eDiscovery, financial audits, and regulatory compliance - streamline your processes and boost accuracy with AI-powered financial analysis (Get started for free)
7 Key Financial Metrics Management Consultants Should Master for Effective Audits in 2025
7 Key Financial Metrics Management Consultants Should Master for Effective Audits in 2025 - AI-Driven Revenue Growth Analysis Tools
The use of AI in revenue growth analysis signifies a profound change in how businesses manage pricing and revenue. These tools, powered by sophisticated algorithms and machine learning, allow companies to adjust prices in real-time and make more informed choices. This responsiveness is crucial for navigating the increasingly competitive business environment. Companies are increasingly embracing platforms that automate many revenue-related tasks, which allows consultants to focus on more strategic work instead of manually handling data. This trend emphasizes the importance of consultants developing a solid understanding of these AI-powered tools. Gaining expertise in the AI-enhanced financial metrics that drive these tools will be essential for performing thorough and effective financial audits in the coming years, particularly as market conditions continue to shift. If consultants are not well-versed in this technology they will find it difficult to evaluate the effectiveness of their clients revenue-generating strategies.
It's fascinating how AI is transforming the landscape of revenue growth analysis. These tools can sift through mountains of data much faster than human analysts, enabling near real-time identification of revenue patterns and unusual fluctuations. Some of these tools even boast predictive capabilities, leveraging historical data and other factors to forecast future revenue with surprising accuracy, though claims of 90% are bold.
Further, the ability to segment customer data in sophisticated ways can reveal opportunities previously hidden within the noise. This opens the door to more focused marketing strategies, potentially boosting customer conversions. It's intriguing how some tools are starting to incorporate natural language processing to understand customer sentiment from diverse sources like social media and surveys. This could be a game-changer for refining sales strategies.
From a practical perspective, the impact on workflow is undeniable. AI can significantly accelerate data reconciliation, relieving analysts of tedious tasks and allowing them to focus on higher-level thinking. The iterative nature of some of these AI platforms, using reinforcement learning, promises continuous improvement in accuracy and insights over time.
Looking at the bigger picture, these tools have the potential to provide a more holistic understanding of revenue drivers by blending internal and external economic data. This could help anticipate and mitigate the effects of external economic shifts. And from an audit perspective, one of the big wins is the reduction in human error which can lead to flawed financial assessments.
The ability to model different scenarios, akin to "what-if" analyses, lets businesses explore the potential outcomes of various revenue-generating approaches. It's also interesting that the application of AI often surfaces inefficiencies in current sales processes that might have gone unnoticed otherwise. This ability to uncover and address these inefficiencies could unlock previously untapped revenue streams. While exciting, the implementation of these AI solutions requires careful consideration and understanding to ensure they produce the desired outcomes.
7 Key Financial Metrics Management Consultants Should Master for Effective Audits in 2025 - Customer Retention Metrics in the Age of Subscription Models
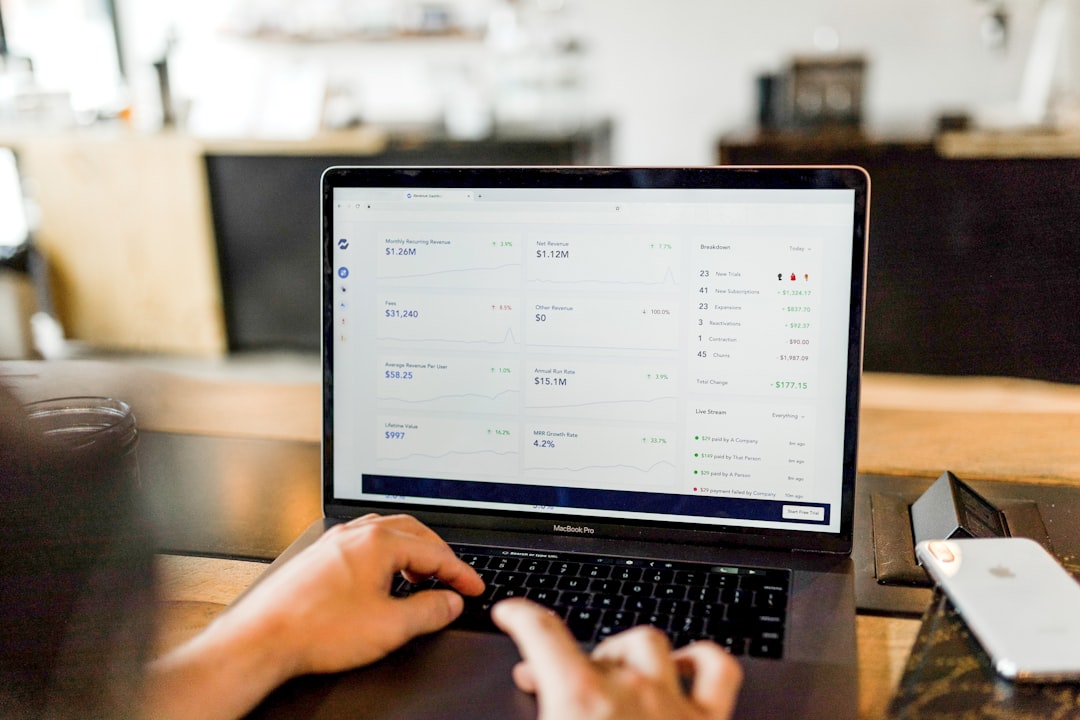
Within the context of subscription-based businesses, tracking customer retention has become critically important for long-term success. Metrics like customer retention rate and churn rate provide a window into customer satisfaction and engagement, elements vital to sustaining a subscription model. The prevalence of churn, with average monthly rates exceeding 40%, underscores the need for businesses to proactively manage customer relationships and understand why subscribers choose to leave.
Understanding how to calculate and interpret these metrics is essential. For example, the simple act of tracking customers at the beginning and end of a period, combined with new acquisitions, allows a business to calculate the retention rate.
Beyond simply retaining subscribers, however, businesses also need to consider revenue retention. This dual focus – retaining subscribers and sustaining revenue flow – is important because it highlights the intertwined nature of customer loyalty and overall financial health. This concept is particularly relevant in the world of Software as a Service (SaaS) where sustainable growth depends on a stable base of paying customers.
Given that different industries, customer bases, and pricing models can significantly affect churn, businesses need to develop retention strategies tailored to their specific circumstances. Failure to do so could lead to significant revenue losses and hinder growth. Effectively leveraging retention metrics can provide businesses with deeper insights into customer behavior, helping them develop strategies to improve loyalty and reduce churn. Ultimately, this translates to healthier bottom lines and more sustainable businesses in the competitive landscape of subscription models.
In the realm of subscription-based services, understanding how customers interact with a product or service over time is crucial. One of the core metrics is **customer retention rate**, which essentially tells us what percentage of customers stick around for a set period. This metric gives a general sense of overall satisfaction and how engaged customers are with the service.
Subscription services face a constant battle against **churn rate**. Studies indicate that the average monthly churn rate can reach around 41% with a significant portion, around 30%, being voluntary decisions, while 10% is often due to involuntary factors. This highlights the need for companies to actively try and keep customers.
Calculating retention rate isn't overly complex. You can use a straightforward formula: (Customers at the End of a Period - Newly Acquired Customers) / Customers at the Beginning of the Period * 100. This basic calculation gives a starting point to measure how many customers are sticking around.
Analyzing these metrics alongside other financial data offers companies a much richer picture. This can lead to better predictions of revenue and how to strategize growth. We see that understanding when customers typically order, as captured by metrics like ordering frequency, can influence inventory management and the entire supply chain.
It's important to consider both **revenue retention** and **subscriber retention**. While having more paying customers is generally a good thing, understanding what motivates customers to stick around gives a better overall health picture of the business.
Subscription models, particularly in the realm of Software as a Service (SaaS), are heavily dependent on retention for success. Keeping customers long-term impacts a company's viability and ability to remain competitive.
It's a constant challenge: subscription models face the reality of losing customers on a regular basis. A company's ability to create good strategies to engage and keep customers is absolutely critical. Understanding *why* customers choose to leave is a key part of improving retention rates.
Churn rates vary drastically across different businesses. Things like the industry, who the target customers are, and pricing strategies all play a role. There's no one-size-fits-all approach to retention, so targeted strategies are key.
Successful customer retention efforts can lead to more profit. By understanding what causes customers to cancel a subscription, companies can refine their strategies to improve customer loyalty. It's a continuous process of learning and adapting to customer behavior.
7 Key Financial Metrics Management Consultants Should Master for Effective Audits in 2025 - Operational Efficiency Benchmarks for Remote Auditing
Remote auditing, propelled by the shift to digital work environments, has brought into sharp focus the need for operational efficiency benchmarks. The cost landscape of auditing varies widely, with factors like firm size and audit type impacting expenses. This highlights the necessity for auditors to refine their processes and manage costs effectively.
The transition to remote audits, spurred by the pandemic, has underscored the importance of tracking performance. Key Performance Indicators (KPIs) have become even more vital in ensuring audits maintain quality while teams continue to perform effectively. This adaptation calls for a recalibration of audit priorities and the strategic adoption of technologies that support remote collaboration. These tools, ranging from document sharing platforms to video conferencing, can play a major role in the overall success of remote audits.
Furthermore, the rising complexity of modern businesses increases the stakes for operational efficiency. Auditors need to establish robust benchmarks to navigate the challenges of remote audits in this changing landscape. The goal is to not just maintain, but improve efficiency, particularly as the use of artificial intelligence in revenue analysis reshapes the audit environment. While some efficiencies are gained, over-reliance on technology can also create new areas where risk needs to be managed. Finding the right balance is key.
It's interesting to see how remote auditing has shifted the landscape of financial audits, especially considering the changes brought about by the pandemic. While some initial concerns were raised about the shift to remote work, it's becoming clearer that remote auditing can actually improve efficiency and perhaps even enhance the quality of audits.
Some studies suggest that remote auditing can cut costs by 10 to 20% for firms, mainly because of the reduction in travel and other expenses. Interestingly, these same studies also point to a potential reduction in audit time by as much as 50%. It's curious how auditors can access the necessary documents quickly through digital means.
Further, I've seen reports that indicate remote collaboration platforms can accelerate communication, with some researchers suggesting up to a 40% decrease in delays. This quicker feedback loop seems to translate into faster decision-making during the audit process. This seems promising as it allows auditors to be more agile in making changes during the audit. However, it's worth noting that studies have shown that during the pandemic, audits experienced significant delays due to the pressure on the system.
The move towards digital tools also seems to be improving error detection. For example, using dynamic data visualizations in digital platforms can potentially increase error detection rates by 25%. While it's not always clear how this will play out in practice, it's a promising area worth exploring further. This is intriguing because traditional audit practices heavily relied on spreadsheets, which are relatively static.
Additionally, some of the tools used in remote auditing allow multiple stakeholders to access and review files simultaneously, potentially speeding up review cycles and resulting in a 15% reduction in overall audit time. It's also notable that cloud-based data storage is improving access to audits and related documents, increasing the overall flexibility of organizations in managing these records.
Another aspect to consider is the impact on the auditors themselves. It seems that the flexibility of remote work can lead to higher job satisfaction and possibly reduced burnout. There's a strong indication that it's allowing auditors to operate in environments that suit them best.
Artificial intelligence is also starting to find its place in the remote audit environment, with researchers showing that it can detect anomalies in financial data with a high degree of accuracy – around 85% in some cases. While it's still a nascent field, it highlights the potential to shift away from traditional manual processes and potentially further enhance audit accuracy.
However, we can't overlook the critical importance of data security in a remote environment. It's encouraging to see that cybersecurity protocols designed for remote audits are becoming more robust, with improvements in data protection leading to a roughly 40% increase in the effectiveness of preventing unauthorized access to audit records. It's vital to make sure the confidentiality and integrity of data are fully protected during remote audit processes.
Ultimately, remote auditing appears to be a fascinating area of innovation, offering the potential to improve efficiency, enhance quality, and, if managed correctly, maintain security. However, as with any technological change, continued careful monitoring and evaluation will be crucial as the field evolves and matures.
7 Key Financial Metrics Management Consultants Should Master for Effective Audits in 2025 - ESG Compliance Metrics in Financial Reporting
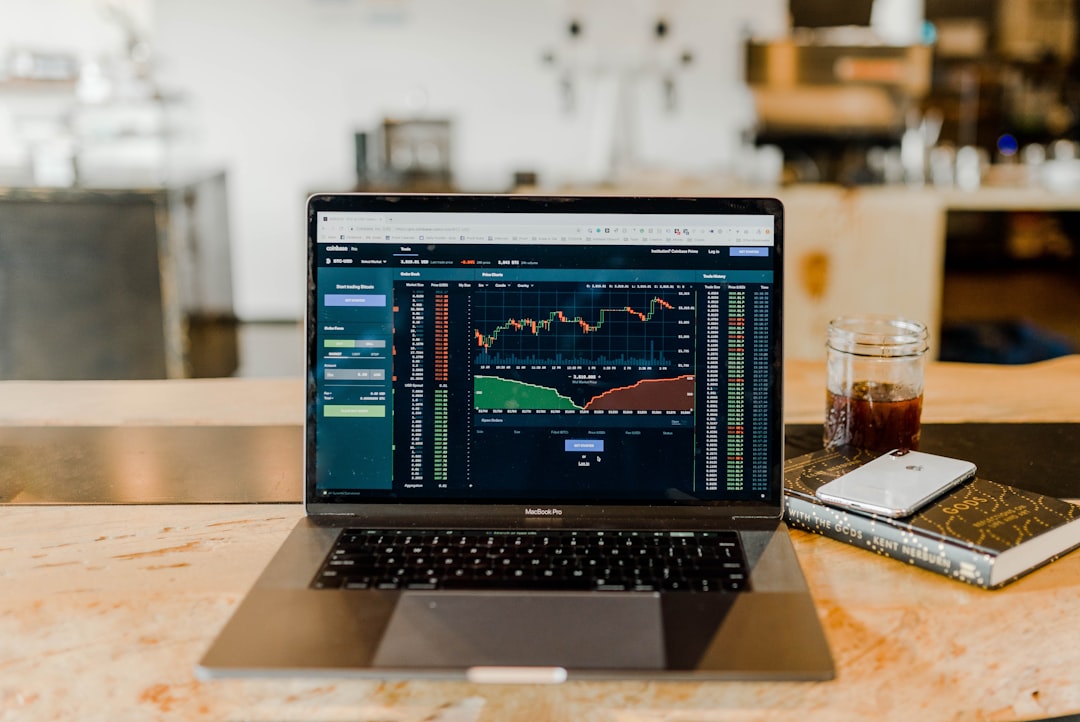
ESG considerations are increasingly woven into financial reporting, prompting a need for businesses to develop a consistent system for identifying and measuring relevant ESG metrics. This shift is driven by a heightened focus from investors, including private equity players, who are demanding more transparency into how companies manage environmental, social, and governance issues. Key ESG metrics, like greenhouse gas emissions and diversity data, are now commonly reported and seen as critical markers of a company's commitment to sustainability.
However, the challenge remains in effectively incorporating ESG considerations into the overall corporate strategy. Often, this leads to a disconnect between ESG efforts and the company's narrative, making it difficult for investors to grasp the financial impact of these initiatives. Additionally, a lack of standardization in ESG reporting continues to be an obstacle. There's a growing call for more consistent and reliable ESG disclosures, leading to the development of various frameworks aimed at achieving this goal. This need for consistent measurement and reporting is particularly important in the current environment, and suggests that we are in a period of evolution as the expectations around ESG reporting continue to change.
Creating a consistent way to pinpoint important ESG factors is key for accurate measurement and reporting. This helps investors and company leaders make better choices. It's interesting how private equity groups, from the big players (GPs) to smaller ones (LPs) and the companies they invest in, are paying more attention to ESG. Some of the commonly tracked ESG metrics include things like how much greenhouse gas a company produces, diversity in the workplace, and the amount of tax paid. These give a sense of how well a company is doing in terms of sustainability.
ESG reporting is crucial for investment funds to understand and control the risks tied to environmental, social, and governance issues. These risks can influence a company's financial health. A lot of companies are working with outside experts to help with ESG reporting. These outside companies collect and examine data related to ESG factors. It's become clear that combining ESG and traditional financial data into one reporting system is essential for managing performance in a way that considers long-term sustainability.
Advanced tech, like artificial intelligence, is increasingly used to process and interpret complex ESG data. Although a majority of the biggest companies (over 95% of the S&P 500) put out sustainability reports, it's surprising that only a small number successfully connect their ESG efforts to their overall financial picture in a clear way. It's a concern that many companies haven't successfully linked their ESG plans to their overall business strategy. This makes it tough for investors to understand how these initiatives might impact financial outcomes.
It's a challenge to make sure that ESG reports are consistent and high quality. This has pushed the need for standard reporting frameworks and measurement methods. While there's a lot of talk about ESG, the lack of a uniform approach to tracking and reporting can be confusing for investors who are trying to compare companies. It's something worth exploring as it could improve transparency and create more reliable benchmarks across industries. It's also interesting to see how new rules and regulations about ESG are affecting the financial reporting landscape. It's not always easy for companies to keep up with the evolving set of guidelines and expectations around ESG.
7 Key Financial Metrics Management Consultants Should Master for Effective Audits in 2025 - Blockchain-Based Customer Lifetime Value Calculation
Blockchain technology offers a potentially more accurate and transparent way to calculate Customer Lifetime Value (CLV). By storing and analyzing detailed transaction data in a secure and tamper-proof manner, blockchain can provide a much more comprehensive picture of individual customer behavior. This allows businesses to build a more nuanced understanding of how customers interact with their offerings over time, ultimately leading to more precise CLV assessments.
This approach supports the idea behind the Resource-Based View (RBV), which emphasizes leveraging unique organizational strengths to gain a competitive edge. Blockchain, by providing a detailed view of customer interactions, can help businesses tailor strategies to maximize engagement and customer retention, reinforcing the RBV approach.
While blockchain holds immense potential in calculating CLV, companies need to be aware of the complexities of integrating and managing blockchain-based data within their existing systems. As blockchain technology matures, its role in guiding financial audits is likely to grow, potentially impacting how consultants approach audits in 2025. It's an area to watch, but at this point, it's still early days and the complexities of implementation are not to be underestimated.
Customer Lifetime Value (CLV) is a key metric that estimates the total revenue a business can expect from a single customer over their entire relationship with the company. Typically, it's calculated using a simple formula: the number of purchases multiplied by the value of each purchase and then multiplied by the average lifespan of a customer. But whether to focus on revenue or profit when determining the 'value of purchase' is a critical decision that impacts the overall meaning of the calculation.
There's a whole range of different formulas and models used for calculating CLV, each highlighting the importance of certain factors that contribute to a customer's overall value. From basic calculations to sophisticated models that require a ton of transaction data, it can get complex quickly.
The idea of strategically managing CLV is important for achieving short-term wins and long-term financial success. The Resource-Based View (RBV) theory emphasizes that firms can use their unique resources and capabilities to maximize CLV and gain a competitive edge. Having the ability to process a massive amount of transaction data is vital for businesses to create detailed customer-specific metrics.
Understanding CLV helps businesses optimize marketing strategies, which leads to improved profitability and better decision-making. Effective CLV metrics can reveal important insights that impact the overall business strategy and financial results, encouraging businesses to both keep existing customers and acquire new ones.
Blockchain, with its decentralized and immutable nature, offers the potential to enhance CLV calculations. By storing customer interactions and transactions on a blockchain, businesses can potentially minimize data security risks and strengthen data integrity compared to traditional centralized databases. Since blockchain transactions are transparent, it could lead to greater trust between businesses and customers, especially as it relates to how their behavior translates into their overall lifetime value. The immutable nature of the data can ensure the accuracy of CLV calculations over time.
Using blockchain's real-time capability to track customer behaviors can help businesses quickly adapt their marketing efforts to boost retention. This could prove useful as customers increasingly demand personalized and responsive experiences. Smart contracts could automate the calculation process, potentially leading to increased efficiency and a reduction in human errors that can distort calculations.
One of the challenges is integrating blockchain data with other sources like social media and e-commerce platforms. Doing this could create a holistic view of customer engagement and provide a deeper understanding of various customer segments. While intriguing, implementing loyalty programs based on blockchain-based tokens presents its own set of challenges and needs careful consideration. The potential for reducing fraud through the use of blockchain could be beneficial, as fraud can significantly skew CLV assessments.
However, concerns about the accessibility and control of data on blockchain still linger. The potential for democratizing data, while potentially fostering a more equitable business environment, needs careful thought as it relates to privacy. Overall, blockchain has the potential to revolutionize CLV calculations, but it's still a developing field with complexities that need to be addressed.
7 Key Financial Metrics Management Consultants Should Master for Effective Audits in 2025 - Churn Rate Prediction Using Machine Learning Algorithms
Predicting customer churn has become increasingly important for businesses, especially those with subscription models, as a way to improve customer retention and bolster revenue. Machine learning has emerged as a powerful tool for this purpose, using predictive analytics to pinpoint customers likely to cancel and help uncover the reasons why. By understanding the factors that lead to churn, businesses can better target retention efforts. Various machine learning algorithms are being tested to see which provides the most accurate predictions across different industries, such as e-commerce and the banking sector. The need for this kind of analysis is particularly evident in industries reliant on consistent customer subscriptions, like software-as-a-service, where losing customers can have a major impact on revenue stability. Because customer behavior is complex, consultants advising clients need to understand the potential of these AI-powered predictive tools to ensure they are effectively guiding their clients in today's competitive environment. While some progress is being made, fully integrating these advanced prediction capabilities into operations is still a challenge.
Forecasting customer churn using machine learning has become a popular area of research, but it's interesting that sometimes traditional statistical approaches, like logistic regression, can be equally effective, particularly when working with smaller or less complex datasets. This suggests that a balanced approach, combining both modern and established techniques, might be the most insightful path forward.
While machine learning algorithms can boost prediction accuracy, it's notable that the human element, particularly understanding customer sentiment through feedback and surveys, remains critical for grasping the root causes of churn. It seems that algorithms, on their own, can struggle to capture the nuances of human emotions and motivations, highlighting the need for a more comprehensive approach.
Developing churn prediction models requires a lot of data, but it's important to realize that the quality of data varies significantly. Customer behavior data, for instance, can be very uneven and sometimes has a bias towards certain behaviors. This can present issues when training models as it can significantly impact prediction accuracy and ultimately skew the effectiveness of the models.
The effectiveness of churn prediction models hinges on the careful selection and engineering of features. Surprisingly, factors related to customer engagement, like the number of service requests or how often they interact with a product, can be better predictors than factors like their purchase history. This emphasizes the significance of identifying the most impactful features when designing a model.
Customer churn is a dynamic process, and the models used to predict it need to keep up. Studies show that models regularly updated with fresh data, such as weekly or monthly, tend to capture changes in customer behavior more accurately. This highlights the need for agility in churn management strategies, adapting to the evolving patterns of customer behavior.
Churn rates aren't consistent across industries. SaaS companies, for instance, may experience rates of over 30%, while e-commerce companies, likely because they rely on transactions instead of subscriptions, tend to have lower rates—around 10-20%. This showcases how approaches to churn management need to be tailored to different industries and business models.
Companies that invest in churn prediction tools may be surprised by the cost savings they can achieve. Reducing churn, even by a small percentage, can significantly impact revenue and often provides a greater return than the cost of acquiring new customers. This highlights the potential of leveraging churn prediction to improve overall business performance.
Most churn models are designed to predict who will churn. However, there's a newer and more strategic approach called prescriptive analytics. These models don't just predict churn; they suggest actions to proactively improve customer retention, giving companies a significant advantage in managing churn.
It's interesting how often companies undervalue the impact of even small improvements in their churn prediction models. A seemingly minor 5% boost in prediction accuracy can result in substantial financial benefits, emphasizing the value of continuous model refinement and optimization.
As companies adopt more sophisticated machine learning techniques, it's important to consider the ethical implications related to customer data privacy and transparency. Consumers might be concerned about how their data is being used for predictive analysis. This underscores the need for companies to reconsider their approach to data governance and cultivate a more transparent relationship with their customers.
eDiscovery, financial audits, and regulatory compliance - streamline your processes and boost accuracy with AI-powered financial analysis (Get started for free)
More Posts from financialauditexpert.com: