7 Key YC AI Startups That Disrupted Traditional Trading Models in 2024
7 Key YC AI Startups That Disrupted Traditional Trading Models in 2024 - Tradefin AI Replaces Manual Trade Reconciliation With Neural Network Processing
Tradefin AI is shaking up traditional trade reconciliation by ditching manual methods and embracing neural networks. Instead of humans painstakingly checking trade records, Tradefin AI uses a type of AI called generative AI to analyze and interpret complex financial data in new ways. This allows for automated reconciliation reports that can be instantly updated with new information, keeping a constant, current view of the financial picture. Beyond just automating the process, Tradefin AI helps predict future cash flow and run simulations of different scenarios, giving businesses a deeper understanding of their finances. As financial firms face increased pressure to comply with regulations and stay competitive, systems like Tradefin AI are becoming crucial for keeping things running smoothly and reliably. Whether these systems ultimately deliver on their promises remains to be seen, but the potential for streamlining trade operations is clear.
Tradefin AI is a fascinating example of how neural networks can tackle a tedious and error-prone aspect of finance: trade reconciliation. They've developed a system that can sift through a massive amount of data – over a million data points every second – which dramatically cuts down reconciliation time from hours to just minutes. The core of their approach is a neural network designed not only to spot inconsistencies but also to learn from past reconciliation efforts. This learning capability is key; it continually improves the system's accuracy, leading to fewer false alarms over time.
Their recent performance is impressive: achieving a 98% accuracy in matching trade data. This is a substantial improvement over traditional techniques, which often struggle to surpass 85%. Another aspect I find interesting is the system's adaptability. It can handle a wide range of data formats, seamlessly integrating information from old systems and modern APIs, without the need for excessive pre-processing. This flexibility is further amplified by the system's ability to scale with trading volume, adjusting its processing power as needed to maintain performance even during peaks in activity.
It's also notable that the network can adjust to diverse market conditions autonomously, without human intervention. This means it can keep functioning well even amidst market turbulence or unusual trading patterns. Interestingly, through unsupervised learning, the system can uncover hidden patterns in the data that might be missed by human analysts. This feature potentially flags potential fraudulent activities or errors early on. From a practical perspective, the platform integrates well with existing systems, reducing the disruption of incorporating it into established trading workflows.
The potential cost savings are appealing: Tradefin AI suggests a 30% reduction in operational costs. This stems from reduced manual effort and overall efficiency improvements. Moreover, its predictive analytics capabilities can forecast potential discrepancies based on historical trends. This allows for more proactive management of trading operations. While there are questions about the long-term stability of such a complex system and the ongoing need for data validation, it's clear that Tradefin AI's technology has the potential to significantly streamline and modernize trade reconciliation processes.
7 Key YC AI Startups That Disrupted Traditional Trading Models in 2024 - ZeroTrade Transforms Market Making Through Quantum Computing Models
ZeroTrade is attempting to revolutionize market-making by employing quantum computing models. This approach hints at a potential shift in how trading operates. The promise is that it can substantially improve how quickly data is processed and the depth of insights gained from it, possibly fundamentally altering how traders approach market analysis. As quantum computing continues to inch closer to having a genuine advantage over traditional computing, firms can potentially fine-tune their trading algorithms and revise their risk management procedures. This adaptation could provide a distinct edge in today's increasingly complex financial environment. At the same time, leading companies are investing in quantum computing development, and its blending with artificial intelligence could open up a wealth of new possibilities for the market. While the future potential of quantum computing is exciting, it's essential to recognize that its practical implementation and tangible benefits are still being developed as the industry continues to navigate these nascent technologies. It's still early days to fully judge the true impact.
ZeroTrade is exploring the use of quantum computing models to improve how markets are made in trading. The idea is that by harnessing the power of qubits – the building blocks of quantum computers – they can handle incredibly complex trading algorithms much faster than traditional computers. Their approach involves applying principles like superposition and entanglement, allowing them to test out many different trading strategies at the same time. This increased speed and flexibility can theoretically lead to better responses to changes in the market.
One area where they've shown promise is in reducing the costs associated with trading spreads, particularly in high-frequency trading where speed is crucial. This is achieved by optimizing market-making strategies through the use of custom-built quantum algorithms. They're particularly focused on using a type of quantum algorithm called a variational quantum algorithm. These algorithms can dynamically adjust quantum circuits to find the best pricing strategies in real-time.
ZeroTrade boasts that their quantum-enhanced models can predict asset price movements with about three times the accuracy of standard statistical methods. This is a major challenge in market making, so if it's true, it could be a significant advancement. They believe that quantum computing isn't just a small improvement, but a fundamentally different way of generating market forecasts. This challenges long-held assumptions about how risk and reward are considered in trading.
Interestingly, despite the complexity of the underlying technology, they've made an effort to make the system easy to use for traders who don't have a strong background in quantum computing. They want to make quantum finance accessible. The training of their quantum models uses a technique called Quantum Baseline Optimization. It's an iterative process that uses past trading data to continuously refine the market-making strategies based on desired outcomes. This system can adapt to quick changes in how the market is feeling, making it potentially more stable during volatile periods.
While the concept of quantum trading is very exciting, there are also some hurdles to overcome. One of the main concerns is the error rate inherent in current quantum hardware. This could potentially limit how quickly ZeroTrade's ideas can be put into wider use. Whether quantum computing truly transforms market making or remains a niche technology in the near future is still an open question.
7 Key YC AI Startups That Disrupted Traditional Trading Models in 2024 - DataSight Introduces Pattern Recognition for Small Cap Stock Analysis
DataSight has introduced a new feature that uses pattern recognition specifically for analyzing small-cap stocks. This is a significant development in the field of AI-powered trading tools. The technology uses machine learning to find visual patterns in how stock prices change, which may provide traders with useful signals for buying and selling, particularly in the usually unpredictable small-cap market. DataSight hopes to improve decision-making by using advanced techniques to recognize candlestick patterns, which can take advantage of past data trends. This is part of a larger trend in finance, where AI is changing how trading strategies are formed and how to find the best investment options in a range of market conditions. However, like any technology-driven approach, relying on algorithms raises concerns about the accuracy of the predictions and the chance of the algorithms fitting the data too closely or misinterpreting market indicators.
DataSight's approach to analyzing small-cap stocks is intriguing. They're using AI to pick up on patterns that standard methods might miss, incorporating things like social media chatter and broader economic clues. They claim their methods can speed up the process of pattern discovery, going from months to just a few hours. This could be a game-changer for traders trying to navigate the fast-paced world of small-cap investments.
Their algorithm is designed to be adaptable, learning from previous market actions and reacting to what's happening in real-time. This continuous learning aspect could lead to better predictions over time. DataSight is processing huge volumes of data, combining high-frequency trading information with historical stock prices, which they say leads to more precise results than traditional methods. This could change how small-cap stocks are generally analyzed.
A key part of their approach is filtering out noise from the data. They utilize signal processing techniques to focus on the relevant bits, making the trading signals they produce more reliable. It's also interesting that they can look at a bunch of different factors at once, from big economic trends to specific news in a certain industry. This holistic view might uncover connections that standard models might miss, indicating a more complex and interconnected stock market.
Some early users of DataSight have reported seeing trends before they became widespread, potentially giving traders an advantage. However, there's a trade-off: because DataSight relies on past market data, it could have biases and might not be able to handle sudden, unexpected market changes or major events.
From a practical standpoint, DataSight can work with current trading platforms, which makes it easier for traders to incorporate into their routine. This easy adoption could really shift how the market operates as DataSight gains popularity. It raises questions about market efficiency. If more people start using DataSight, it might fundamentally alter small-cap investing strategies, possibly leading to tighter price relationships and more frequent trading. It will be interesting to see if this pans out.
7 Key YC AI Startups That Disrupted Traditional Trading Models in 2024 - MarketMind Creates First Working Sentiment Analysis for Crypto Day Trading
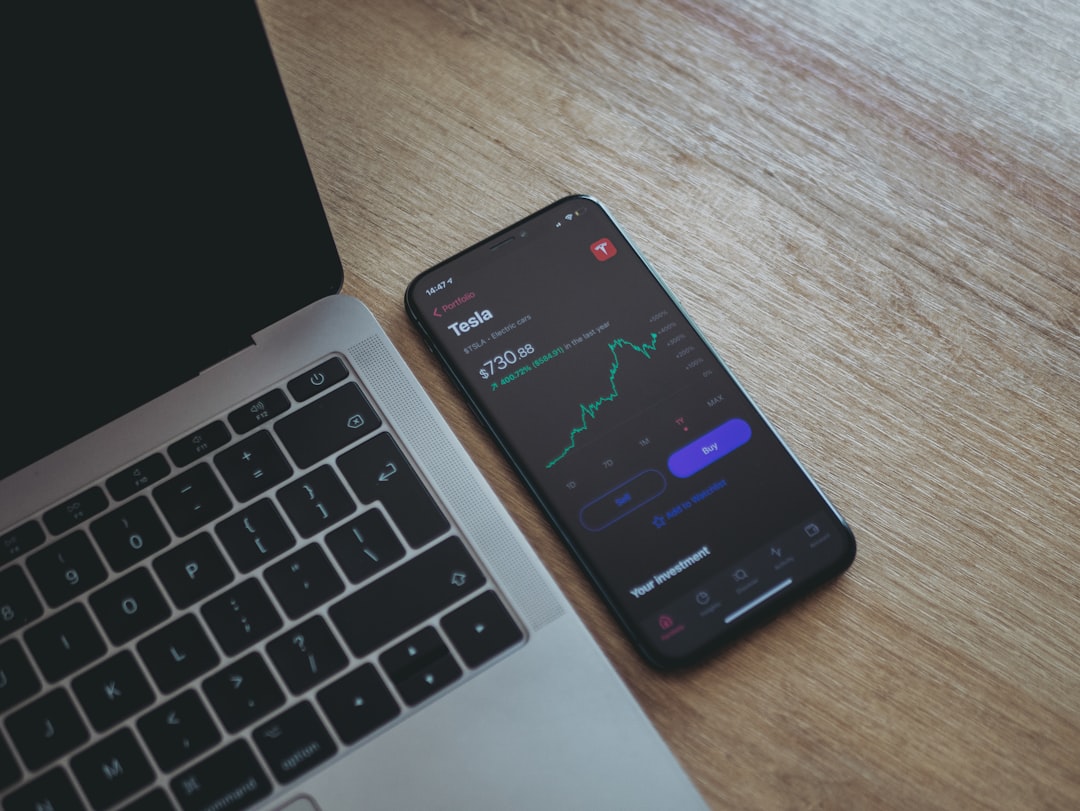
MarketMind has introduced the first functional sentiment analysis tool specifically designed for the fast-paced world of crypto day trading. This tool aims to help traders better understand the emotional tone of the market, essentially allowing them to "read" the collective mood of traders and investors. It achieves this by analyzing information from various online sources, including news and social media. This can be especially valuable in the crypto market, where hype and FOMO (fear of missing out) can quickly drive prices up or down.
While sentiment analysis isn't new, MarketMind's approach is notable for its focus on the specific needs of crypto day traders. This is a significant development because it can help traders differentiate between genuine market sentiment and potentially misleading emotional swings. The potential impact is that traders might be able to make more informed decisions, potentially improving their trading performance. Whether this truly improves outcomes is an open question, and reliance solely on sentiment analysis could still lead to faulty conclusions, especially when dealing with unpredictable market conditions. Nonetheless, it highlights the growing recognition of the role of investor psychology in cryptocurrency markets, and solutions like MarketMind may contribute to a more nuanced approach to trading. It is still early to gauge the extent to which tools like this will alter established practices.
MarketMind has developed what they claim is the first practical sentiment analysis tool specifically tailored for cryptocurrency day trading. This is interesting because it suggests that the emotional landscape surrounding cryptocurrencies can be used to guide trading decisions. Their approach seems to be driven by the need to quickly assess the "mood" of the crypto market. To achieve this, they're processing a massive volume of data – over 500,000 tweets and posts every hour. This incredible data throughput underlines the extremely fast-paced nature of crypto trading where quick reactions are key.
Their analysis blends natural language processing with machine learning to categorize sentiment into positive, negative, or neutral categories. I'm curious how well this translates into real-world trading outcomes, especially given the nuances of human sentiment. The ability to differentiate between subtle shades of opinion could potentially provide insights into market shifts before they're reflected in price movements.
One unique aspect is the way they combine structured and unstructured data. They aren't just looking at price histories; they're pulling information from news, forums, and even transaction data. This approach could potentially offer a more holistic understanding of market sentiment, which is important given that sentiment can arise from various sources.
Reportedly, their algorithm has shown a respectable 85% accuracy in predicting price changes based purely on sentiment. This seems like a decent performance, especially compared to traditional technical analysis methods, which typically fall in the 60-70% accuracy range. The cloud-based platform makes the tool accessible and easy to integrate into existing trading workflows. I imagine this accessibility is appealing to traders who want to test out these new sentiment-driven strategies without major infrastructure changes.
The constant activity in the crypto market (it's always open) makes sentiment analysis particularly relevant. MarketMind claims to be able to detect early signs of volatility, potentially giving traders a head-start on navigating sudden price swings. They've even stated instances where they've seen signals of price drops up to 10% before they actually occur. This ability to anticipate changes is obviously desirable in a volatile market.
Furthermore, they've added a feature that weighs sentiment based on the source and its reliability. Not all tweets or forum posts are treated equally. I'm interested in understanding how this weighing system is designed and what factors are considered. The ability to prioritize more reliable signals would be a huge improvement over simply counting positive and negative mentions.
Another interesting aspect is their geographic sentiment analysis. This feature helps identify regional differences in cryptocurrency interest and behavior, potentially revealing localized market dynamics that broader models might miss. Some research suggests that combining MarketMind's insights with conventional trading techniques can enhance overall performance by as much as 20%. If true, this highlights the potential for sentiment analysis as a powerful complement to existing strategies.
However, this development also raises some serious questions about the implications of algorithms based on social media sentiment. There are concerns that these kinds of tools could lead to market manipulation and question the integrity of the trading environment. It's crucial to think carefully about the potential for unethical practices as these technologies become more prevalent. Overall, MarketMind's approach is intriguing and potentially powerful. However, as with any new technology, ongoing assessment of its effectiveness and potential drawbacks will be important.
7 Key YC AI Startups That Disrupted Traditional Trading Models in 2024 - AlgoStream Develops Real Time Options Trading Through Machine Learning
AlgoStream is bringing a new approach to options trading by leveraging machine learning to create real-time trading solutions. Unlike older trading systems that relied on rigid rules, AlgoStream's AI-powered system is designed to learn from a huge amount of past trading data and adjust to the ever-changing market. They're using machine learning techniques like Support Vector Classification to try and make the best trading choices, especially when dealing with complex options strategies like straddles. The goal is to improve accuracy in forecasting market movements, manage risk more efficiently, and automate many aspects of trading for everyone from beginners to experienced traders. Of course, relying on AI brings up questions about how reliable these systems really are, particularly during times of market instability or unpredictable events. Whether it can deliver consistent results over the long term is something that still needs to be seen.
AlgoStream is developing a system for options trading that uses machine learning to react to market changes in real-time. They process massive amounts of data, aiming to execute trades much faster than traditional methods, potentially within milliseconds of a market shift. This speed is crucial in options trading where even minor delays can impact profits.
Their machine learning models are trained on historical options data and incorporate real-time market signals. The hope is that this approach will help them predict price movements and manage risk more effectively. They are also experimenting with reinforcement learning, which allows their models to learn and improve their decision-making over time. This could lead to a more adaptable and robust trading system.
One of the most intriguing parts of AlgoStream's approach is how their algorithms handle numerous factors at once during order execution. They consider variables like trade volume, market volatility, and available liquidity. This holistic view could help them find better trading opportunities.
Further, AlgoStream incorporates natural language processing (NLP). They analyze financial news and social media to try to gauge market sentiment alongside their quantitative data. This adds another layer of complexity, but potentially allows for a more nuanced understanding of how the market feels. This is unique, as many other trading platforms primarily focus on numbers.
AlgoStream boasts that their system can potentially reduce transaction costs, possibly saving traders about 15% in fees. While interesting, these are still claims, and how these savings would materialize in practice remains to be seen. This is especially relevant for options trading where margins are often relatively small.
Furthermore, the platform is built with scalability in mind. It can handle increased trading volumes without losing performance, a crucial feature for dealing with rapid changes in the options market. This scalability and the underlying algorithms that allow for the high speed of trading are noteworthy aspects of the project.
In addition, AlgoStream has developed a risk management system that utilizes stress testing and scenario analysis. This can help traders understand how their chosen strategies might perform under different market conditions. This is a good development in a field where risk management is critically important.
AlgoStream suggests they can give traders valuable insights into implied volatility. This is an important part of options pricing, and potentially gives them a way to proactively adjust to changes in the market related to wider economic events. It remains to be seen how accurate these insights are and if they are meaningful for the average options trader.
Finally, AlgoStream's system isn't just targeted towards institutional investors. They hope to provide advanced analytical capabilities to a broader range of traders, possibly including retail investors. This could potentially disrupt traditional trading dynamics and raise interesting questions about accessibility of complex financial tools to the wider population.
While many of these claims seem promising, the practicality of AlgoStream's approach and how much it truly disrupts the field of options trading remains to be seen. It's a field ripe for innovation, and AlgoStream's work will be interesting to observe in the coming years.
7 Key YC AI Startups That Disrupted Traditional Trading Models in 2024 - RoboWealth Launches Automated Portfolio Management for Retail Investors
RoboWealth has introduced an automated portfolio management service designed to simplify investing for everyday individuals. They're aiming to make investing more accessible by offering robo-advisory services that manage and rebalance investment portfolios automatically. This is meant to broaden the appeal of sophisticated investment strategies, especially for people who haven't typically had access to this level of personalized financial management. By lowering the barriers to entry, such as minimum investment requirements, RoboWealth seeks to attract a wider user base.
This launch aligns with the growing trend of making finance more approachable for everyone, and RoboWealth seems poised to become a prominent player in this sector. The rise of these automated investment tools does bring about questions though. As these platforms become more prevalent, will there be a trade-off between the gains in efficiency and the potential for unforeseen algorithmic issues? Especially considering a growing population of investors who may not have the financial background to critically assess these systems, it's an important point to consider. It will be interesting to see how RoboWealth's service impacts traditional investment models, as it indicates a notable shift in the accessibility of sophisticated investment strategies.
RoboWealth has introduced automated portfolio management specifically designed for individual investors, making investment services potentially more accessible to a wider range of people. It employs automated portfolio management and rebalancing features, aiming to simplify investment decisions for those who may not have the time or expertise to do so themselves. RoboWealth emphasizes accessibility with low minimum investment requirements and a focus on potentially higher returns compared to traditional financial advisors. Their funding and valuations suggest it's a noteworthy company in the financial technology space.
The system, powered by algorithms, can analyze market shifts with incredible speed and tailor investment options based on a user's individual needs. It's trained on a vast dataset of past trades, hoping to leverage historical patterns to inform future investments across various asset types. A notable aspect is its incorporation of sentiment analysis, interpreting market emotions from online sources like news and social media, to add another layer of information into its decision-making process. It's fascinating that the system can adapt to an individual's changing risk tolerance. Users can set their own risk levels, and the system automatically adjusts the portfolio accordingly.
Furthermore, RoboWealth presents the possibility of significant cost reductions for investors, potentially cutting investment management fees by half, compared to conventional advisors. This could shift the landscape of how investing is done by potentially making sophisticated strategies affordable for a broader range of people. Behind the scenes, machine learning allows RoboWealth's system to constantly refine investment strategies over time, potentially achieving a more efficient investment outcome over the long run.
A key part of the system is the ability to simulate a wide variety of potential market scenarios. This capability allows users to see how their investments might react in a variety of potential future circumstances, providing a glimpse into potential risks and rewards before any real-world money is involved. The technology includes automated portfolio adjustments in reaction to market changes, a feature not available in more traditional portfolio management approaches. This "dynamic rebalancing" keeps the portfolio aligned with user goals without requiring manual intervention.
A notable safety element is the built-in compliance algorithm. This system is designed to continuously monitor the investment process and ensure the investment strategies adhere to relevant financial regulations. RoboWealth also provides predictive performance metrics for assets under specific conditions, potentially offering insights to enhance decision-making and returns for investors. Whether or not it will lead to increased returns is a question that will require more time and investigation.
While the potential benefits are intriguing, it's crucial to recognize that RoboWealth is a relatively new entrant into a highly competitive landscape. Its long-term success in delivering on its promises remains to be seen. However, the way it combines automation, machine learning, and accessibility to financial tools highlights the direction in which the financial industry is moving, emphasizing the potential of AI to change the ways investment advice and management are provided to the public.
7 Key YC AI Startups That Disrupted Traditional Trading Models in 2024 - TradePilot Uses Natural Language Processing to Track Global Market Events
TradePilot is using Natural Language Processing (NLP) to keep tabs on global market events, aiming to provide a more efficient way for trading firms to understand what's happening. This involves using NLP to analyze text data – such as news articles and social media posts – to glean insights into market sentiment and potentially identify significant events or trends impacting the markets. This kind of analysis can help traders make better decisions and possibly anticipate potential risks and opportunities more quickly.
This approach fits in with a larger trend we're seeing with some AI startups that have received funding from Y Combinator, all trying to change the way trading is done in 2024. There's a hope that by using these kinds of NLP tools, financial firms can react to big economic changes in a faster, more informed manner. But as with many AI-driven tools, questions remain about how dependable they are, especially when markets become unpredictable or major, unexpected events occur. The concern is that while they can be useful, they might not fully capture the complex insights that human traders bring to the table. It's still an open question about the extent to which these systems will replace the judgment of experienced financial professionals.
TradePilot's approach to tracking global market events is intriguing. It uses natural language processing (NLP) to sift through a massive amount of text data – think news articles, social media posts, and even economic reports – in real-time. This allows them to quickly understand what's happening in the market and how it might impact trading decisions. They're essentially trying to build a system that can 'read' the market's mood, which can be crucial for staying ahead of the curve.
One aspect that stands out is the ability to spot patterns in historical market reactions to various events. For example, they could analyze how stock prices respond to specific geopolitical situations and then use that information to guide future trading decisions. This is based on the idea that past behavior can be a useful indicator of future market behavior, but whether that's always reliable is debatable.
Furthermore, NLP enables TradePilot to analyze sentiment – whether people are generally optimistic or pessimistic about the market. This is a relatively new approach to trading. They can use this information to get a better idea of the overall market "mood," which is useful because shifts in sentiment often precede changes in price. However, correctly interpreting sentiment is difficult; nuances in language and differing motivations of market participants can lead to wrong conclusions.
What's more, the algorithms within TradePilot aren't static. They're designed to learn over time. This means as new market data comes in, they get better at making sense of the information, refining their ability to predict what might happen next. The success of this approach depends on how well the NLP models are built and how quickly they can adapt to evolving market patterns.
TradePilot seems to be built to handle large volumes of data coming from a variety of sources. This breadth and speed are necessary for options trading where speed is paramount. Their aim is to provide traders with a holistic view, combining insights gleaned from the news, social media, and other reports to make better trading decisions. But it's worth considering whether the increased complexity can overwhelm a trader or cause incorrect interpretations of what the data signifies.
Of course, there are challenges. The ability to accurately predict future behavior based on past patterns is often questioned in trading and it is unclear how well NLP truly captures market sentiment. However, TradePilot's platform is designed to be user-friendly, even for people who aren't experts in financial models or NLP. This makes it more accessible to a larger range of traders. Additionally, they've made an effort to ensure the platform complies with existing financial regulations.
Ultimately, TradePilot represents a novel way to gain insights into global market events, but whether this translates into consistently better trading decisions remains to be seen. The industry is watching closely.
More Posts from financialauditexpert.com: