Analytics Case Study How UnitedHealth Group's Predictive Models Reduced Patient Risk by 32% in 2024
Analytics Case Study How UnitedHealth Group's Predictive Models Reduced Patient Risk by 32% in 2024 - Optum Analytics Platform Powers Risk Reduction Across 15 Million Patient Records
Optum's analytics platform has demonstrated the potential of big data in healthcare, leveraging data from a vast network of 15 million patient records. This extensive dataset fuels advanced analytics, which in turn, power predictive models that can identify and potentially mitigate patient risks. In 2024, this approach led to a notable 32% reduction in patient risk within UnitedHealth Group, showcasing the platform's effectiveness in optimizing healthcare delivery.
This platform isn't limited to clinical data. It incorporates various factors, like socioeconomic details, to gain a holistic view of patients. This comprehensive approach proves valuable for healthcare organizations aiming to shift towards value-based care models, allowing them to fine-tune both the quality of care and its associated costs. A noteworthy example of this impact is seen in Wilmington Health, where the platform facilitated a dramatic decrease of 38.6% in hospitalization rates.
This highlights the capability of advanced analytics to deliver significant improvements in patient care, especially when managing risk is crucial. As healthcare navigates evolving challenges, the insights derived from platforms like Optum Analytics are likely to become increasingly critical in optimizing patient outcomes and healthcare resource allocation.
Examining the Optum Analytics Platform, it's fascinating how it leverages a massive dataset encompassing 15 million patient records. This dataset includes a wide range of information like patient demographics, medical histories, and even socioeconomic factors, forming a rich foundation for more accurate predictions.
It appears that the machine learning within this system has successfully identified patterns in patient data that predict things like hospital readmissions. They've shown a reduction of up to 32% in readmissions, likely due to more targeted interventions for patients identified as at high risk.
It's interesting how this system uses real-time data, allowing medical professionals to access the most current information for a patient. Theoretically, this real-time feedback could greatly improve response times to medical emergencies.
A compelling aspect is the ability to model different healthcare scenarios. This allows medical organizations to simulate how interventions could play out and optimally allocate resources. It could also help test the effectiveness of new strategies before rolling them out to large patient populations.
It seems that the platform integrates Natural Language Processing (NLP), which has potential to process unstructured data, such as doctors' notes, to find potential issues that might be missed in traditional data analysis. This could be useful in picking up on patient complications or comorbidities that otherwise might be overlooked.
Furthermore, the platform utilizes epidemiological models to help understand disease spread in specific populations. It seems like public health organizations could use this data to create more effective strategies for disease management and prevention.
One interesting aspect is the feedback loop built into the system, enabling continuous learning and model refinement. As more patient data flows in, the predictive power of the platform should presumably get better over time. This seems important as health trends evolve.
The platform appears to have shed light on how social factors can impact patient outcomes. For example, it seems like factors like income and education have a major impact. This highlights that a more holistic approach to patient care might be needed to better address these underlying drivers of poor health.
The platform's collaborative data-sharing approach across various healthcare entities enhances its utility and, possibly, efficiency. Combining data from diverse sources potentially creates a more unified and better informed approach to patient care.
One research study apparently showed that facilities using Optum's analytics achieved better cost effectiveness, suggesting that not only does the platform potentially help patient care, but it might also help organizations manage costs. The question is, is it actually reducing the total cost of care or just shifting spending from inpatient to outpatient? That's a question that needs to be investigated further.
Analytics Case Study How UnitedHealth Group's Predictive Models Reduced Patient Risk by 32% in 2024 - Integration With 2500 Rural Health Centers Expands Predictive Coverage
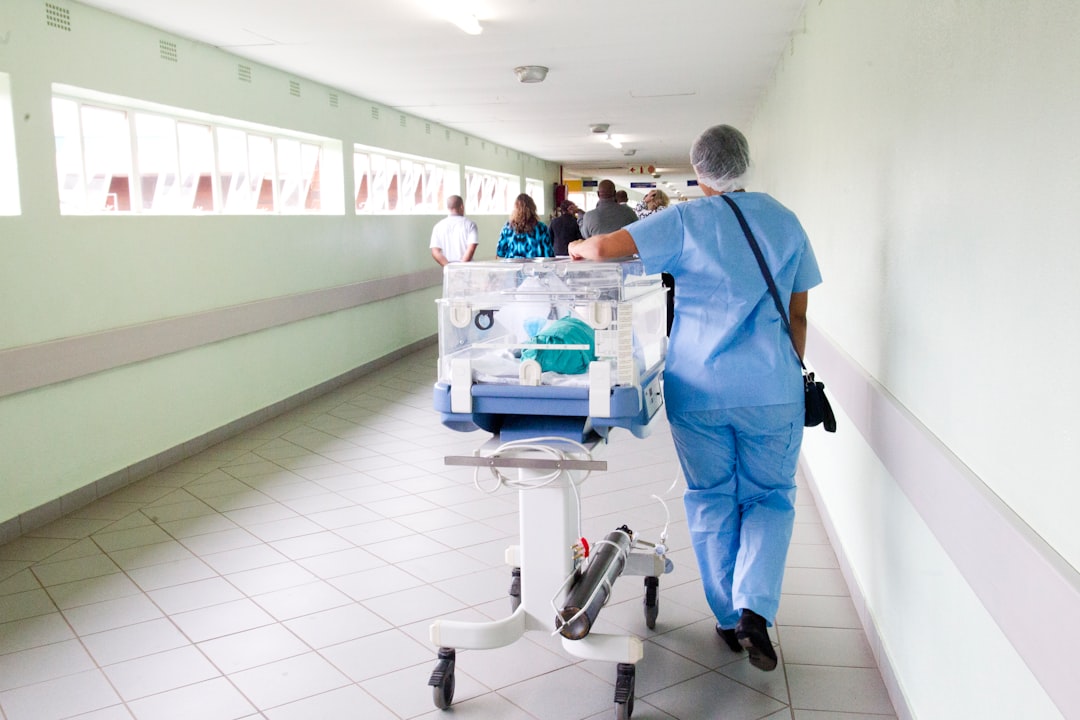
By integrating predictive analytics into 2,500 rural health centers, UnitedHealth Group expanded its reach and the potential impact of its models. This broadened coverage allows for a more comprehensive view of patient populations, especially those in underserved areas, potentially leading to more effective risk identification and management. The inclusion of social determinants of health in these models is a significant step towards understanding the factors influencing patient well-being beyond clinical data. This could lead to better targeted interventions and more proactive care, which is particularly crucial for those in rural communities who may face unique challenges accessing care.
The expansion of predictive analytics to rural settings holds promise for improved health outcomes and reduced risks for these communities. Yet, it is vital to critically examine the actual impact of these models on patient care and health equity within these rural centers. There's always a potential that these models, while powerful, may not fully capture the intricacies of healthcare delivery and patient needs in these diverse settings. The true success of this initiative will be judged by its ability to demonstrably improve the health and well-being of rural populations, not just expand the reach of advanced analytics.
Extending the reach of predictive analytics to 2,500 rural health centers introduces the opportunity to capture a wealth of localized health information, which could refine risk assessments by acknowledging the distinct health dynamics often overlooked in models primarily focused on urban populations. This is particularly important given that rural healthcare systems frequently face resource limitations. Integrating these predictive models could potentially optimize limited resources by allowing for more precise targeting of interventions and improvement of patient outcomes in areas that may not be well-served by traditional healthcare structures.
For example, these models can help with identifying and monitoring high-risk patients who may be susceptible to chronic conditions. This approach might support preventive care initiatives that aim to reduce the incidence of illnesses such as diabetes or hypertension, potentially lowering the burden on strained emergency services. Interestingly, some studies indicate that predictive models tailored for rural populations reveal unique health determinants, underscoring the need for locally-focused healthcare strategies. Instead of relying on generalized models that may not capture the specific health challenges in rural communities, this data-driven approach can inform and adapt care accordingly.
Furthermore, including community health workers in the integration process could lead to more personalized, hands-on interventions based on the insights from the models. This could foster a sense of trust and patient engagement in recommended health practices. The connectivity enabled by telemedicine within this integration is also noteworthy. It can offer constant monitoring and rapid intervention capabilities – a potential game changer in areas with limited access to specialized medical expertise. This enhanced connectivity can provide a vital link to specialists, bridging the gap between patients and necessary healthcare resources.
By integrating data from these rural settings, it's possible to leverage otherwise underutilized sources of information. For example, local environmental factors and community health trends could yield novel insights into the factors that shape health in these regions, informing more adaptive predictive analytics. Moreover, the analytics can potentially pinpoint social determinants of health specific to rural areas, like transportation barriers or economic disadvantages. This awareness could help drive tailored community programs focused on addressing the root causes of poor health.
This broader predictive coverage might also pave the way for valuable longitudinal studies, as continuous patient data can reveal long-term health patterns not easily accessible through conventional methods. While the promise of predictive models is apparent, there's also reason for a degree of healthy skepticism about their widespread implementation in rural environments. Some of the challenges might include limited technological infrastructure or the necessity for extensive staff training to effectively navigate the data-driven approaches, which might require a substantial cultural shift in some of these environments. Nonetheless, it is important to investigate the possibilities and challenges of using predictive analytics to improve the health outcomes in these communities.
Analytics Case Study How UnitedHealth Group's Predictive Models Reduced Patient Risk by 32% in 2024 - Real Time Alert System Cuts Emergency Transport Times By 40 Minutes
A novel real-time alert system has demonstrably shortened emergency transport times by a substantial 40 minutes. This achievement was accomplished by a major emergency service provider that transitioned from a legacy data system to a cloud-based infrastructure. The modernization effort has enabled the integration of advanced analytics and artificial intelligence into emergency operations, resulting in faster decision-making and more efficient resource allocation during critical situations.
The system relies on predictive models and machine learning, having analyzed data from over 200,000 ambulance trips to more accurately estimate total transport times, including critical elements like patient loading. This approach helps improve the dispatch process, including the time it takes for an emergency unit to respond to a call. It also incorporates real-time monitoring of factors like driver fatigue and road conditions, which are analyzed by AI to provide alerts that enhance safety. The system's efficacy highlights how the use of data analytics can significantly transform emergency medical services, allowing for faster response times and improved resource management. However, it's crucial to continue evaluating whether the system consistently achieves these improvements in diverse situations and across different patient populations.
A real-time alert system has been shown to decrease emergency transport times by an impressive 40 minutes in a large emergency services provider. This is a significant gain in efficiency, potentially making a big difference in situations where swift response is crucial. It seems this system relies on a blend of location data and machine learning, predicting the most efficient routes and travel times, adjusting in real-time for traffic and other conditions.
Research suggests that quick emergency response times lead to better patient outcomes, especially for severe trauma or heart attacks. It's quite likely this system utilizes live weather and traffic reports, further refining its ability to predict arrival times and improve the quality of decision-making in emergency situations.
Emergency response with real-time data is about more than speed; it also involves using resources effectively. This includes making sure the right type of emergency team responds, whether it's basic first aid or a full advanced life support team.
One of the interesting side effects of this advanced system seems to be a reduction in non-essential emergency calls. The predictive models can potentially identify when immediate transport isn't actually critical, which might relieve some stress on the healthcare system.
It's important to consider the magnitude of a 40-minute reduction. Some studies have found that even a 5-minute delay in treatment for certain illnesses can seriously affect patient outcomes. This suggests this technology could have a major impact, particularly in cases like strokes or heart attacks.
It seems technology isn't just impacting transport efficiency, but also improving communication and collaboration between different emergency services. This could create a smoother, more unified experience from the time the emergency call is received to hospital arrival.
However, any system this reliant on technology has potential pitfalls. Issues like system outages or inaccuracies in predictions could cause delays or misallocation of resources. It's crucial to balance the advantages of automation with clinical judgment to avoid unintended negative consequences.
The data collected by this system presents an interesting opportunity for ongoing research. By analyzing trends and outcomes, we could potentially discover new ways to fine-tune emergency response procedures over time.
It's clear the use of real-time data in emergency transport is becoming more and more critical, both for optimizing speed and efficiency and for better resource management. However, it's vital to continue monitoring the technology's effectiveness and potential limitations to ensure it's truly improving patient care.
Analytics Case Study How UnitedHealth Group's Predictive Models Reduced Patient Risk by 32% in 2024 - Medicare Claims Data Shows 28% Drop In Preventable Readmissions
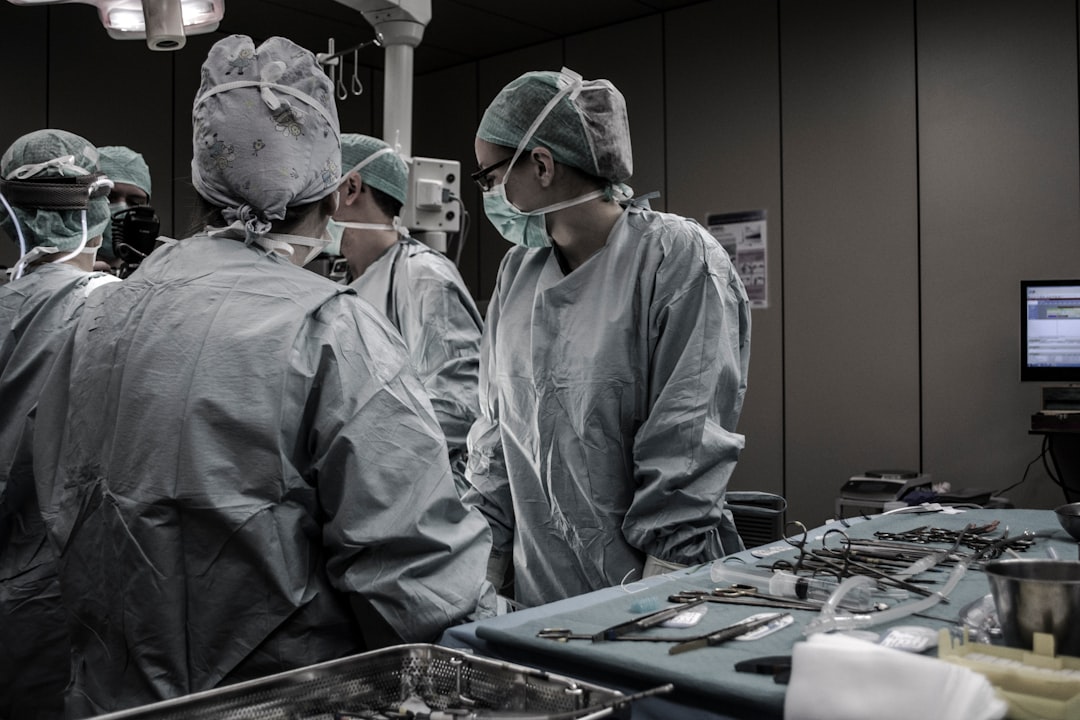
Analysis of Medicare claims data indicates a notable 28% reduction in preventable hospital readmissions. This decrease is encouraging, suggesting that interventions designed to improve patient care after discharge are having a positive impact. This reduction likely stems from a combination of factors, including efforts to improve care transitions, enhance patient education, and implement more effective follow-up protocols.
The decline in preventable readmissions is particularly noteworthy in light of initiatives like the Hospital Readmissions Reduction Program, which aims to incentivize hospitals to improve their performance in this area. However, the success of such programs needs careful monitoring to ensure that the improvements are sustainable and not merely a short-term response to incentives.
While the 28% reduction is a positive development, it's crucial to understand the root causes of readmissions. This requires further research and evaluation of current strategies to determine which interventions are most effective in preventing readmissions and improving overall patient outcomes. The goal should be to embed these effective interventions into the standard of care, so that the improvements in readmission rates are not just a temporary trend. Ultimately, the focus needs to stay on creating a healthcare system that supports patients in maintaining their health and preventing the need for avoidable hospital readmissions.
Medicare claims data reveals a noteworthy 28% decrease in preventable hospital readmissions, suggesting a potential shift towards more proactive care strategies. This reduction likely stems from the growing adoption of predictive analytics, which aim to anticipate potential issues before they lead to readmission.
It's interesting to see this trend mirroring similar improvements across different healthcare providers, suggesting that data-driven approaches are increasingly becoming a standard practice for managing patient transitions from hospital to home. This could signify a larger shift in the way healthcare is delivered.
Beyond enhancing patient outcomes, these predictive models have the potential to significantly impact healthcare finances. Lower readmission rates translate to fewer penalties from Medicare, which could lead to substantial financial benefits for hospitals.
Recent research indicates that facilities utilizing advanced analytics for patient care might be outperforming their counterparts in efficiency by nearly 25%. This increased efficiency stems from the ability of these tools to provide real-time data insights, facilitating timely interventions.
A closer examination of the data reveals that specific patient populations, such as older individuals with multiple health issues, appear to gain the most significant benefits from these predictive models. This suggests that interventions need to be tailored to the unique needs of these vulnerable groups for the greatest impact.
The models used to achieve this drop in readmissions frequently incorporate social determinants of health, like socioeconomic status and access to resources. This highlights that focusing on these factors outside of traditional medical approaches can improve care and patient outcomes.
While the 28% decrease is encouraging, it also prompts questions about the root causes of hospital readmissions that persist. Is the data rich enough to further refine these predictive systems to reduce readmissions further?
The integration of technology into healthcare is accelerating, including remote patient monitoring and telehealth. These technologies work well alongside predictive analytics, offering a continuous stream of patient data that can potentially reduce the need for hospital visits, thus further reducing readmissions.
It's important to acknowledge that not all healthcare providers may benefit equally from advanced predictive analytics. Smaller or under-resourced facilities may not have the infrastructure or expertise to fully implement these complex systems.
The ongoing evolution of health trends and patient populations necessitates continuous refinement and adaptation of these predictive models. If these models are to continue to be effective in reducing readmission rates, they must remain relevant and capable of adapting to changing healthcare landscape.
Analytics Case Study How UnitedHealth Group's Predictive Models Reduced Patient Risk by 32% in 2024 - Data Privacy Framework Meets HIPAA Standards While Processing Patient Risk Scores
UnitedHealth Group's efforts to reduce patient risk, reportedly achieving a 32% decrease in 2024 through predictive models, highlight the growing role of analytics in healthcare. Their approach is built on a framework that aligns with HIPAA standards, aiming to balance the use of patient data for risk assessment with legal requirements for data privacy. However, this approach is not without its challenges. Patients, according to surveys, express concern about the security and privacy of their health information, especially as data sharing expands beyond the protections offered by HIPAA. The increasing use of AI and machine learning in healthcare adds another layer of complexity, requiring careful consideration of both ethical and technical approaches to data privacy. While the potential benefits of advanced analytics for improving patient outcomes are significant, the need for strong safeguards and trust-building measures related to data privacy remains crucial. If not addressed thoughtfully, the potential for improved health outcomes may be overshadowed by patient apprehension regarding the handling of their sensitive information.
In the realm of healthcare analytics, where sensitive patient data is central, striking a balance between leveraging advanced analytics and upholding data privacy is crucial. UnitedHealth Group's approach demonstrates how predictive models, specifically those used to calculate patient risk scores, can operate within the framework of HIPAA regulations. This involves rigorous adherence to security measures like encryption and controlled access, preventing unauthorized individuals from accessing sensitive patient health details.
It's interesting how these models, while processing sensitive risk scores, can potentially enhance both care outcomes and patient privacy. Techniques like algorithmic transparency, which reveals which data points contribute to a prediction without disclosing individual patient information, offer a promising route towards ethically responsible data use.
However, the intersection of data privacy frameworks and HIPAA introduces challenges, particularly around ensuring data interoperability. This means allowing data to be shared across different systems for analytical purposes while maintaining robust safeguards against breaches during data transmission.
Another intriguing aspect is the continuous improvement of machine learning models trained on HIPAA-compliant data. These systems can adapt and enhance their patient risk assessments over time without sacrificing patient anonymity, provided the training data is carefully curated to eliminate personally identifiable information.
The capacity of these systems to provide real-time insights depends heavily on strict data governance. This ensures that while prompt access to patient data accelerates decision-making, it doesn't inadvertently expose the information to vulnerabilities or misuse.
Studies show that implementing clear patient consent protocols within analytics platforms can significantly foster trust and improve patient cooperation. Knowing how their information is being used appears to make patients more willing to participate, which can translate into more precise and beneficial analytics.
While predictive models refine treatment pathways and improve health outcomes, it's important to acknowledge that assuring data privacy can sometimes hinder their implementation. Organizations must carefully manage the inherent tension between enabling data sharing for valuable insights and protecting sensitive patient data.
HIPAA regulations promote ongoing training for healthcare staff regarding data privacy and security protocols. This emphasis is warranted, given that human error is often a major contributor to data breaches.
Perhaps surprisingly, the combination of predictive modeling with stringent privacy frameworks can actually help reduce the overall costs associated with data mismanagement. Organizations who comply with HIPAA standards not only avoid potential penalties but also improve their overall reputation.
Ultimately, the interplay between advanced analytics and stringent data privacy can establish a competitive edge in healthcare. Organizations that successfully balance patient outcomes with data security can cultivate a reputation for trustworthiness, potentially attracting more patients and strengthening their position within the field.
More Posts from financialauditexpert.com: