ability to interpret nuanced risk factors is still limited. The nature of financial crimes is constantly changing, and the systems used to analyze contracts need to adapt to stay ahead, ensuring that AML measures remain effective.
The intricate language used in financial agreements often obscures potential red flags, especially those related to money laundering. Automated systems aim to decipher this complexity, but the ever-evolving nature of legal terminology means these systems require consistent updates to maintain their effectiveness. This highlights a challenge for the long-term utility of these tools.
Going beyond simple keyword searches, these advanced systems can evaluate the context around specific terms to uncover potential risks. For example, if a contract features unusual combinations of parties or transaction types, it might trigger an alert that suggests further investigation for money laundering.
The flow of funds between parties can be dissected by these automated systems, pinpointing inconsistencies or unusual patterns that might be overlooked during traditional manual reviews. This capability greatly strengthens the ability to detect intricate financial schemes often associated with money laundering.
Rather than relying on fixed rules or past experiences, these automated systems have the advantage of learning in real-time through continuous monitoring of transactions. This adaptability empowers them to counter emerging money laundering tactics as they evolve, which is vital as criminal methods are constantly updated.
When dealing with international contracts, automated systems can harmonize the interpretation of laws from various jurisdictions and highlight compliance concerns specific to each location. This function is essential for multinational companies operating across borders with differing legal frameworks.
By employing historical data, many automated systems can assign risk scores to specific contract clauses, which helps compliance teams prioritize their reviews. This helps optimize the use of resources and focus attention on the areas that pose the highest potential risks.
These systems can construct profiles of typical client or party behavior by analyzing past transactions. If future activity deviates from this established norm, it can indicate potential issues. These profiles help understand 'normal' behavior and flag deviations.
Some systems are built to integrate contract analysis with real-time transaction monitoring to improve overall compliance efforts. This collaboration means changes to contracts can trigger alarms if they correlate with irregular financial activity, linking events more efficiently.
While these systems learn from massive datasets, there is a danger that they may inadvertently perpetuate existing biases found in the data, leading to potentially unfair or discriminatory compliance decisions. To counteract this, new AI models are designed not only to spot bias within the training data but also to mitigate their impact on the outcome, which is crucial to building trust in these tools.
Beyond simply understanding legal terms, more sophisticated systems also employ sentiment analysis. This method allows them to interpret the underlying tone and intent in contractual language, potentially revealing hidden risks or potentially malicious intentions in financial agreements. This offers a more nuanced way of understanding risk.
The Role of AI in Enhancing Anti-Money Laundering Compliance for Contract Review - AI's Role in Continuous Monitoring of Contract Parties
AI is playing a growing role in the ongoing monitoring of parties involved in contracts, especially in the context of preventing money laundering. AI systems are capable of continuously examining contracts to assess the behavior and actions of the involved parties, which helps identify potential risks as they emerge. These systems leverage sophisticated machine learning and natural language processing techniques to spot anomalies in activities and flag transactions that could require a closer look. However, despite the powerful features of AI, it's critical that human experts are still involved. They play a vital part in understanding the more intricate risks and ensuring they are properly handled. The constantly changing nature of financial crimes continues to be a significant obstacle, even for the most advanced AI tools. This means maintaining a combined human and AI approach is still essential for achieving effective compliance and protecting against illicit financial activity.
AI is increasingly involved in continuously monitoring contract parties, particularly within the context of anti-money laundering (AML) efforts. It can handle contracts written in a variety of languages and dialects, making it a valuable asset for multinational businesses that navigate diverse legal frameworks. This expands the reach of global AML initiatives by ensuring contracts are understood and compliant with local regulations. Beyond recognizing established patterns of suspicious activity, AI, through machine learning, is also capable of identifying emerging and novel tactics used by criminals involved in money laundering. This adaptability is especially important as the methods used by these groups evolve rapidly, posing a challenge for traditional compliance practices.
These AI-driven tools go beyond conventional methods by automatically gathering and analyzing unstructured data, such as contracts, emails, and other textual sources, to provide a more thorough risk assessment process. This wider perspective empowers compliance teams to find unusual financial activities that might have otherwise gone unnoticed. AI enhances resource allocation through assigning risk scores to contract clauses based on historical compliance data. This data-driven prioritization helps compliance experts concentrate on the most critical risks. Continuous monitoring through AI generates real-time alerts when changes occur within a contract, particularly within sections dealing with transactions. This allows for a quick response to potentially suspicious activity rather than relying on periodic review processes.
These advanced systems can also make complex contract relationships easier to understand for compliance teams through visualizations. These visual representations bridge the communication gap between the technical aspects of the analysis and actionable insights for all stakeholders. By combining data from diverse sources, AI creates thorough behavioral profiles of those involved in contracts. These profiles establish a baseline of what's considered "normal" behavior, highlighting any unusual activity that might indicate money laundering.
However, AI's dependence on historical data for training introduces a potential challenge—the risk of bias. There's ongoing research to develop algorithms capable of identifying and minimizing these biases to ensure fair and equitable compliance practices. The speed at which AI analyzes contracts and identifies potential issues is significant, decreasing the time it takes to conduct compliance checks from weeks to a matter of hours. This improved responsiveness is crucial for organizations to react quickly to possible money laundering activities. New systems are also integrating sentiment analysis into the process. This deeper understanding of the intent behind the language could revolutionize how compliance teams evaluate contract language, ultimately leading to more accurate identification of potential issues.
While AI shows a lot of promise in bolstering AML compliance, it's essential to acknowledge the evolving nature of legal terminology and criminal tactics. AI models will require consistent updates and improvements to remain effective in this environment. Ultimately, the integration of these new tools alongside human expertise will be crucial for sustained success in mitigating financial crime.
The Role of AI in Enhancing Anti-Money Laundering Compliance for Contract Review - Challenges and Limitations of AI in AML Contract Review
Implementing AI in AML contract review, while promising, comes with inherent challenges and limitations. AI systems, powered by machine learning and natural language processing, can certainly improve efficiency and identify patterns within contracts. However, their dependence on existing data for training can lead to biases being unintentionally reinforced, potentially skewing compliance decisions. The ever-changing landscape of financial crimes and the constant evolution of legal language require ongoing adjustments to AI models to remain effective. This need for continuous updates can be difficult to manage. Moreover, the complex nature of risk assessment often requires a human element. AI might miss subtle risks that a human expert would catch, making a combined human-AI approach necessary for achieving truly robust AML compliance. The reliance on AI should be balanced with the understanding that its capabilities are not limitless, and human expertise remains vital in many aspects of compliance.
While AI holds great promise in bolstering AML compliance within contract review, there are still a number of hurdles to overcome. AI systems, despite being trained on huge datasets, often face challenges in separating genuine insights from overwhelming amounts of data. This can lead to critical information getting lost in the noise. The intricate and ever-changing language of contracts, coupled with cultural variations in legal interpretations, makes it difficult for AI to stay truly effective. Keeping these systems updated with the latest legal phrasing is a constant battle.
Another concern is the potential for bias in AI models. Since they often learn from past data, they can inadvertently absorb and amplify existing biases. This could result in unfair targeting of specific demographics during AML investigations. It's a worry that these systems might inadvertently promote inequitable practices if not carefully managed.
There's a risk of organizations becoming over-dependent on AI, thinking it's a magic bullet for AML. This can lead to blind spots as AI currently lacks the ability to fully comprehend the nuances of human behavior and the subtleties of fraudulent schemes. A truly effective strategy requires a sensible balance of human and AI capabilities.
Getting AI systems to smoothly integrate into existing compliance workflows can be challenging. The goal isn't to replace humans but to augment their efforts, which calls for careful planning to ensure compliance with all regulations. Despite being designed for continuous monitoring, AI may not be agile enough to keep pace with the constantly evolving tactics used in financial crimes. There could be lapses in real-time surveillance that lead to missed opportunities for detection.
Furthermore, the inner workings of many AI algorithms are difficult to understand, which can be a roadblock for compliance teams trying to use the AI's recommendations. They may struggle to understand the rationale behind the AI's suggestions, leading to confusion and possibly misinterpretations. The AML rules and regulations differ greatly between countries, and it's a challenge for AI systems to adapt seamlessly to this variety. This could cause trouble for multinational businesses navigating different legal landscapes.
Over time, AI models can lose their accuracy ("model drift") due to shifts in the type of data they analyze. This affects their ability to reliably pinpoint money laundering activities. Regulators are paying increased attention to how AI is being used in compliance, particularly concerning issues like fairness and accountability. This means AI developers and users will need to continuously adjust and explain their approaches.
These challenges highlight the need for a nuanced and cautious approach to using AI for AML contract review. It's a domain where the benefits of the technology need to be weighed against these potential limitations to ensure responsible development and use.
eDiscovery, financial audits, and regulatory compliance - streamline your processes and boost accuracy with AI-powered financial analysis (Get started for free)
More Posts from financialauditexpert.com: