eDiscovery, financial audits, and regulatory compliance - streamline your processes and boost accuracy with AI-powered financial analysis (Get started for free)
AI-Powered ISO 9001 Audit Analytics 7 Key Efficiency Gains for Financial Auditors in 2025
AI-Powered ISO 9001 Audit Analytics 7 Key Efficiency Gains for Financial Auditors in 2025 - Natural Language Processing Spots Transaction Anomalies 85% Faster Than Manual Reviews
Natural Language Processing (NLP) is revolutionizing how financial institutions spot suspicious transactions, significantly outpacing traditional manual reviews. This technology can achieve an 85% speed increase, which is crucial given the constantly rising volume and intricacy of financial dealings in the rapidly evolving world of financial technology. As the use of AI and machine learning expands, companies can analyze massive datasets with unprecedented speed, ultimately boosting their fraud prevention efforts. Furthermore, the persistent need to keep customer information up-to-date makes NLP a valuable tool in streamlining Know Your Customer (KYC) procedures, firmly establishing its importance in contemporary financial auditing. While this efficiency is promising, it's important to consider the implications of relying on complex algorithms and the potential need for human oversight in sensitive financial contexts.
In the realm of financial auditing, the application of Natural Language Processing (NLP) is showing immense promise in accelerating the detection of unusual financial activities. NLP techniques excel at sifting through massive volumes of transaction data, uncovering patterns and anomalies that might go unnoticed during standard manual reviews. This advantage arises from NLP's ability to examine various aspects of a transaction simultaneously, recognizing discrepancies in real-time. Human auditors, on the other hand, are generally constrained to scrutinizing individual transactions one at a time, hindering their ability to spot subtle connections or deviations.
NLP's effectiveness also benefits from the ability to be trained on historical transaction data. These learning processes allow for the development of behavioral profiles for legitimate financial actions. Once these norms are established, any deviations that surpass predefined thresholds can be flagged as potentially anomalous. In essence, NLP helps establish a baseline of what's normal, thus enabling more accurate identification of abnormalities.
Interestingly, NLP can potentially enhance the reliability of audit outcomes, mitigating the possibility of human bias or errors that sometimes creep into manual review processes. This capacity for impartial evaluation is an attractive feature. Moreover, the ability of NLP to continually update and refine its understanding of financial data based on new information is a significant strength. As financial markets evolve and patterns shift, the algorithms underpinning NLP systems can adjust accordingly, maintaining their efficacy.
Human auditors, as well as the human review process itself, are subject to fatigue and limitations in their attention span. In contrast, NLP algorithms can analyze data 24/7 without tiring. This consistent, unrelenting scrutiny is especially valuable for detecting fraudulent transactions or other illicit financial maneuvers.
Furthermore, NLP has shown promise in revealing unusual patterns or activities that human reviewers may have missed. This highlights the potential for NLP not just to augment, but to potentially improve upon the existing capabilities of human-based audits.
The reach of NLP extends beyond just recognizing numerical anomalies. It can also analyze the text-based components of financial communications. By interpreting the language and context, NLP can identify irregularities that might not be apparent through numeric assessments alone. This capability offers an extra layer of analysis.
Beyond efficiency gains, the application of NLP in audits carries potential for reducing operating costs. By entrusting routine transaction checks to NLP, human professionals can concentrate on tasks that demand more sophisticated analytical and reasoning skills. This re-allocation of human resources can potentially optimize workflow and streamline overall audit processes.
However, the ideal future of NLP in financial auditing likely involves a hybrid model. A collaborative approach, wherein human knowledge and machine learning synergize, could lead to a more holistic audit. It would be a future where quantitative and qualitative data are seamlessly intertwined to achieve a comprehensive and rigorous assessment of financial records. It's an evolving area and further research is needed, but this area has shown potential for substantial improvements in the speed, effectiveness, and cost-efficiency of financial auditing.
AI-Powered ISO 9001 Audit Analytics 7 Key Efficiency Gains for Financial Auditors in 2025 - Smart Pattern Recognition Maps Risk Areas Across 50,000 Audit Documents Daily
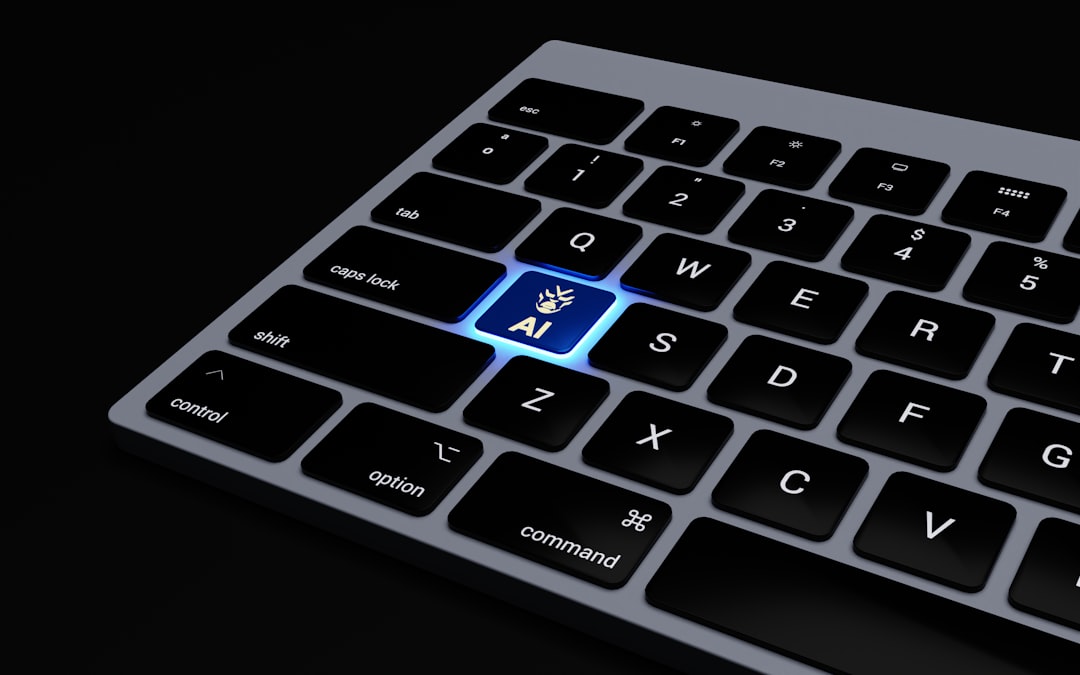
AI-driven pattern recognition is transforming how auditors analyze vast quantities of audit data to understand and manage risk. These systems can process 50,000 audit documents daily, swiftly identifying patterns and unusual trends that might signal potential issues. This rapid analysis allows auditors to develop more accurate and timely risk assessments, enabling proactive mitigation of emerging threats. The capability to predict risks offers a significant advantage in today's dynamic financial landscape.
However, while promising, the growing reliance on complex AI algorithms raises questions about maintaining human oversight and assuring the reliability of audit findings. The increasing integration of AI tools into the audit process highlights a broader trend towards leveraging technology to increase efficiency and accuracy in financial auditing. The field is evolving, and the development of these tools must balance innovation with considerations for data integrity and human intervention where needed.
Imagine a system capable of plowing through 50,000 audit documents each day – that's the kind of data volume we're talking about with smart pattern recognition. It's a fascinating development, as humans simply can't sift through that much information in a reasonable time frame.
These systems are designed to build a dynamic risk map in real time, essentially turning traditional audits into proactive risk management initiatives. The auditor can immediately identify the areas of highest risk and direct their attention accordingly, which is much more efficient than a traditional, more linear process.
One of the things that intrigues me most is the ability of these systems to spot anomalies that might escape human eyes. By employing complex algorithms, they can detect subtle deviations that suggest potential irregularities or even fraud. This enhanced detection capability is pretty significant.
Furthermore, these AI systems are trained to understand what "normal" looks like in terms of financial transactions. They learn from past data to develop baseline patterns and then flag anything that falls outside of that norm. It's like they develop a kind of intuition about the usual flow of transactions.
Another aspect that's interesting is their ability to analyze data from numerous perspectives simultaneously. This multi-faceted approach allows them to uncover relationships and patterns that might remain hidden during a more limited, single-angle analysis. It's as if we gain a 360-degree view of the data.
The scalability of these technologies is quite compelling. They can be readily adapted to handle increasing volumes of data without needing a huge increase in resources. This is a huge advantage in a world where data is rapidly growing.
Of course, implementing these systems can lead to cost savings. They automate the review of routine data, freeing up auditors for more advanced analytical tasks. This shift in focus is pretty significant.
Interestingly, these systems help to "clean up" the data during the early stages of analysis. They can standardize data formats and improve data quality. This process reduces the "noise" within the data, making the patterns more discernible.
These tools often have intuitive interfaces, allowing auditors to see the results of the analysis in an accessible way. This visualization aspect makes it easier for auditors to communicate findings and make well-informed decisions.
Finally, these systems provide a detailed audit trail and complete logs. It's all about maintaining transparency and accountability in a highly regulated field like finance.
I still have some questions about the reliability and potential biases that might be inherent in the algorithms themselves. The black-box nature of certain AI models does lead to some concerns. However, it's undeniable that these smart pattern recognition tools offer a considerable advantage in the field of financial auditing. It's definitely an area that deserves further exploration and deeper investigation.
AI-Powered ISO 9001 Audit Analytics 7 Key Efficiency Gains for Financial Auditors in 2025 - Automated Evidence Collection Reduces Manual Data Entry By 6 Hours Per Audit
Automating the process of gathering evidence is dramatically changing how audits are performed, potentially saving up to six hours of manual data entry per audit. This time savings allows auditors to focus on higher-level tasks, such as in-depth analysis and strategic planning, ultimately boosting the overall quality of the audit. AI's role in automating routine aspects, such as data entry and sample selection, simplifies the workflow and reduces human errors, which in turn can inspire more confidence in the results. It's crucial, however, to realize that despite the clear benefits of automation, human oversight remains a critical component to address the multifaceted nature of auditing and any potential biases that AI-powered systems might introduce. As the financial auditing field increasingly embraces AI-driven tools, we're likely to see a growing emphasis on data-driven approaches to audit practice, reshaping the future of the industry.
It's intriguing how the automation of evidence gathering during audits can potentially save up to six hours of manual data input per audit. This shift away from tedious, repetitive data entry allows auditors to delve deeper into more intricate aspects of their work – like identifying trends and uncovering insights hidden within the data. This reallocation of time towards higher-level analysis is a significant aspect of this developing field.
Interestingly, by taking the human element out of certain repetitive data tasks, there's a reduced chance of human error impacting the quality of the data. This in turn suggests a possible increase in the overall accuracy of audit outcomes, which is a valuable pursuit for anyone involved in ensuring the integrity of financial records. While the technology driving this automation is still relatively new, the potential impact on accuracy could be notable.
One of the advantages I find interesting about automated data collection is the flexibility it provides when audit workloads increase. Unlike human auditors who might be impacted by increasing workload and experience burnout, automated systems can smoothly handle larger datasets without needing a proportional increase in personnel. This scalability characteristic is becoming increasingly relevant in an era of constantly growing volumes of financial data.
Furthermore, automated systems often have the ability to seamlessly integrate data from numerous sources. This allows for audits to be conducted using the latest available information, which is especially critical in rapidly evolving financial environments. While this integration is positive, there's always the possibility of needing to understand the data flow more comprehensively so that its accuracy can be further assured.
Though often discussed from the perspective of its role in data entry, evidence collection automation also plays a role in how organizations manage compliance. Streamlining the tracking of compliance data can benefit auditors by providing a well-organized overview of relevant documents and evidence. This organization of information is especially important as financial regulations frequently evolve, and keeping pace with the changes often creates a heavy administrative burden.
This automation can also facilitate a higher degree of collaboration. When everyone involved in the audit process uses a standardized and automatically-updated set of data, it creates a smoother flow of information and insights. However, it's important to ensure the automation doesn't inadvertently create new barriers to communication or that it introduces new risks to privacy or confidentiality.
Another intriguing application of automation is the potential to integrate and analyze historical data. Auditors can benefit from understanding trends and anomalies over time to contextualize current audits, perhaps allowing for more effective predictive analysis of future financial conditions. It will be interesting to see how this capability develops further and how the information it generates is best used in audit practices.
Besides improving workflow efficiency, there are also economic benefits to consider. Automated systems can potentially reduce the overall operating costs of the audit process by reducing labor-intensive data entry. Re-allocating funds from basic data handling to more strategic and nuanced aspects of auditing could be beneficial for organizations in this industry.
Automation can also help create a greater focus on high-priority risk assessment and mitigation strategies. When auditors are freed from tedious data entry, they can spend more time assessing the potential vulnerabilities and developing preventative solutions. This increased concentration on risk could lead to more proactive and effective audit strategies.
And finally, through automation, there is a chance to establish greater consistency in the application of audit procedures across different projects. Often, maintaining consistency across a team of human auditors can be a challenge. While automated systems don't eliminate human judgment completely, it can at least standardize many of the more objective elements of the auditing process, improving the rigor and reliability of every audit. However, we should ensure any bias inherent in automated systems is accounted for, and the systems are not used in a way that undermines fairness.
The ongoing development and refinement of these automated tools is certainly worth watching, and this specific capability in automated evidence collection provides a good illustration of the ongoing transformation occurring in the auditing field.
AI-Powered ISO 9001 Audit Analytics 7 Key Efficiency Gains for Financial Auditors in 2025 - Real-Time Quality Monitoring Flags Non-Compliance Issues Within 30 Seconds
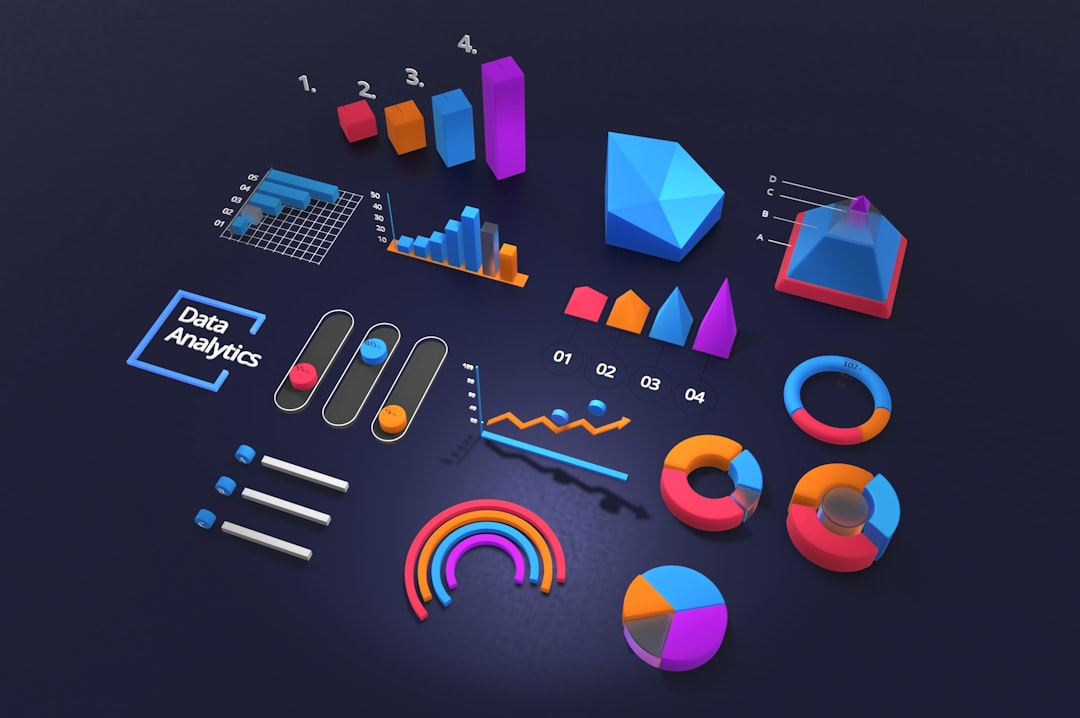
Real-time quality monitoring within the context of financial auditing is increasingly important. It can pinpoint non-compliance issues in a remarkably short time frame—within 30 seconds. This quick identification allows auditors to address problems immediately, potentially minimizing larger future issues. AI is significantly improving the speed and thoroughness of ISO 9001 audits. This includes not only faster compliance assessments but also better process streamlining. With AI, auditors can potentially reduce the risk of oversight errors and generate more dependable risk assessments. It's important to consider how conventional audit approaches will need to adapt to this evolving environment. However, in a field as complex as finance, the importance of human auditors and their judgment cannot be overstated, particularly given the potential biases that could be inherent in automated systems. The future of auditing likely involves a careful balance between advanced technology and the valuable human expertise that underpins trust and transparency in financial matters.
The ability to pinpoint compliance issues within a mere 30 seconds using real-time quality monitoring is a significant change from traditional audit practices, which can sometimes take days to surface similar problems. This speed offers a new level of responsiveness to potential violations, potentially leading to quicker resolutions and a more agile audit process. It also suggests a reduction in the need for constant human oversight, which is intriguing. Instead of relying on scheduled reviews, audits become more dynamic and potentially more reliable, as issues are identified immediately, minimizing the possibility of missing critical deviations from regulatory standards.
This capability of real-time monitoring leads to more adaptable audit strategies. As compliance flags appear, auditors can quickly re-focus their attention to the areas showing heightened risk, rather than strictly following a pre-determined audit plan. This flexibility seems beneficial, but one needs to be mindful of the possibility that it could lead to an over-reliance on flagged issues and a disregard for less urgent, but still important, parts of the audit.
Another fascinating feature of real-time quality monitoring is the ability to simultaneously monitor numerous data streams. This holistic view across different compliance metrics can uncover subtle connections and trends that might not be evident during a more sequential review process. This cross-referencing of information could improve insights, but we must remain cautious about the potential for introducing errors in interpreting the large volume of data collected.
Moreover, the ongoing nature of real-time monitoring allows for a detailed historical record of compliance issues. This continuous data accumulation aids in trend analysis, which can be useful for building more proactive risk management strategies. The idea of predicting potential issues based on historical data is quite promising, but it is important to verify that any trends observed are accurate and not influenced by biases within the system.
The audit trail generated by these automated systems is another critical aspect. The transparent and tamper-proof nature of this digital record contributes to the integrity of the audit process and the trust stakeholders have in its outcome. The existence of a strong audit trail can help promote confidence in the audit process but only if the system itself is inherently robust and reliable.
Furthermore, the swift identification of issues through real-time monitoring allows organizations to better allocate their resources. Auditors can shift their efforts from reacting to issues to building stronger preventative measures, leading to potentially improved audit outcomes. While this shift in focus may be advantageous, we need to ensure that the rapid pace of addressing flagged issues doesn't lead to overlooking potential root causes or neglecting essential parts of the audit process.
The sophistication of these systems allows them to learn from past data and improve their accuracy over time, potentially reducing the occurrence of false positives. These false alarms can be a distraction for human auditors, and reducing them could help focus their efforts on truly significant compliance matters. Though potentially beneficial, there's always a need for human oversight to avoid blind reliance on algorithms and to ensure these systems are operating as intended.
The integration capabilities of real-time monitoring also stand out. It can interact with other AI-driven tools, such as NLP and intelligent pattern recognition, offering a multifaceted and potentially more comprehensive approach to audit and compliance activities. While this integration can enhance overall efficacy, we must also be aware of the complexities of maintaining the coordination between these various tools.
Finally, real-time quality monitoring provides scalable solutions as organizations grow. As the quantity of data to be analyzed increases, the technology can adapt to the increased workload without a major increase in resources. It will be fascinating to see how these systems continue to evolve to manage the ever-increasing complexity of audits in the future. However, concerns about the potential for system errors or biases in managing such large datasets need to be addressed.
Overall, real-time monitoring for quality control and compliance promises exciting developments for the audit process. While many benefits are apparent, maintaining a healthy balance between automation and human oversight will be essential to realizing the full potential of these advancements and ensuring that they do not lead to unforeseen complications in the complex world of finance.
AI-Powered ISO 9001 Audit Analytics 7 Key Efficiency Gains for Financial Auditors in 2025 - Predictive Analytics Forecasts Control Weaknesses 3 Months Before They Occur
AI-powered predictive analytics is transforming how financial auditors anticipate and manage risks. By analyzing extensive data, these systems can forecast potential control weaknesses within organizations up to three months before they actually materialize. This advanced capability allows for more proactive responses to emerging issues, potentially preventing larger problems down the road. These systems work by recognizing patterns and trends in historical data, helping auditors develop more precise risk assessments. Furthermore, the ability to predict these control vulnerabilities can lead to better-informed decisions, enabling organizations to stay ahead of potential issues.
While this innovative approach promises greater efficiency and a more proactive stance towards risk management, it's important to acknowledge the complexity involved. The reliance on sophisticated algorithms within these systems can introduce the possibility of errors or biases. This potential for inaccuracies emphasizes the need for human auditors to maintain a critical eye and to validate the output of these advanced systems. Ultimately, as with most AI-powered tools, a healthy balance between the efficiency of automation and the judgment of skilled financial professionals is key to ensure the reliability and trustworthiness of audit results.
Predictive analytics, particularly when coupled with AI, has emerged as a powerful tool for forecasting control weaknesses in various industries, sometimes up to three months before they actually occur. This capability offers a significant shift in the auditing paradigm, moving from a reactive, post-event analysis to a proactive, preventative approach. The ability to see potential problems on the horizon allows auditors to adjust their audit strategies and resource allocation more effectively, focusing their efforts on higher-risk areas.
These systems leverage the power of machine learning to identify patterns and relationships within historical operational and performance data. The algorithms learn from the data, ultimately building models capable of predicting future outcomes. While intriguing, this approach relies on the quality and completeness of the historical data used to train the models. Inaccurate or incomplete data can lead to misleading predictions, highlighting the importance of careful data management in this context.
Interestingly, the use of these predictive tools has been linked to a decrease in audit errors. By anticipating potential control breakdowns in advance, auditors can avoid mistakes stemming from oversight or a failure to identify a developing compliance issue. However, it's essential to recognize that predictive analytics is not foolproof. These models are not always perfect, and they can occasionally produce incorrect forecasts. Consequently, human auditors continue to play a vital role in reviewing and validating the outputs of these systems.
One of the most significant benefits of predictive analytics for audit efficiency is its ability to streamline processes. By targeting high-risk areas identified by the models, auditors can focus their efforts where they're most needed. This shift can potentially reduce the overall time and resources dedicated to the audit process. Of course, this advantage hinges on the reliability of the predictive model's output. If the predictions are frequently inaccurate, it can actually lead to a less efficient audit process.
These systems also offer a degree of adaptability. As new data becomes available, the predictive models can be retrained, refining their capacity to anticipate future issues. This ongoing learning process is particularly valuable in industries experiencing rapid regulatory change or evolving market dynamics. Maintaining the accuracy of the models requires continued attention to ensure that the underlying algorithms keep pace with shifts in the operational environment and avoid producing stale or obsolete predictions.
The availability of forward-looking insights provided by predictive analytics enhances decision-making within audit teams. Instead of solely reacting to past events, auditors can anticipate challenges and devise proactive strategies. This foresight could help organizations avoid costly penalties associated with non-compliance. However, it's crucial that the predictions are communicated effectively within the organization and that the findings are used to drive practical improvements in operational practices. Simply generating predictions without taking action based on the insights gained would limit the true value of this technology.
Early identification of potential control weaknesses can help organizations avoid the substantial financial costs associated with non-compliance issues or penalties. This ability to anticipate and potentially mitigate risks can be a significant driver of cost savings. It is also important to consider that these systems are generally quite complex, and implementing them might necessitate a significant initial investment. Careful evaluation of both the cost and benefits is necessary for organizations considering incorporating predictive analytics into their audit process.
Predictive analytics is capable of offering a holistic view of risk across the different departments and functions within an organization. This comprehensive view is a valuable asset for improving organizational resilience by allowing a more unified and strategic approach to mitigating risk. However, the accuracy of this broad view depends on the accuracy of the individual models used in each department and the effectiveness of communication and information sharing across the organization.
The tools used to develop predictive models often include behavioral analysis components. This capability allows auditors to delve into the "why" behind predicted weaknesses, helping them to gain a better understanding of the contributing factors. It provides a richer context for developing effective compliance strategies. It's important to exercise caution in interpreting these behavioral patterns, as the reasons behind observed behaviors can be difficult to determine conclusively, and incorrect assumptions could lead to flawed conclusions.
The early identification of potential issues through predictive analytics creates a unique opportunity for targeted training and development programs. By anticipating areas of weakness, organizations can invest in proactive solutions to enhance their compliance culture and reduce the likelihood of future issues. This approach can contribute to a more sustainable and robust compliance framework for the organization. However, the training itself must be relevant to the nature of the predicted risks and should be tailored to the needs of the specific individuals within the organization who are most likely to address those vulnerabilities.
Despite the promising advancements in predictive analytics, auditors must retain a critical perspective. The inherent limitations of the models and the potential for biases within the data and algorithms demand continued scrutiny. The integration of predictive analytics into the auditing process is still a developing field, and further research and refinements will continue to shape how these systems are used in the future.
AI-Powered ISO 9001 Audit Analytics 7 Key Efficiency Gains for Financial Auditors in 2025 - Machine Learning Algorithms Cut Report Generation Time From 5 Days to 6 Hours
Machine learning has significantly sped up the process of creating audit reports, bringing the time down from five days to a mere six hours. This substantial improvement allows auditors to devote more of their time and energy to understanding the information revealed by the audit rather than getting caught up in report writing. As AI becomes increasingly integrated into audit processes, the potential for machine learning to streamline tasks and increase efficiency is likely to reshape the financial auditing landscape moving forward. Nevertheless, it's crucial to be wary of over-dependence on automated systems. The use of AI raises legitimate questions about appropriate human oversight and the accuracy of the algorithms used in these systems.
Machine learning algorithms have significantly shortened the time it takes to generate audit reports, reducing it from a five-day process to a mere six hours. This is a remarkable improvement, suggesting that leveraging machine learning's capabilities can drastically cut down on the time previously spent on routine tasks. While impressive, I'm curious how the algorithms themselves maintain their accuracy over time as the types of audits change. I wonder if the developers are incorporating some kind of mechanism to prevent the algorithms from becoming stale or too rigid in their application.
These algorithms possess a learning capability—as they process more data from various audits, their understanding of common patterns and potential deviations improves. This ongoing refinement of the algorithms potentially leads to higher quality audits. However, if the training data is skewed or if there are underlying biases in the way the algorithm is designed, this potential for improvement could be hampered. I believe it's crucial to thoroughly validate the accuracy of these algorithms over time and to ensure that they're updated regularly to incorporate new and relevant audit practices.
One of the advantages of using these systems is the ability to efficiently handle large volumes of data that could easily overwhelm human auditors. This is important because financial institutions often have massive datasets that need to be reviewed. The ability to process these datasets efficiently is a significant advantage, though I am concerned about the potential for bias or errors when the system is processing extremely complex or unstructured data. Furthermore, there needs to be careful consideration of the storage and security protocols for this data.
Interestingly, the use of these algorithms seems to lead to a reduction in human errors during the report generation phase. While this is promising, I am curious about the exact nature of these errors. Are they mostly simple transcription errors or more complex analytical errors? This will have to be carefully tracked and studied to confirm the true efficacy of these algorithms in this area. It's also essential to consider that these algorithms should not lead to a reduction in human oversight. It's vital to ensure that the algorithms are applied responsibly and that human expertise is used to interpret their outputs and validate the results.
While traditionally audit data analysis was a post-event activity, these modern algorithms allow for real-time insights, allowing for quicker reactions to any observed anomalies. This is quite beneficial, though it also leads to the necessity of making sure the decision-making process based on these insights is robust. I am curious if there are methods built into these algorithms to flag situations where human input might be required, or if the algorithms are designed to be fully autonomous in their analysis and conclusions. The implications of the level of autonomy will need to be carefully assessed.
The time saved by the more rapid report generation can be re-allocated to higher-level analysis and strategic decision-making by human auditors. This redirection of effort is promising, but I wonder about the potential for deskilling in the auditing profession. Will the use of these algorithms lead to a decrease in the need for highly skilled auditors, or will it create new types of skilled professionals who are better able to interact with these advanced tools? This is an important issue that will need careful monitoring over time.
The algorithms have the ability to predict potential problem areas based on patterns discovered in previous audit reports. This has the potential to transform audit practices, enabling more proactive risk management, which is quite a valuable development. However, we need to make sure that these predictions are reliable and that the systems are not leading to a false sense of security. It will be crucial to understand how the systems are validated and how any incorrect predictions are tracked and corrected.
These algorithms are easily scalable, allowing them to handle larger amounts of data as an organization grows. This scalability is important, as audit workloads are likely to increase in the future, especially as businesses expand. However, if the systems are not regularly updated or optimized as datasets grow larger and more complex, performance could be affected and results might become less reliable. Ensuring these algorithms remain efficient as they manage large volumes of data is an ongoing challenge.
Machine learning algorithms can be linked to various parts of the audit function. This ability to link different parts of the process creates the opportunity for a more comprehensive view of financial health. However, these integrated systems will become more complex to understand and to debug when things go wrong. This interconnectedness offers benefits but also carries some additional risks, both from a technical and a human-error perspective.
As a final point, these automated systems maintain a thorough audit trail. This is a vital element for regulatory compliance and for instilling confidence in the audit process. However, ensuring the long-term security and integrity of these audit trails will be crucial. It's important to make sure that these records are protected from any attempts to tamper with them or to alter them in any way. Furthermore, clear guidelines about how to access, store, and use this data will be needed to protect the confidentiality of sensitive financial data.
The improvements in audit efficiency driven by machine learning are noteworthy. However, as we continue to develop and utilize these technologies, it's essential to carefully consider the implications. It's not simply a matter of replacing human auditors with machines but rather of creating a collaborative ecosystem where human expertise and AI capabilities work together to produce reliable and insightful audits.
AI-Powered ISO 9001 Audit Analytics 7 Key Efficiency Gains for Financial Auditors in 2025 - Voice-To-Text Technology Transforms Audit Interviews Into Searchable Documents
Voice-to-text technology is revolutionizing how audit interviews are documented and analyzed. No longer limited to basic transcription, this technology now provides highly accurate results, often exceeding 99% accuracy, across a wide range of languages. This means the spoken word during an interview can be instantaneously transformed into a searchable text document, significantly improving the efficiency and depth of the audit process. Auditors can easily capture and retrieve essential information from interviews, allowing for more thorough analysis of the data.
However, with this newfound efficiency comes a potential downside. The reliance on automation raises questions about the possible loss of the subtle cues and deeper understanding that human auditors typically bring to the interview process. Striking the right balance between the speed of technology and the nuanced insights of experienced auditors will be a key challenge moving forward. By seamlessly incorporating voice-to-text into their workflows, auditors can reclaim valuable time previously spent on manual documentation, allowing them to focus on more complex and strategic tasks. The potential for improved efficiency and deeper insights is compelling, but maintaining the right balance between technological advancement and human understanding will be essential for a reliable and insightful auditing process.
Voice-to-text technology has advanced significantly in recent years, evolving from rudimentary speech recognition into surprisingly accurate and versatile tools. These systems can now transcribe audio in a wide range of languages, achieving accuracy rates approaching 99% in favorable conditions. This level of accuracy is impressive, particularly in the context of audit interviews where capturing every nuance of a conversation is crucial.
The speed at which these systems convert spoken language into text is another notable improvement. Auditors can now have a readily available transcript almost instantly after an interview concludes, boosting productivity. It's interesting to consider how this immediacy affects the way auditors approach interviews – are they more focused on the conversation itself when they don't need to scramble to take notes? It seems likely that the reduced cognitive load on note-taking could enhance comprehension and information retention.
Furthermore, the flexibility of input options is quite useful. These systems can handle audio from various sources, including smartphones and conference microphones. This adaptability is helpful in diverse audit environments, making it easier to capture interviews in a variety of settings. I wonder about the potential impact on data quality when using different audio devices – does it matter if it's a high-quality microphone versus a phone recording, for example? There seems to be more research needed in that area.
Interestingly, the algorithms driving these systems are becoming more sophisticated. They're not simply transcribing words; they're starting to understand the context of the conversation. This means that auditors may be able to extract key themes and important information directly from the transcript without having to spend time sifting through the entire text. The implications for enhancing efficiency in that regard are interesting and worth investigating further.
Another noteworthy feature is the ability to archive these transcripts in searchable formats. This capability creates a valuable resource for future audits. Auditors can easily access and review historical interview data to help inform their decisions during current or future audit tasks. It's quite useful for maintaining consistency and completeness across a series of audits.
Beyond simply capturing the conversation, some cloud-based services are introducing real-time collaboration capabilities. Audit teams can now annotate and comment on transcripts during the interview itself, making the audit process more interactive. It's a good example of how technology can enhance teamwork and communication, but there are questions about how to best manage the different inputs and to ensure everyone involved is contributing to the overall goal of the audit.
Data security is a crucial consideration in this context, particularly given the sensitive nature of the information typically discussed in audit interviews. Fortunately, many voice-to-text platforms incorporate security features like encryption and data anonymization. It's crucial that these features are tested rigorously and regularly to ensure that the confidentiality of sensitive financial information is preserved.
Lastly, it's becoming more common to seamlessly integrate voice-to-text transcription services with other audit tools. This type of integration is helpful for streamlining the entire audit workflow. It allows auditors to have a more holistic view of the information they're gathering, from interviews to related documentation.
While this technology is showing promise, there are some lingering questions. How do these systems adapt to different accents and dialects? Can they reliably handle the complexity of financial terminology? What is the potential impact of these tools on the human skills needed for auditing? And how do we ensure that these technologies are used ethically and responsibly?
Voice-to-text technology is clearly evolving rapidly. As it becomes more integrated into audit practices, it will be fascinating to see how it reshapes the audit process. It's likely that the future of auditing will involve a sophisticated interplay between human judgment and AI-driven capabilities, and this technology could play a significant role in that future.
eDiscovery, financial audits, and regulatory compliance - streamline your processes and boost accuracy with AI-powered financial analysis (Get started for free)
More Posts from financialauditexpert.com: