AI-Driven Underwriting The New Frontier in Insurance Risk Assessment as of 2024
AI-Driven Underwriting The New Frontier in Insurance Risk Assessment as of 2024 - AI algorithms revolutionize traditional underwriting processes in 2024
The insurance underwriting landscape is undergoing a dramatic shift in 2024, driven by the power of AI algorithms. These sophisticated algorithms are revolutionizing traditional methods by enabling insurers to assess risk with unprecedented accuracy. The ability to analyze vast datasets in real time, incorporating factors like personal details and online activity, provides a more holistic view of risk profiles. This detailed analysis allows for a move towards customized insurance solutions tailored to individual needs.
Beyond risk assessment, AI is streamlining the entire underwriting process, leading to increased efficiency and a more positive customer experience. The deployment of tools like chatbots and predictive modeling are examples of this automation, further reshaping the industry's operations. While the potential benefits are significant, the integration of AI also brings new challenges. Concerns around the ethical use of personal data and the inherent biases that might be present in these algorithms need to be addressed to ensure fair and equitable underwriting practices. It's a necessary balance to navigate as the insurance sector moves into a more automated, data-driven future.
In 2024, we're witnessing how AI algorithms are accelerating the pace of underwriting. They can analyze massive datasets and extract valuable information from various sources at an incredible speed, surpassing human underwriters' abilities. This speed boost translates to quicker decisions and efficient processing of countless risk factors within seconds.
The capability of natural language processing (NLP) within these algorithms allows them to delve into unstructured data, including social media and online reviews. By understanding the nuances of language, we can get a richer and more nuanced view of a risk profile, providing a more in-depth context for risk assessment than before.
Furthermore, these AI systems learn from each decision and outcome, refining their risk assessments over time. These machine learning models are becoming more sophisticated, enabling them to pinpoint risk patterns that were previously missed by traditional underwriting methods. This includes potentially predicting claim behaviors and detecting fraudulent activity more effectively.
Interestingly, some of these AI systems are discovering subtle correlations between socioeconomic information and claims. This is prompting us to re-evaluate our conventional risk assumptions in underwriting. It's also led to insurers offering personalized policies. We're seeing a shift towards tailored premiums based on individual risk profiles, rather than relying solely on broad demographic segments.
One of the potential upshots of AI-driven underwriting is cost reduction for insurance providers. Theoretically, this could result in lower premiums for consumers while maintaining the same coverage, but only time will tell if these benefits are truly passed on.
However, along with the promise comes some challenges. The inherent "black box" nature of some AI systems raises valid questions about transparency. It can be difficult to understand exactly how the algorithms are assessing risk and making decisions. This lack of clarity might create a barrier to trust for some policyholders.
The use of predictive analytics in underwriting is becoming increasingly sophisticated, allowing insurers to forecast future risks more precisely. These insights are impacting a range of areas, influencing everything from how premiums are set to broader insurance strategies.
As this field continues to evolve, concerns about bias in algorithmic decision-making are rightfully coming into focus. It's crucial for insurers to ensure fairness in underwriting practices and prevent any perpetuation of existing biases or inequalities in their algorithms. This is an essential ethical discussion within this evolving technological landscape.
AI-Driven Underwriting The New Frontier in Insurance Risk Assessment as of 2024 - Machine learning enhances risk profiling accuracy using big data
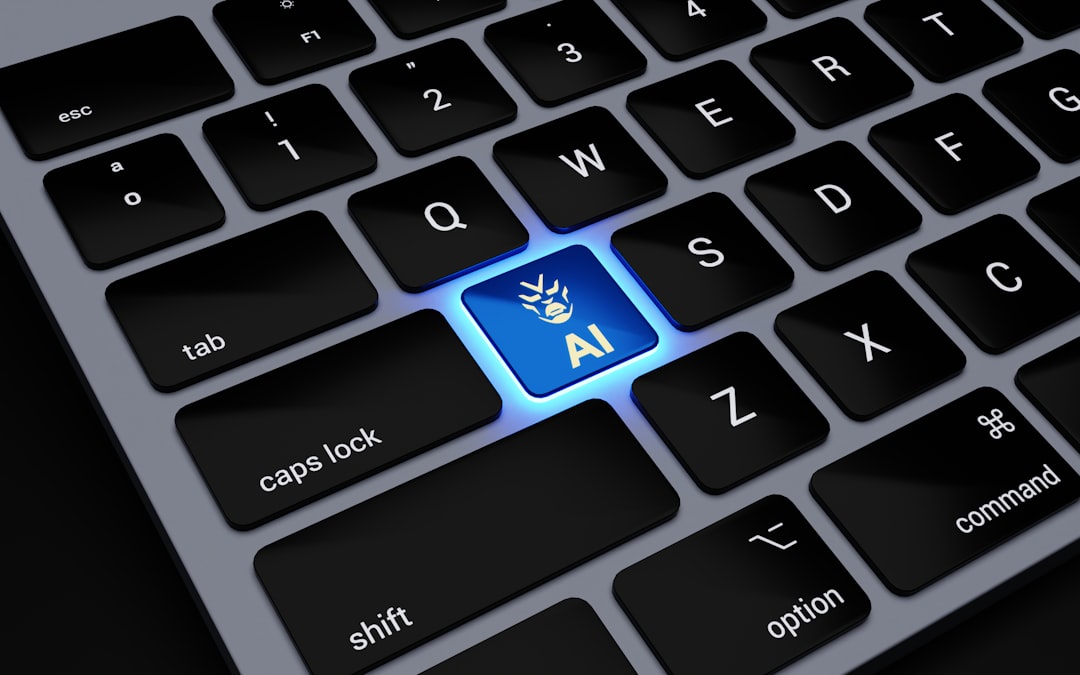
The application of machine learning techniques is significantly improving the precision of risk profiling within insurance underwriting by harnessing the power of big data. This allows insurers to consider a much broader range of factors in their assessments, from genetic and demographic details to online behavioral patterns. The result is a more sophisticated understanding of individual risk profiles. These advanced algorithms are identifying patterns and correlations that were previously missed by traditional methods, leading to more accurate risk prediction and potentially better identification of high-risk individuals or groups. Machine learning also offers the possibility of refining risk calculators and assessment models, ultimately enhancing the overall accuracy of underwriting.
However, the integration of these powerful AI tools isn't without its challenges. The complex nature of some machine learning algorithms can make it difficult to understand exactly how they arrive at their conclusions, raising concerns about transparency and the potential for bias in decision-making. While promising greater efficiency and accuracy, the industry must navigate the complexities of ensuring fair and unbiased underwriting practices as AI plays a larger role. This is a crucial consideration as insurers move further into the realm of AI-driven underwriting. The path towards fully integrated AI solutions in the insurance space is undoubtedly still evolving and will require careful consideration of these complexities.
Machine learning is significantly enhancing the precision of risk profiling by leveraging the wealth of data available through big data. By incorporating a broader range of factors, including genetic, imaging, and demographic data, machine learning has empowered organizations to gain a much more nuanced understanding of risk. This shift has the potential to improve risk assessment accuracy across a wide spectrum, though researchers are still investigating its practical application.
We're witnessing a shift away from traditional risk assessment models that rely on static parameters. Machine learning models offer a dynamic approach, continually adapting and refining risk profiles based on the influx of new data. This allows insurers to respond more effectively to emerging risks and adapt to changing circumstances in real-time, leading to a more agile and responsive underwriting process.
Interestingly, this capability has opened up avenues for exploring individual behavioral patterns and their correlation with risk. For example, we can now analyze social media activity and identify patterns that may hint at future claim likelihood. It's a fascinating area of research that expands beyond the traditional reliance on demographic factors.
The application of machine learning isn't confined to traditional data types. The rise of visual data analysis through computer vision allows for assessing property conditions from images and videos, revealing insights that questionnaires might miss. These advancements are reshaping the way we approach risk assessment in various insurance areas.
One of the most promising applications of machine learning is its potential for fraud detection. The ability of machine learning algorithms to identify patterns and anomalies hidden within vast datasets is impressive. Some preliminary studies suggest significant reductions in fraudulent claims, indicating a potential for considerable improvements in claim management. While these are encouraging early findings, more research is needed to validate these results broadly.
Predictive modeling has moved beyond its initial role in risk assessment, now impacting strategic decisions within insurers. The capability to anticipate future risks and trends is changing how products are offered and marketing campaigns are planned. This broader influence of AI is leading to a shift in the way insurance businesses operate.
Despite the benefits, there are still hurdles to overcome. The initial costs associated with building the necessary data infrastructure and developing these complex algorithms can be substantial. The question of whether these investments will ultimately translate into long-term financial advantages for insurers remains open. Furthermore, as algorithms become increasingly complex, maintaining transparency becomes more challenging. It's crucial for insurers to balance model sophistication with the need for understandable and explainable decision-making processes to maintain trust and transparency with clients. This is a key area of research and ethical consideration as we move towards a more AI-driven insurance landscape.
AI-Driven Underwriting The New Frontier in Insurance Risk Assessment as of 2024 - Automated workflows reduce human error and increase efficiency
Automated workflows are proving valuable in the underwriting process by reducing human error and boosting efficiency. Automating repetitive tasks through these workflows allows insurers to expedite risk assessment and ensures more consistent decision-making. The ability to analyze massive datasets with AI tools significantly lowers the chances of mistakes often associated with manual review. As these automated systems become more common, the expectation is that underwriting will become more dependable. However, ongoing monitoring of these AI-driven workflows is crucial to identify and address any potential bias or lack of transparency issues that might emerge. As the insurance sector embraces AI further, finding the balance between efficiency gains and ensuring ethical practices will be paramount.
Automating workflows within underwriting has the potential to significantly reduce human error, a major source of inaccuracies in traditional processes. This reduction in errors stems from the ability of machines to consistently execute predefined tasks with a level of precision that often surpasses human capabilities, particularly when dealing with repetitive data entry or complex calculations. It's not just about speed, though that is a benefit. We're talking about removing the potential for simple mistakes that can cascade through an underwriting process. For example, a small typo in a policyholder's date of birth, if missed, can cause delays and frustrations down the line. While humans will always be fallible, automation attempts to create a robust system to minimize these mistakes.
Workflow automation can greatly enhance efficiency by expediting processing times and increasing the overall throughput of applications. The faster turnaround times not only satisfy customers but also help insurers handle larger volumes of applications, which is especially relevant in a growing and competitive market. But we need to consider if speed always translates to quality. It's a balance we need to carefully assess, especially in underwriting. Just because it's faster doesn't mean it's better.
Another interesting aspect is the enforced consistency in data handling that automation brings. When tasks are automated, the same set of criteria is used for every application. This standardization reduces potential for subjective bias in evaluating risk profiles. This is a subtle yet important aspect of AI. We need to think about how these systems will maintain fairness and be impartial in their assessment.
Furthermore, the ability to log and analyze errors within automated workflows offers insights into the process itself. We can observe where things are going wrong and adjust accordingly. This is critical to the long-term optimization of an underwriting process, because it allows us to improve how we design the system, and perhaps reveal flaws that might have otherwise been missed. This constant evolution through iterative improvement is crucial to reaping the full potential of this technology.
Automation also offers scalability, enabling insurance companies to adapt more quickly to changes in business volume or market conditions. We don't have to dramatically increase our workforce with every spike in demand. This can make the insurance business more responsive to changing external factors. However, this comes with a caveat. We need to understand if automation only increases the efficiency of processes or if it fundamentally changes what we expect of insurance.
Integrating predictive analytics into automated workflows presents interesting possibilities. By flagging potential errors early in the process, before a final assessment, we create a proactive layer of quality control. These tools can be used to identify unusual data patterns or conflicting information that might suggest the need for human review, helping to catch issues earlier in the flow.
Real-time data processing is another benefit that automation brings. Insurers can rapidly respond to dynamic risk factors like shifts in market conditions or regulatory changes. This can be useful in navigating increasingly volatile insurance landscapes. We're not just looking at historical trends, but actively looking at immediate changes in risk that may impact the current environment. However, it's important to consider how these rapid responses impact overall fairness and how it could be gamed.
By removing human limitations, such as cognitive bias or the ability to process extremely large datasets, we can develop more nuanced risk assessment models using AI. This translates to potentially more accurate assessments of individual or group risk profiles. This also opens a can of worms. If we are able to predict behaviors with greater accuracy, do we create a future of underwriting based on predicted behavior rather than current actions? Is this fair or are we introducing a form of pre-crime profiling in insurance?
Finally, the potential for cost savings is a key driver for many insurers exploring automation. Estimates vary, but reductions in operational costs can be substantial, perhaps leading to lower insurance premiums for consumers. But it's important to remain skeptical. We need to see if these cost reductions are passed along to consumers or whether they simply result in increased profits for the companies adopting them.
These are all areas to continue to research and analyze as this rapidly evolving technology matures.
AI-Driven Underwriting The New Frontier in Insurance Risk Assessment as of 2024 - Real-time data integration enables dynamic policy adjustments
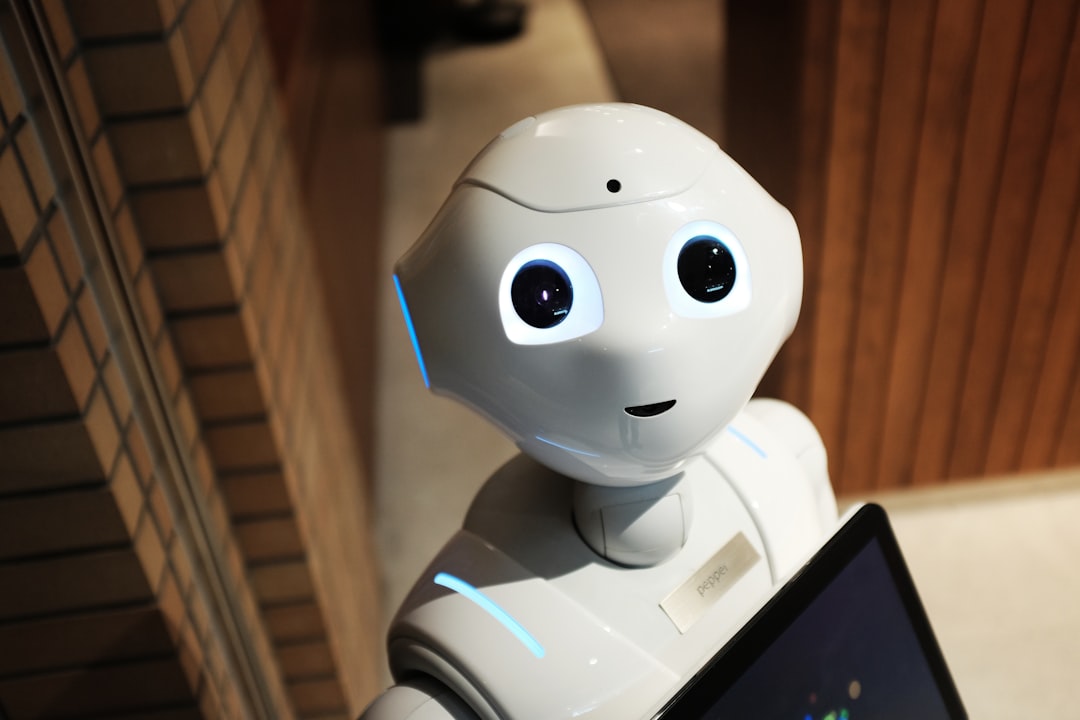
The integration of real-time data is fundamentally altering how insurance companies manage risk. It allows for dynamic policy adjustments, meaning insurers can react quickly to changes in a policyholder's risk profile and adapt to shifts in the insurance market. This adaptability leads to a more tailored approach to insurance, providing coverage that better aligns with individual circumstances. Furthermore, real-time adjustments streamline operations by eliminating the reliance on outdated information, promoting a more efficient process. However, the speed of these adjustments also raises questions about fairness and the potential for biases to be embedded in the algorithms used for risk assessment. Moving forward, it's crucial that the industry carefully considers ethical implications of data usage to ensure equitable underwriting practices. The transition to this new era requires navigating complex considerations if the potential benefits of real-time data integration are to be fully realized.
The integration of real-time data into the underwriting process is a significant development, allowing insurers to adapt policy terms dynamically. This means that, instead of relying on static data points, insurers can now react to changes in a policyholder's risk profile almost instantly. For instance, if someone's driving habits shift, or if there's a sudden increase in crime in their neighborhood, the insurance policy can be adjusted accordingly, reflecting the newly identified risks. While this dynamic approach to policy management seems like a promising development, we need to think about the potential for it to create a much closer relationship with the client – perhaps too close. It could mean policies are adjusted extremely frequently, requiring a constant awareness and engagement with the client.
This real-time data flow also enables insurers to use very granular datasets, such as neighborhood-specific crime statistics or localized weather events. This level of detail allows for much more precise risk assessments, especially within property and casualty insurance. However, using this hyper-local data has potential ethical ramifications. Will it inadvertently create biases in pricing, favoring certain areas over others, and potentially even deepening existing inequalities in access to insurance? It's a consideration for the future of this field.
Additionally, the capability to process real-time data provides an opportunity to glean insights into a policyholder's behavioral shifts. Imagine a scenario where a car insurance policy adjusts itself automatically if the vehicle is being driven more frequently, potentially leading to a slight increase in premiums. This presents a potential shift in how risk is managed, from infrequent checks to a continuous stream of evaluation of individuals and their habits. But what if these behaviors are misinterpreted or manipulated to the advantage of the insurance company? Are we creating a system that incentivizes constant vigilance and monitoring of individuals' lives?
Furthermore, the ability to react to regulatory changes almost immediately means insurers can better ensure ongoing compliance. They can automate adaptations to new legislation, ensuring that their products remain compliant. While this seems efficient, we have to consider the potential pitfalls. Are we creating systems that are less human-centered and potentially over-reliant on automation? Who is responsible if the automatic adjustment causes harm?
Lastly, this dynamic adjustment capacity can foster a competitive edge for insurers. By using real-time data to tailor policies and prices quickly, some companies could gain an upper hand over competitors. However, we must consider the consequences of this competitive environment. Could it lead to an even more fragmented and competitive insurance market, potentially causing instability? Would we see pricing wars based on this ability to adapt, or would it encourage more collaboration between providers?
Overall, the integration of real-time data for dynamic policy adjustments is a technological leap that warrants further investigation and careful consideration. It promises more personalized and flexible insurance, but it also raises significant questions regarding the balance between responsiveness, fairness, and privacy in the underwriting process. This is a rapidly evolving field with the potential to reshape how we view insurance, and it's essential to engage in these discussions as it unfolds.
AI-Driven Underwriting The New Frontier in Insurance Risk Assessment as of 2024 - Ethical considerations in AI-driven insurance decisions
The rapid adoption of AI in insurance underwriting in 2024, while promising greater efficiency and accuracy, also brings forth a new set of ethical dilemmas. The displacement of human underwriters, as seen in some instances, highlights the need for careful consideration of the social impact of AI integration. This includes the potential for job losses and the need for clear accountability in the decision-making process when algorithms are in charge.
Beyond job displacement, utilizing external consumer data in underwriting raises serious questions about the protection of personal information and the fairness of risk assessments. The algorithms driving these systems could inadvertently perpetuate existing biases or create new ones, potentially leading to discriminatory practices in pricing or coverage. Furthermore, the increasing sophistication of predictive analytics brings with it a new challenge: the ethical implications of using AI to predict future behavior and its potential impact on individual fairness within the insurance context.
These issues underscore the urgent need for ongoing discussions and collaborative efforts between insurers, regulators, and other stakeholders. The establishment of clear ethical guidelines and frameworks for AI in insurance is crucial to ensure that the benefits of these technologies are harnessed while minimizing the risks of unintended consequences. Navigating these complexities will be essential in shaping a future where AI-powered underwriting is both effective and equitable.
The increasing use of AI in insurance underwriting, while offering exciting possibilities, has introduced a new set of ethical considerations we need to carefully examine. One major concern is the reliance on vast amounts of personal data. AI systems often need access to a wide range of information to accurately assess risk, but this raises worries about the protection of individual privacy and the balance between efficiency and data governance. We need to thoroughly consider the ethical implications of how these frameworks are designed and applied.
Another significant concern is the potential for algorithmic bias. Even the most advanced AI algorithms can carry biases present in the data they're trained on. This could lead to unfair treatment of certain groups, especially if there's an inherent skew in the data regarding demographics, income, or other factors used in risk assessment. The consequence of this could be policies that disproportionately penalize certain groups, perpetuating inequalities.
Furthermore, the complex nature of AI can create transparency issues. Often, the decision-making process within these algorithms is hard to fully understand, especially by those whose insurance is being assessed. This can erode trust, as individuals might struggle to understand how the data they share impacts their insurance costs or coverage options. If we are to build public trust, this needs to be carefully considered.
The capability of AI to continuously monitor risk profiles based on real-time data brings a notable shift to the insurance world. However, this constant assessment can lead to abrupt changes in premiums, potentially creating uncertainty and resentment from customers if the process isn't transparent or fair. We need mechanisms in place that ensure equitable changes in insurance coverage or pricing and maintain customer confidence.
The use of predictive analytics to estimate future claim behaviors is another area with ethical implications. While useful for refining underwriting processes, there's a risk of drifting into a kind of preemptive profiling based on potential future behaviors rather than past actions or current circumstances. This raises questions of fairness and whether we want an insurance world built on predicted behaviors versus a track record of actions.
The increasing use of socioeconomic information alongside standard risk assessment data offers greater insight into risk. But this raises concerns about potential biases that could unfairly disadvantage those from lower socioeconomic groups and potentially exacerbate inequalities in insurance access.
In a world of AI-driven insurance, informed consent is a significant challenge. Consumers might readily agree to have their data used for various purposes but without fully understanding the implications of this in the context of real-time monitoring. We must be clear about what is being collected and how it's used.
The ability to customize insurance policies based on constantly updated data poses a challenge to traditional insurance models. We need to think carefully about how these dynamic policies are structured and communicated to customers. It's important that they remain transparent, easy to understand, and fairly priced, while offering the flexibility to adapt to changing circumstances.
The shift to constant data-driven interactions could weaken the historically trust-based relationship between insurers and policyholders. The potential for frequent adjustments to policies, based on data, might erode the feeling of stability and consistency individuals associate with insurance.
Finally, the potential to automate compliance with ever-changing regulations is efficient but brings up questions about human oversight. We need to be mindful of not solely relying on automated systems. There's a risk of accountability and responsibility gaps if something goes wrong within a completely automated compliance system.
These issues are all areas where ongoing research and discussion are crucial. AI-driven insurance is a rapidly developing field with immense promise, but we must address these ethical considerations to ensure it benefits everyone fairly and builds a future where insurance remains a vital source of security and support for everyone.
AI-Driven Underwriting The New Frontier in Insurance Risk Assessment as of 2024 - Regulatory challenges and adaptations for AI underwriting models
The increasing reliance on AI underwriting models in 2024 presents a complex landscape of regulatory hurdles. Balancing the drive for innovation with the need for responsible AI deployment is paramount. Concerns about algorithmic bias, especially as these systems process vast amounts of data, are at the forefront. Ensuring AI models don't unfairly disadvantage specific groups, or replicate societal biases, is crucial for maintaining fairness in underwriting practices. Additionally, the inherent opacity of some AI algorithms, often referred to as the "black box" problem, raises questions about accountability and transparency in risk assessments. Regulators and the industry are grappling with how to maintain oversight while fostering innovation.
To address these issues, a dynamic adaptation of regulatory frameworks is essential. This includes finding ways to ensure that AI models are understandable, or at the very least their decisions are verifiable, by human review. Moreover, consumer protection must remain central. Balancing the need for speed and efficiency with safeguards to prevent misuse of personal data is a delicate tightrope walk. The potential impact on employment and the evolving roles of human underwriters also needs consideration. Ultimately, a collaborative effort involving insurers, regulators, and consumer advocates is crucial to ensure that the transition to AI-driven underwriting is both beneficial and equitable. This will require open dialogue and an ongoing reassessment of best practices to prevent unintended negative consequences.
The rapid rise of AI in underwriting is pushing regulatory bodies worldwide to create new guidelines specifically for these technologies. A key focus is likely to be on ensuring insurers can explain how their AI algorithms make decisions. This is a tricky challenge given the complex, often opaque nature of many machine learning models.
Companies are becoming more aware of the potential for biases within their AI systems, which has led to initiatives to formally evaluate the fairness of these models. These "fairness audits" serve a dual purpose—helping with regulatory compliance and risk management. If an AI system is found to be unfairly discriminating, it could lead to lawsuits, so proactive auditing is potentially a way to prevent negative financial consequences.
One of the more complex issues is the varied regulatory approaches we're seeing globally. Each country, influenced by its own cultural, legal, and economic landscape, is taking a slightly different route. This creates a challenge for large insurers that operate internationally, as they now must comply with a patchwork of overlapping or conflicting regulations. Keeping track of these changes, and having compliance teams able to adapt, is a new operational hurdle for the industry.
Data protection regulations, such as GDPR in the EU, are making it very clear that the collection of data for AI underwriting must be carried out responsibly and transparently. Companies failing to comply with these standards can face large fines or damage their reputation.
Further complicating things is the fact that some AI models are designed to constantly adapt to new data. Regulators will need to create standards that keep up with this speed of change. Insurers will need to continually evaluate their AI systems to ensure they adhere to any updated regulations and evolving expectations.
Companies that prioritize transparency in their use of AI could potentially gain a competitive edge. More and more consumers care about how businesses are using their data, and they are often more willing to trust organizations that are upfront and ethical in their practices. This focus on trust and transparency may drive customer loyalty and attract new clients.
In response to the pace of innovation, some insurers are proactively collaborating with regulators to establish new standards. This approach allows regulators to understand the capabilities and potential risks of these new AI systems and enables insurers to design their AI solutions in a way that satisfies regulatory requirements from the beginning. This hopefully avoids having to retool systems later on.
We may see the future emergence of legal frameworks that hold insurers responsible not only for the results produced by their AI algorithms but also for the methods behind them. If this occurs, insurers will need to develop and implement very rigorous oversight and control systems for their AI underwriting processes.
The use of personal behavioral data is a complex issue that needs careful attention, especially when considering the aspect of informed consent. Insurers need to communicate clearly what data they are collecting, why they are doing so, and how that data will be used. Consumers should understand their rights in this environment, and it is important that there are strong safeguards to protect consumers' privacy.
Industry organizations are beginning to create ethical guidelines for the use of AI in insurance. These guidelines focus on promoting fairness, transparency, and accountability in the hopes of building consumer trust in these systems. This sort of collaborative approach could hopefully lead to a common standard of practice across the sector, simplifying compliance and fostering confidence amongst the insured public.
While the future of AI in insurance holds a lot of promise, the legal and ethical challenges surrounding it are rapidly evolving. Navigating this landscape will require ongoing research, proactive collaboration between stakeholders, and a commitment to building and deploying AI systems that prioritize fairness, transparency, and ethical practices.
More Posts from financialauditexpert.com: